EFSCNN: Encoded Feature Sphere Convolution Neural Network for fast non-rigid 3D models classification and retrieval
Computer Vision and Image Understanding(2023)
摘要
Traditional methods of classification and retrieval for non-rigid 3D models, such as topological structure-based methods and spectral analysis-based methods, high rely on manual interventions, which leads to the fail of generalization. Recently, methods based on deep learning have been proposed for rigid 3D models, but they are usually not suitable for non-rigid 3D models. Due to excessive redundant calculations, point clouds-based methods and multi-view-based methods are also inefficient. In this paper, a novel deep learning framework for non-rigid 3D model classifications is presented. Different from other point clouds-based methods and multi-view-based method, this framework utilize a 3D sphere view and a new convolution way. First, an encoded feature sphere (EFS) is constructed to express the non-rigid 3D model through feature encoding, spherical projection, and crucial points extraction. Then, loop convolution and longitudinal–latitudinal loop convolution are combined into EFSCNN to extract the discriminative feature descriptor of the non-rigid 3D model. EFSCNN is a hierarchical network based on multi-direction convolution, which expands the receptive field and enhances the ability to capture local features. With the single view input EFS, EFSCNN achieves competitive performance on major non-rigid model classification and retrieval benchmarks with significantly faster runtime than previous studies.
更多查看译文
关键词
Non-rigid 3d models,Classification and retrieval,Encoded feature sphere,Multi-direction convolution
AI 理解论文
溯源树
样例
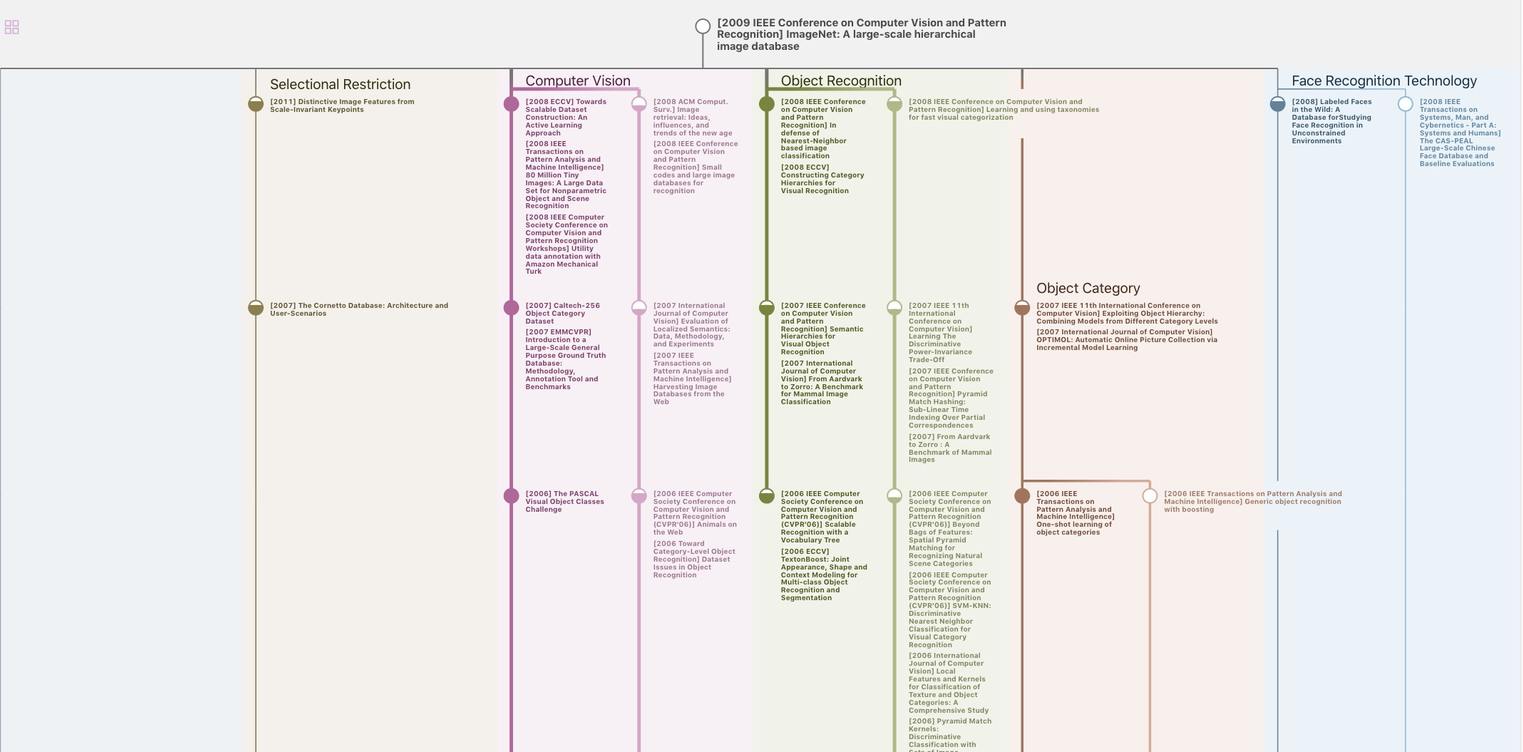
生成溯源树,研究论文发展脉络
Chat Paper
正在生成论文摘要