Automatic modulation recognition using CNN deep learning models
MULTIMEDIA TOOLS AND APPLICATIONS(2024)
摘要
Modulation techniques are widely required in communication applications, especially in wireless communication systems. Thus, it is necessary to classify and recognize types of modulation. Deep learning (DL) models can help precisely recognize the modulation techniques, provided that well-designed and trained DL models for high-performance recognition are developed. In this paper, two different convolutional neural network (CNN) models are proposed for recognition of modulation techniques. These models are applied to two different datasets: the first dataset consists of mathematical vectors, namely RadioML2016.10a and the second dataset contains 24,460 images. The RadioML2016.10a has eleven classes of modulation techniques, namely 8PSK, AM-DSB, AM-SSB, BPSK, CPFSK, GFSK, PAM4, QAM16, QAM64, QPSK, and WBFM, while the images dataset has eight classes of digital modulation techniques, namely 16PSK, 16QAM, 2PSK, 32QAM, 64QAM, 8PSK, 8QAM, and QPSK. The two CNN models are trained and the models' parameters are precisely tuned of for high-accuracy achievement. Experimentally, classification reports of modulation techniques and receiver operating characteristic (ROC) curves are used to analyze the performance of the proposed models. The CNN models provide high performance, with a testing accuracy of 53.65% and 94.39%. The results show that the area under the micro-average ROC curves for the CNNs is 92% and 97%.
更多查看译文
关键词
Modulation techniques,Deep learning,Modulation recognition,Convolutional neural network (CNN)
AI 理解论文
溯源树
样例
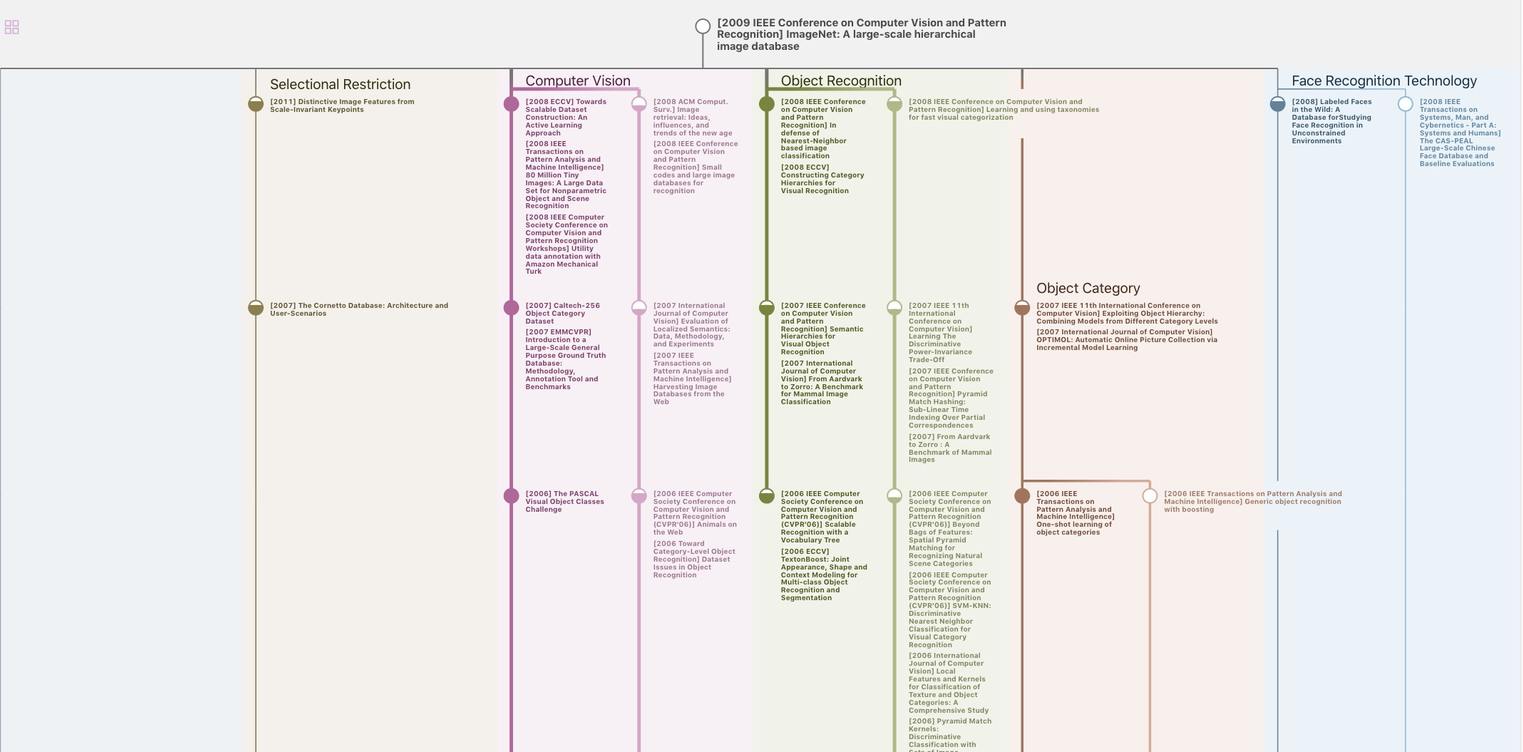
生成溯源树,研究论文发展脉络
Chat Paper
正在生成论文摘要