Anomalous Behavior Detection with Spatiotemporal Interaction and Autoencoder Enhancement
ELECTRONICS(2023)
摘要
To reduce the cargo loss rate caused by abnormal consumption behavior in smart retail cabinets, two problems need to be solved. The first is that the diversity of consumers leads to a diversity of actions contained in the same behavior, which makes the accuracy of consumer behavior identification low. Second, the difference between normal interaction behavior and abnormal interaction behavior is small, and anomalous features are difficult to define. Therefore, we propose an anomalous behavior detection algorithm with human-object interaction graph convolution and confidence-guided difference enhancement. Aiming to solve the problem of low accuracy of consumer behavior recognition, including interactive behavior, the human-object interaction graph convolutional network is used to recognize action and extract video frames of abnormal human behavior. To define anomalies, we detect anomalies by delineating anomalous areas of the anomaly video frames. We use a confidence-guided anomaly enhancement module to perform confidence detection on the encoder-extracted coded features using a confidence full connection layer. The experimental results showed that the action recognition algorithm had good generalization ability and accuracy, and the screened video frames have obvious destruction characteristics, and the area under the receiver operating characteristic (AUROC) curve reached 82.8% in the detection of abnormal areas. Our research provides a new solution for the detection of abnormal behavior that destroys commodity packaging, which has considerable application value.
更多查看译文
关键词
intelligent retail,anomaly detection,graph convolutional networks,action recognition,semantic segmentation
AI 理解论文
溯源树
样例
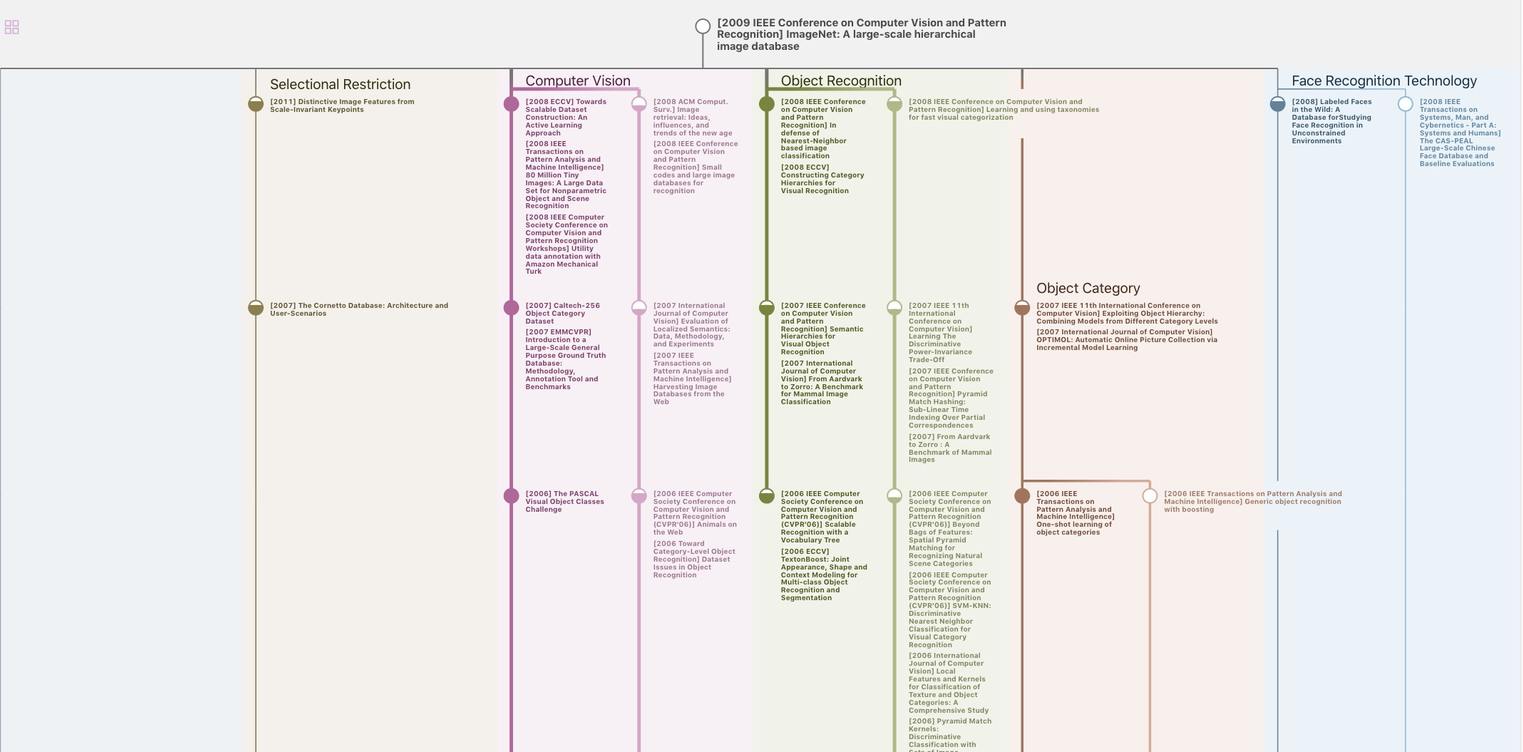
生成溯源树,研究论文发展脉络
Chat Paper
正在生成论文摘要