Local weight coupled network: multi-modal unequal semi-supervised domain adaptation
MULTIMEDIA TOOLS AND APPLICATIONS(2024)
摘要
Existing semi-supervised domain adaptation (SSDA) approaches on visual classification usually assume that the labelled source data are only collected from single modality. However, since single source data cannot fully show the characteristics of the target data, source domain may be collected from multiple modalities (i.e. RGB and depth modalities). Traditional domain adaptation (DA) task makes an unrealistic scenario, where the label space in the source equals to the label space in the target. However, in real-world scenario, source and target domains may have different label spaces. Thus, the irrelevant categories in the source domain will cause two challenges: negative transfer and imbalanced distribution. In this paper, we design a novel deep SSDA framework in an end-to-end fashion, termed Local Weight Coupled Network (LWCN) for effective knowledge transfer, which aims to take advantage of the multi-modal information in the source domain and tackle the mentioned challenges, simultaneously. Specially, we construct the output layer including classification and regression, where the multi-class classifier and the multi-layer feature extractor can be learned jointly for mutual benefits. Empirical evaluations on five cross-domain benchmarks illustrate the competitive performance of our model with respect to the state-of-the-art, especially under the unequal categories scenario.
更多查看译文
关键词
Multi-modal data,Semi-supervised domain adaptation,Unequal categories,Image classification
AI 理解论文
溯源树
样例
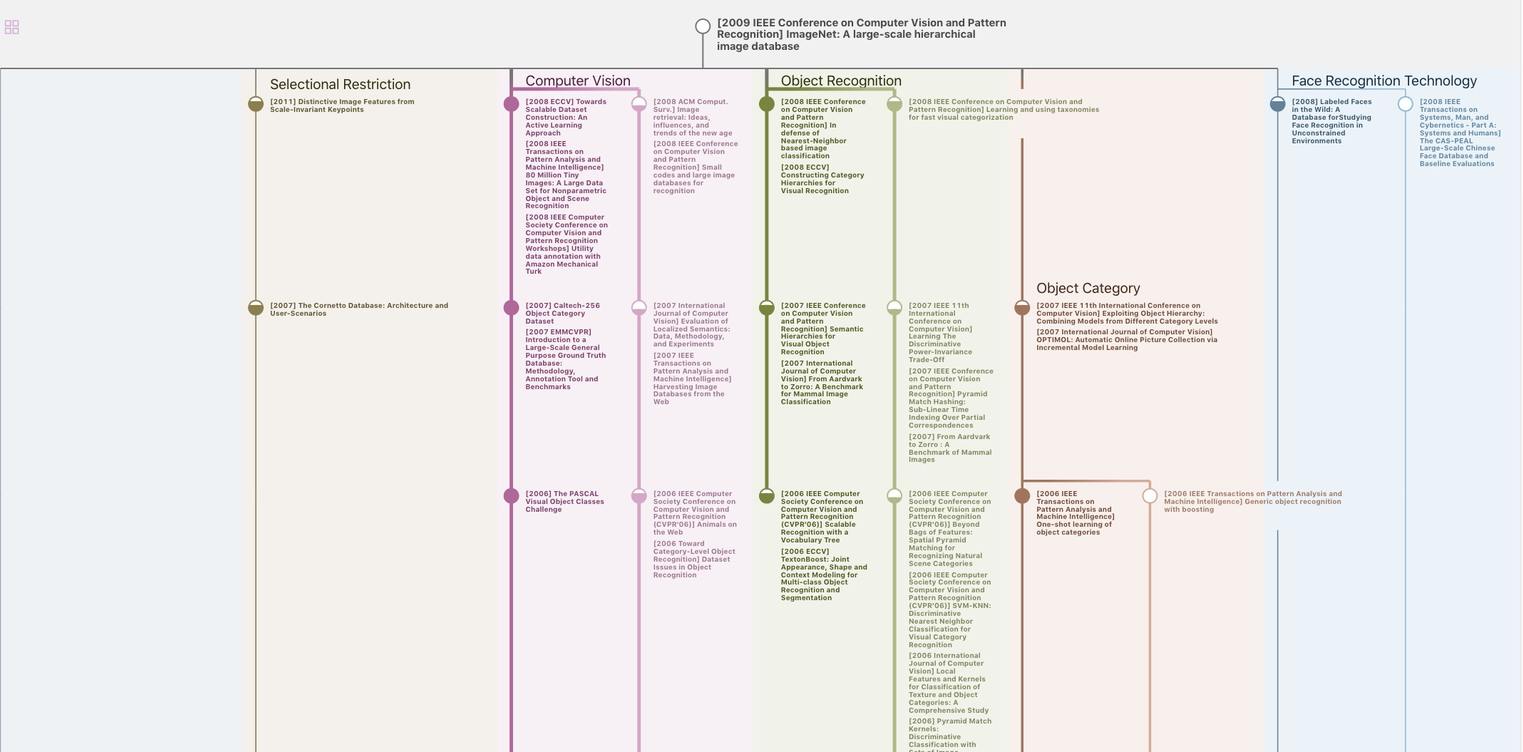
生成溯源树,研究论文发展脉络
Chat Paper
正在生成论文摘要