Accelerating DNN Inference With Reliability Guarantee in Vehicular Edge Computing
IEEE-ACM TRANSACTIONS ON NETWORKING(2023)
摘要
This paper explores on accelerating Deep Neural Network (DNN) inference with reliability guarantee in Vehicular Edge Computing (VEC) by considering the synergistic impacts of vehicle mobility and Vehicle-to-Vehicle/Infrastructure (V2V/V2I) communications. First, we show the necessity of striking a balance between DNN inference acceleration and reliability in VEC, and give insights into the design rationale by analyzing the features of overlapped DNN partitioning and mobility-aware task offloading. Second, we formulate the Cooperative Partitioning and Offloading (CPO) problem by presenting a cooperative DNN partitioning and offloading scenario, followed by deriving an offloading reliability model and a DNN inference delay model. The CPO is proved as NP-hard. Third, we propose two approximation algorithms, i.e., Submodular Approximation Allocation Algorithm (SA3) and Feed Me the Rest algorithm (FMtR). In particular, SA3 determines the edge allocation in a centralized way, which achieves 1/3-optimal approximation on maximizing the inference reliability. On this basis, FMtR partitions the DNN models and offloads the tasks to the allocated edge nodes in a distributed way, which achieves 1/2-optimal approximation on maximizing the inference reliability. Finally, we build the simulation model and give a comprehensive performance evaluation, which demonstrates the superiority of the proposed solutions.
更多查看译文
关键词
Vehicular edge computing,DNN inference acceleration,reliability guarantee,overlapped partitioning,mobility-aware offloading
AI 理解论文
溯源树
样例
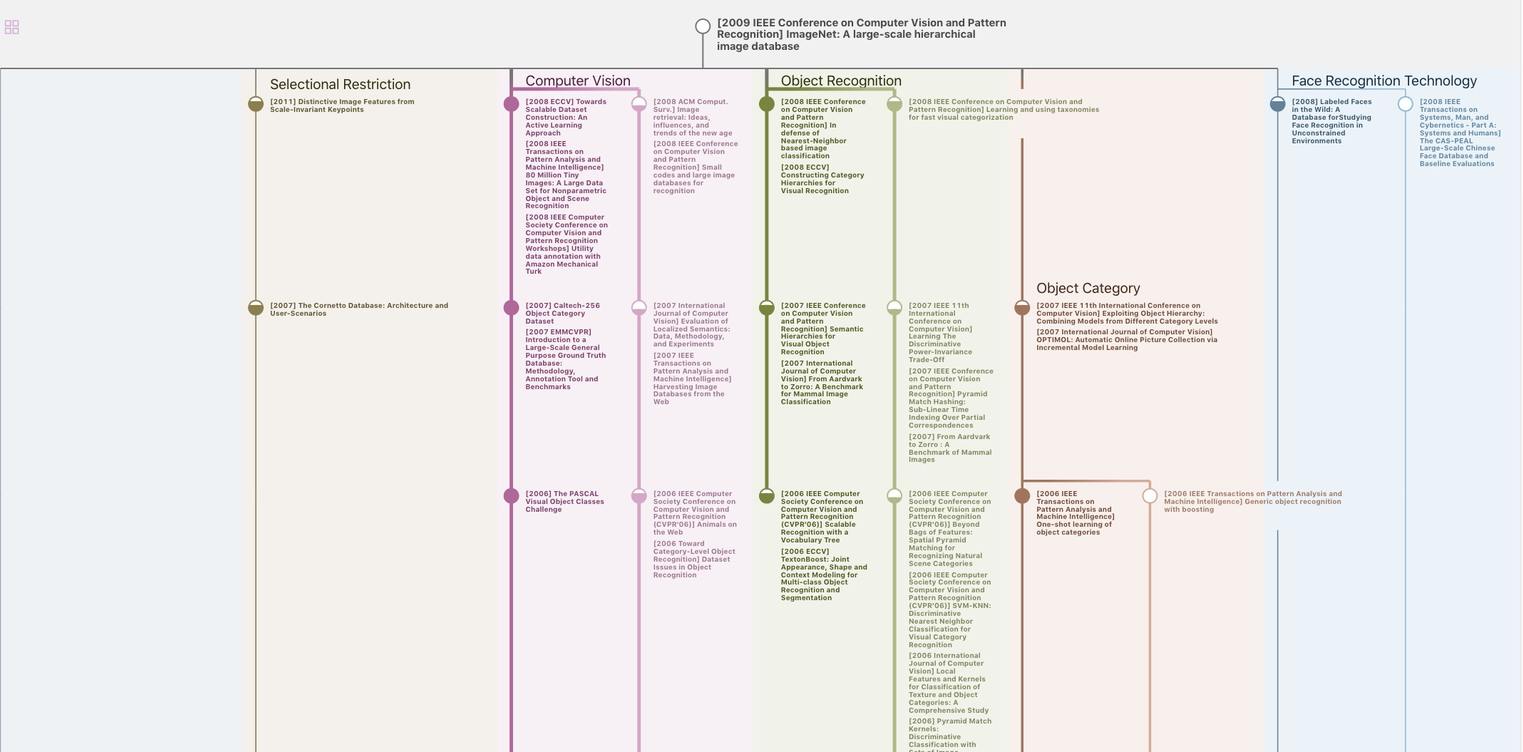
生成溯源树,研究论文发展脉络
Chat Paper
正在生成论文摘要