Joint self-supervised learning and adversarial adaptation for monocular depth estimation from thermal image
Mach. Vis. Appl.(2023)
摘要
Depth estimation from thermal images is one potential solution to achieve reliability and robustness against diverse weather, lighting, and environmental conditions. Also, a self-supervised training method further boosts its scalability to various scenarios, which are usually impossible to collect ground-truth labels, such as GPS-denied and LiDAR-denied conditions. However, self-supervision from thermal images is usually insufficient to train networks due to the thermal image properties, such as low-contrast and textureless properties. Introducing additional self-supervision sources (e.g., RGB images) also introduces further hardware and software constraints, such as complicated multi-sensor calibration and synchronized data acquisition. Therefore, this manuscript proposes a novel training framework combining self-supervised learning and adversarial feature adaptation to leverage additional modality information without such constraints. The framework aims to train a network that estimates a monocular depth map from a thermal image in a self-supervised manner. In the training stage, the framework utilizes two self-supervisions; image reconstruction of unpaired RGB-thermal images and adversarial feature adaptation between unpaired RGB-thermal features. Based on the proposed method, the trained network achieves state-of-the-art quantitative results and edge-preserved depth estimation results compared to previous methods. Our source code is available at www.github.com/ukcheolshin/SelfDepth4Thermal
更多查看译文
关键词
Depth estimation,Self-supervised learning,Adversarial domain adaptation,Thermal image,Thermal vision
AI 理解论文
溯源树
样例
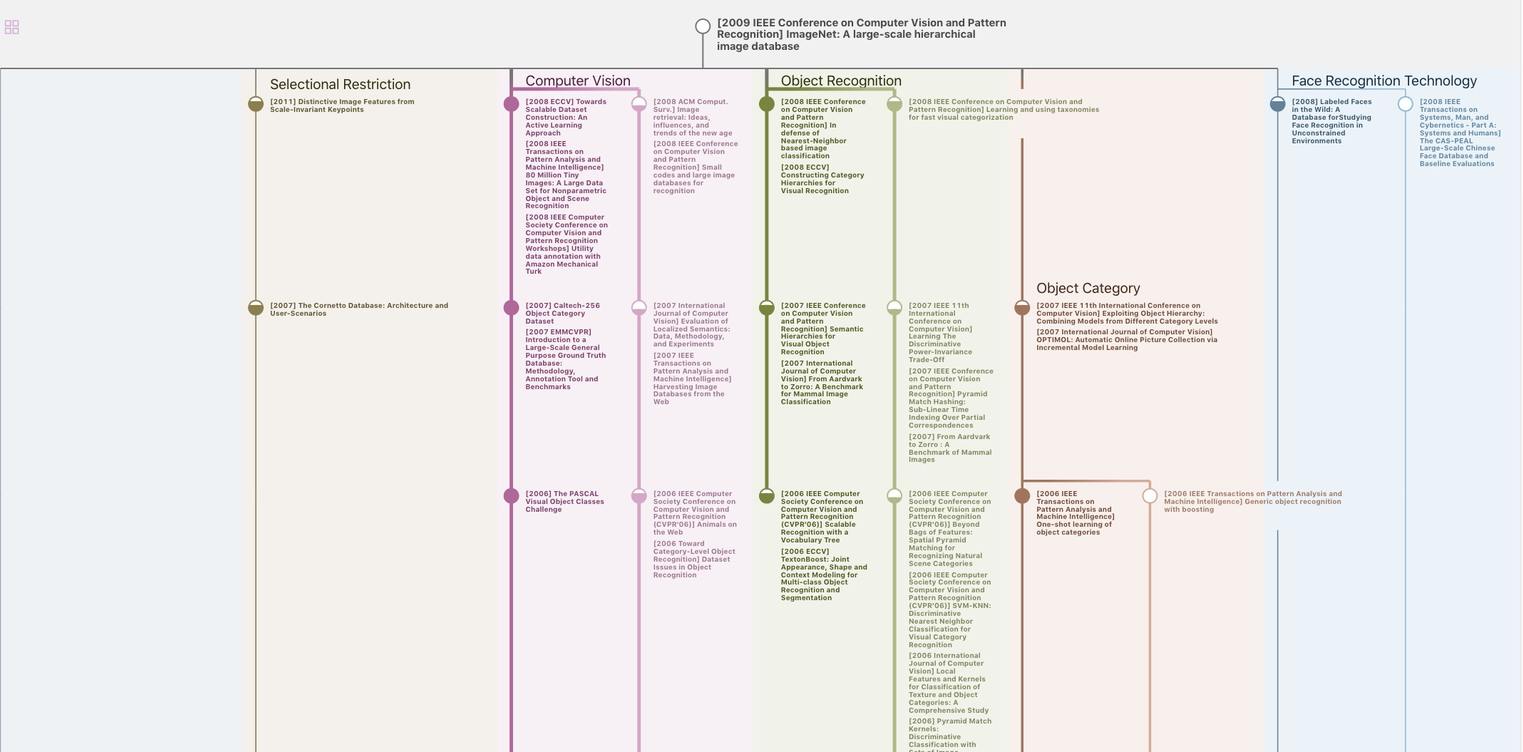
生成溯源树,研究论文发展脉络
Chat Paper
正在生成论文摘要