Study of coffee sensory attributes by ordered predictors selection applied to 1H NMR spectroscopy
MICROCHEMICAL JOURNAL(2023)
摘要
The determination of sensory attributes of coffee samples through trained cupping tasters is one of the main methodologies for coffee quality control. However, this analysis may suffer some distortions due to different ways of interpreting quality from the tasters' perspective. Thus, other, and complementary have been developed as alternative ways to determine sensory attributes. Hydrogen nuclear magnetic resonance (1H NMR) is a technique that allows the chemical study of compounds present in organic matrices. Through 1H NMR it is possible to predict a sensory attribute using chemometric methods. However, 1H NMR generates many variables per sample, making it necessary to use variable selection methods to find the most useful information. Ordered predictors selection (OPS) is a variable selection method developed to search, sort, and select the variables in order of importance. In this study, six sensory attributes from 42 coffee samples were predicted by partial least squares (PLS) and support vector regression (SVR) methods and OPS as variable selection in 1H NMR data set. The integration of areas of the 1H NMR spectra and the full data sets were also used to compare the prediction models. OPS-PLS model was the best strategy to predict aftertaste, balance, and fragrance with root mean squared error of prediction (RMSEP) of 0.24, 0.35, and 0.20, respectively. The coefficients of determination for prediction (R2p) of these models were 0.71, 0.60, and 0.77, respectively. OPS-SVR predominated as the best strategy to predict acidity, body, and flavor with RMSEP of 0.23, 0.21, and 0.22, and R2p of 0.89, 0.75, and 0.83, respectively. None of the models chosen as the best showed the presence of trends in residuals above 5% of significance. Variable selection by OPS can improve the prediction of regression models.
更多查看译文
关键词
Special coffee,Sensory attributes,1H NMR,OPS,Regression models
AI 理解论文
溯源树
样例
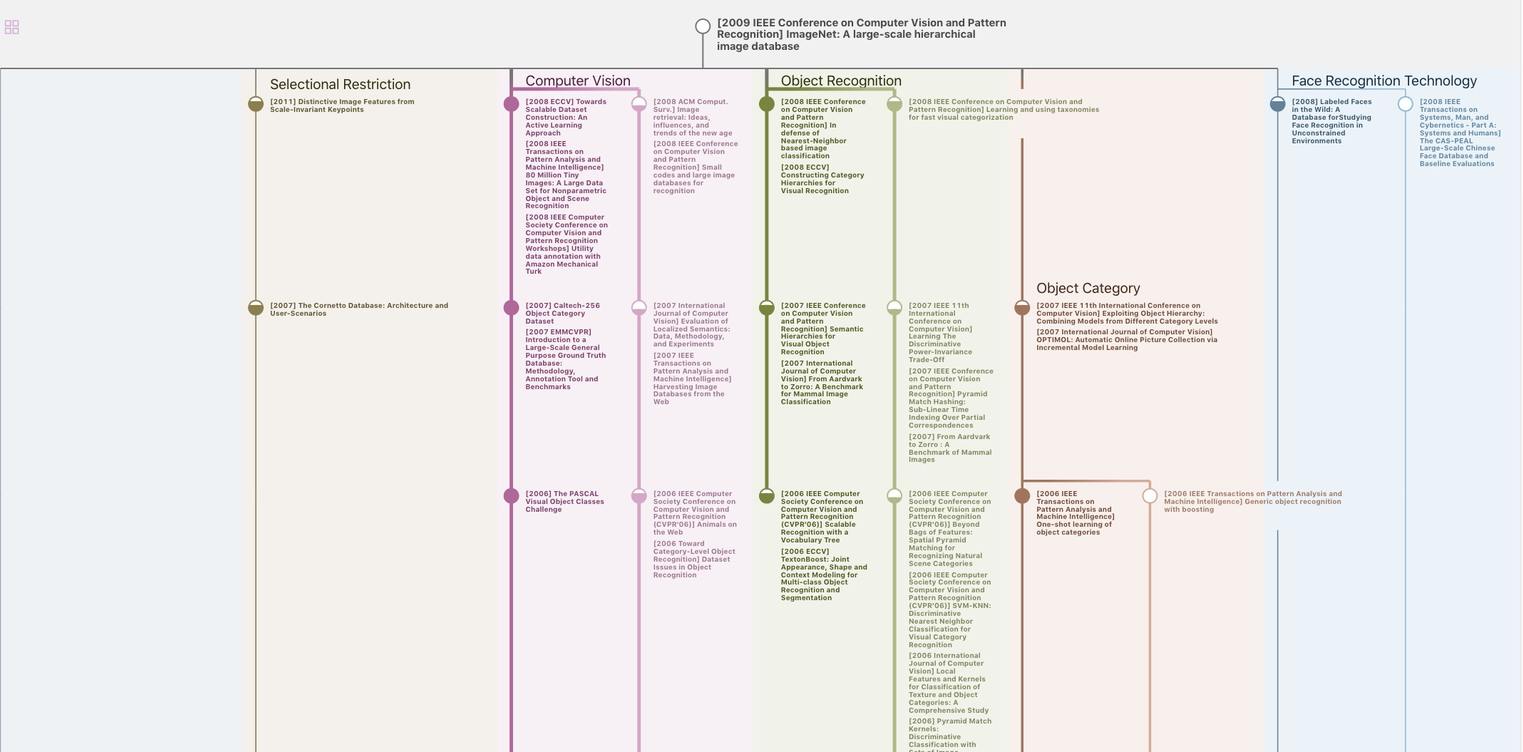
生成溯源树,研究论文发展脉络
Chat Paper
正在生成论文摘要