On the design of KLT-based maximally incoherent deterministic sensing matrices for compressive sensing applied to wireless sensor networks data
WIRELESS NETWORKS(2023)
摘要
The Compressive Sensing (CS) framework explores signal sparsity to reduce the amount of data to transmit or store. In it, signal projections on sensing vectors are used to measure the signal, the set of sensing vectors compose the sensing matrix. Usually, one constructs the sensing matrix to be incoherent to a basis in which the signal is sparse. In this paper, we propose and investigate the use of Karhunen–Loève Transforms (KLTs) as sparsifying bases to construct the corresponding maximally incoherent deterministic sensing matrices. This is done within the context of encoding environmental signals acquired by a Wireless Sensor Network (WSN). The Rate × Distortion (RD) performance of the CS encoding-decoding scheme when the KLT-based deterministic sensing matrices are used is compared against the ones obtained when the CS scheme builds on either the Discrete Cosine Transform (DCT-based) or the K Singular Value Decomposition (K-SVD-based) as sparsifying basis to construct the deterministic sensing matrix. To this end, we also investigate the impact, on the K-SVD-based CS, of the number of elements used to express signals using the K-SVD algorithm. The experimental results show that the proposed KLT-based sensing matrices outperform the DCT- and the K-SVD based alternatives in terms of recovered signal quality, encoding bitrate, and computational cost, which, in WSN applications, is a proxy to energy consumption.
更多查看译文
关键词
Compressive sensing,Deterministic sensing matrix,KLT,K-SVD,Wireless sensor networks
AI 理解论文
溯源树
样例
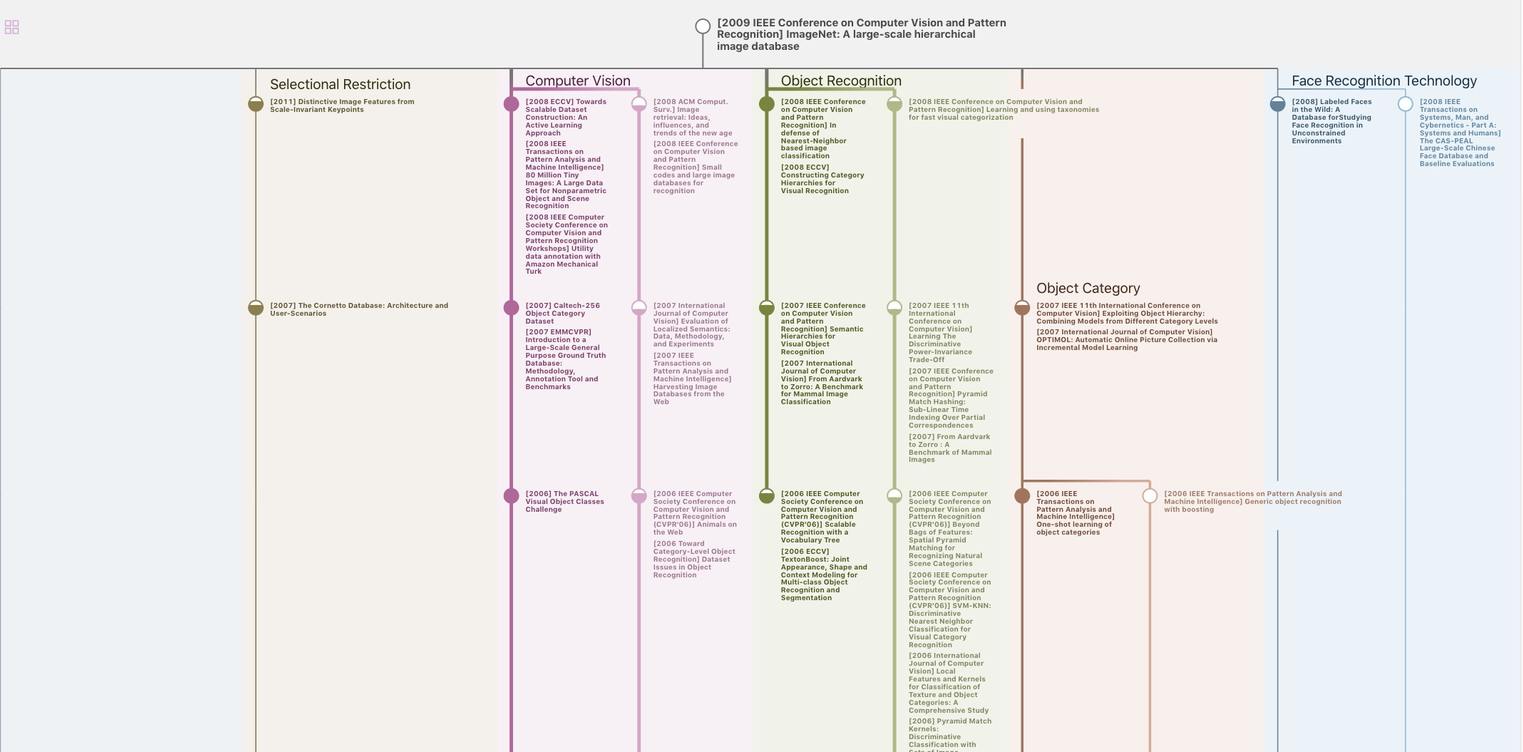
生成溯源树,研究论文发展脉络
Chat Paper
正在生成论文摘要