Bayesian Analysis Methods for Two-Level Diagnosis Classification Models
JOURNAL OF EDUCATIONAL AND BEHAVIORAL STATISTICS(2023)
摘要
Understanding whether or not different types of students master various attributes can aid future learning remediation. In this study, two-level diagnostic classification models (DCMs) were developed to represent the probabilistic relationship between external latent classes and attribute mastery patterns. Furthermore, variational Bayesian (VB) inference and Gibbs sampling Markov chain Monte Carlo methods were developed for parameter estimation of the two-level DCMs. The results of a parameter recovery simulation study show that both techniques appropriately recovered the true parameters; Gibbs sampling in particular was slightly more accurate than VB, whereas VB performed estimation much faster than Gibbs sampling. The two-level DCMs with the proposed Bayesian estimation methods were further applied to fourth-grade data obtained from the Trends in International Mathematics and Science Study 2007 and indicated that mathematical activities in the classroom could be organized into four latent classes, with each latent class connected to different attribute mastery patterns. This information can be employed in educational intervention to focus on specific latent classes and elucidate attribute patterns.
更多查看译文
关键词
diagnostic classification models, latent class analysis, variational Bayesian inference, Gibbs sampling algorithm
AI 理解论文
溯源树
样例
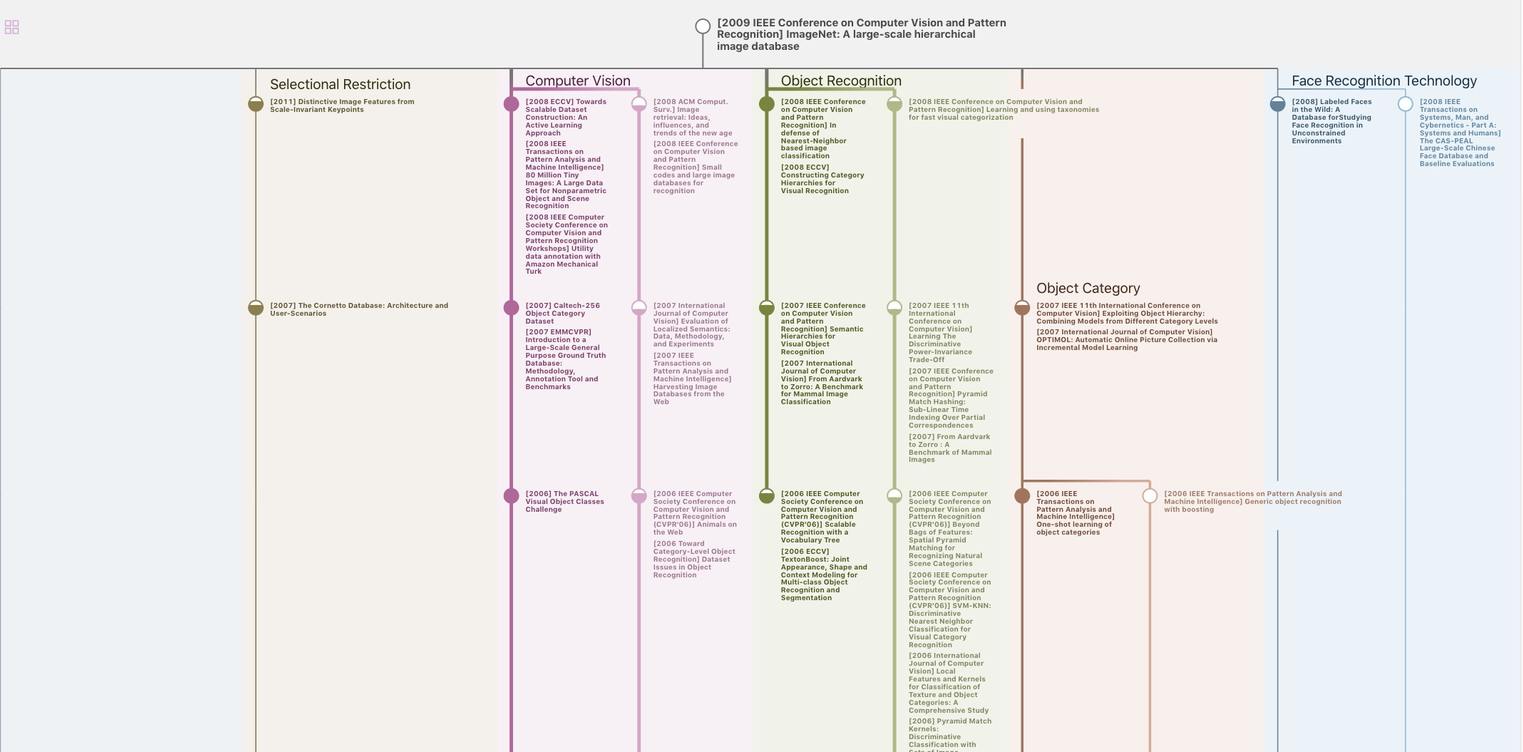
生成溯源树,研究论文发展脉络
Chat Paper
正在生成论文摘要