Machine learning classification of Austin Chalk chemofacies from high-resolution x-ray fluorescence core characterization
AAPG BULLETIN(2023)
摘要
The Upper Cretaceous Austin Chalk (AC) Group is an unconven-tional reservoir that extends across Texas and Louisiana. It is composed of interbedded layers of marly chalks to calcareous-siliciclastic mudrocks that vary in the degree of lamination, bioturbation, mineral abundance, and organic matter richness. Inte-grating lithologic observations with geochemistry is critical for interpreting depositional environments and modeling reservoir properties. Central to this integration is the ability to characterize the geochemistry of core samples at a resolution that captures thin-layered heterogeneity common to mudrock systems. Here, we developed a training data set using a semisupervised chemofa-cies clustering approach that is explored with a deep neural net-work model to predict chemofacies across multiple cores of the AC Group. Eight chemofacies are identified that capture differences in inorganic geochemistry, mineral abundance, rock fabric, and or-ganic matter richness; three classify differences in the marly chalks, four classify differences in the calcareous-siliciclastic mudrocks, and one is transitional between marly chalk and calcareous-siliciclastic mudrocks. Two distinct siliciclastic-carbonate mixing trends are identified that differ in modal abundances of tectosilicates and total clay. Two chemofacies are distinguished based on differences in Mo and V trace element enrichment, suggesting differences in bottom-water redox chemistry. Collectively, this approach pro-vides a means to integrate geochemical measurements and litholog-ical observations to interpret the depositional environments of mudrock systems and is an important step toward upscaling core data to characterize reservoir quality.
更多查看译文
AI 理解论文
溯源树
样例
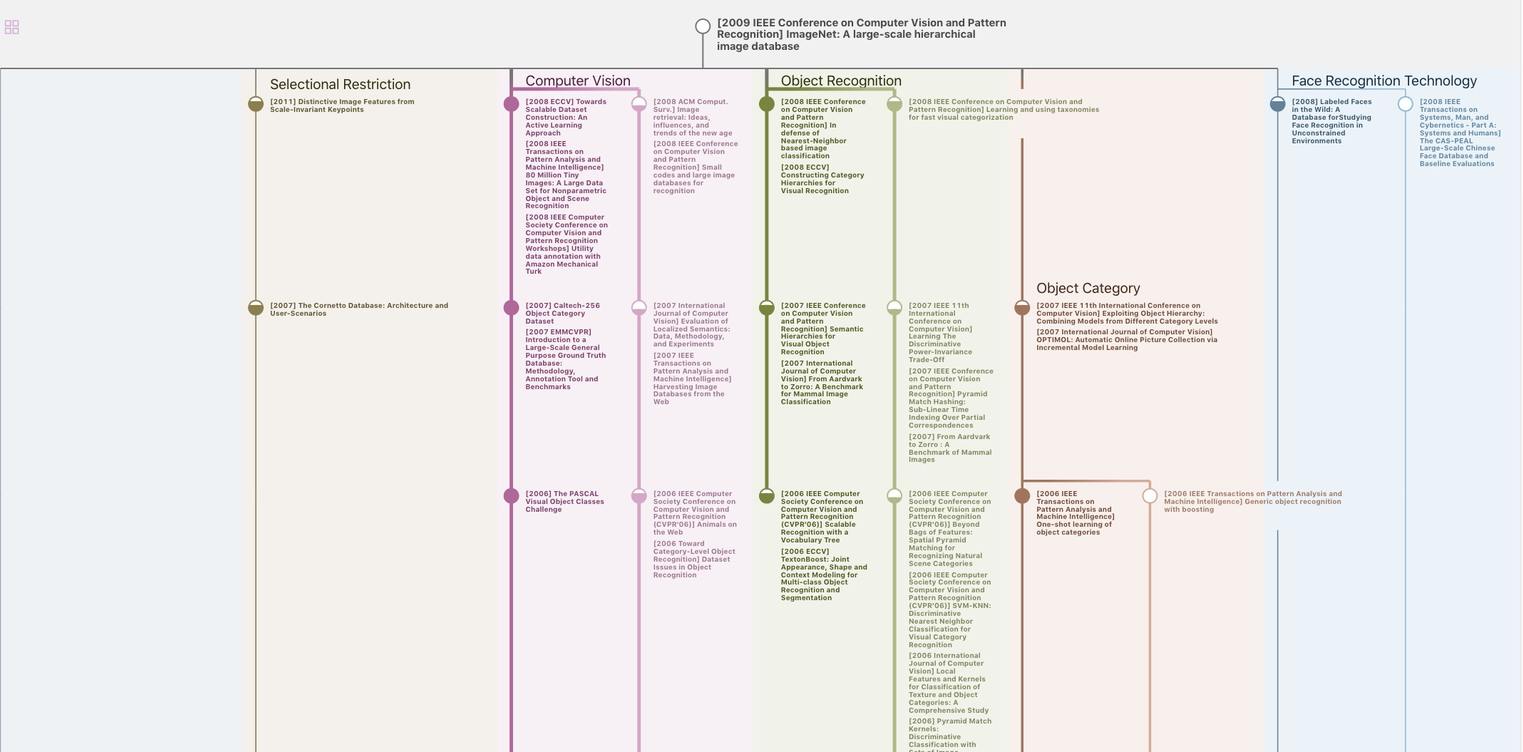
生成溯源树,研究论文发展脉络
Chat Paper
正在生成论文摘要