Bayesian Exploratory Factor Analysis via Gibbs Sampling
JOURNAL OF EDUCATIONAL AND BEHAVIORAL STATISTICS(2024)
摘要
Bayesian methods to infer model dimensionality in factor analysis generally assume a lower triangular structure for the factor loadings matrix. Consequently, the ordering of the outcomes influences the results. Therefore, we propose a method to infer model dimensionality without imposing any prior restriction on the loadings matrix. Our approach considers a relatively large number of factors and includes auxiliary multiplicative parameters, which may render null the unnecessary columns in the loadings matrix. The underlying dimensionality is then inferred based on the number of nonnull columns in the factor loadings matrix, and the model parameters are estimated with a postprocessing scheme. The advantages of the method in selecting the correct dimensionality are illustrated via simulations and using real data sets.
更多查看译文
关键词
Gibbs sampling,model dimensionality,ordering dependence,sparsity,spike-slab prior
AI 理解论文
溯源树
样例
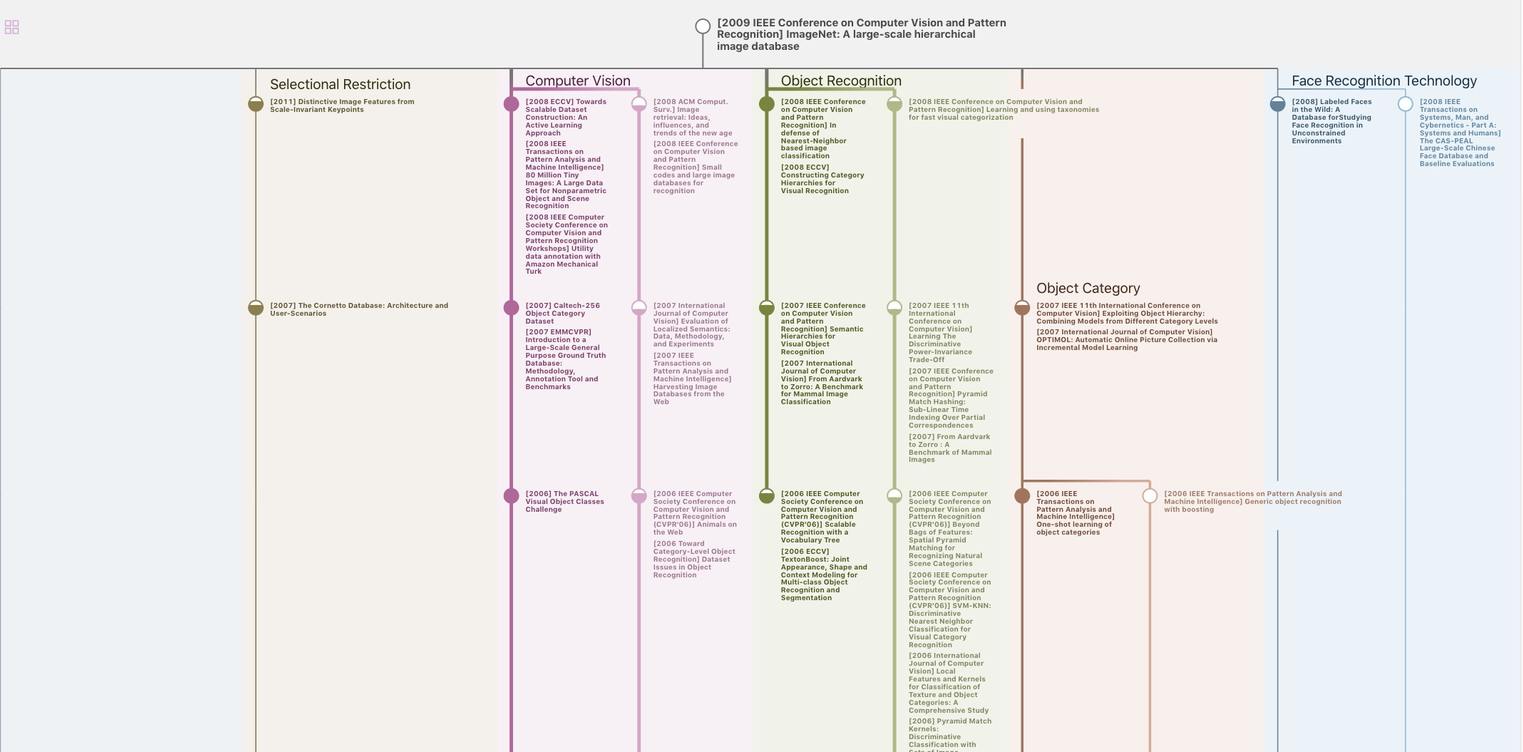
生成溯源树,研究论文发展脉络
Chat Paper
正在生成论文摘要