Evaluating Landslide Susceptibility Using Sampling Methodology and Multiple Machine Learning Models.
ISPRS Int. J. Geo Inf.(2023)
摘要
Landslide susceptibility assessment (LSA) based on machine learning methods has been widely used in landslide geological hazard management and research. However, the problem of sample imbalance in landslide susceptibility assessment, where landslide samples tend to be much smaller than non-landslide samples, is often overlooked. This problem is often one of the important factors affecting the performance of landslide susceptibility models. In this paper, we take the Wanzhou district of Chongqing city as an example, where the total number of data sets is more than 580,000 and the ratio of positive to negative samples is 1:19. We oversample or undersample the unbalanced landslide samples to make them balanced, and then compare the performance of machine learning models with different sampling strategies. Three classic machine learning algorithms, logistic regression, random forest and LightGBM, are used for LSA modeling. The results show that the model trained directly using the unbalanced sample dataset performs the worst, showing an extremely low recall rate, indicating that its predictive ability for landslide samples is extremely low and cannot be applied in practice. Compared with the original dataset, the sample set optimized through certain methods has demonstrated improved predictive performance across various classifiers, manifested in the improvement of AUC value and recall rate. The best model was the random forest model using over-sampling (O_RF) (AUC = 0.932).
更多查看译文
关键词
landslide susceptibility assessment,imbalanced datasets,machine learning,oversampling,undersampling
AI 理解论文
溯源树
样例
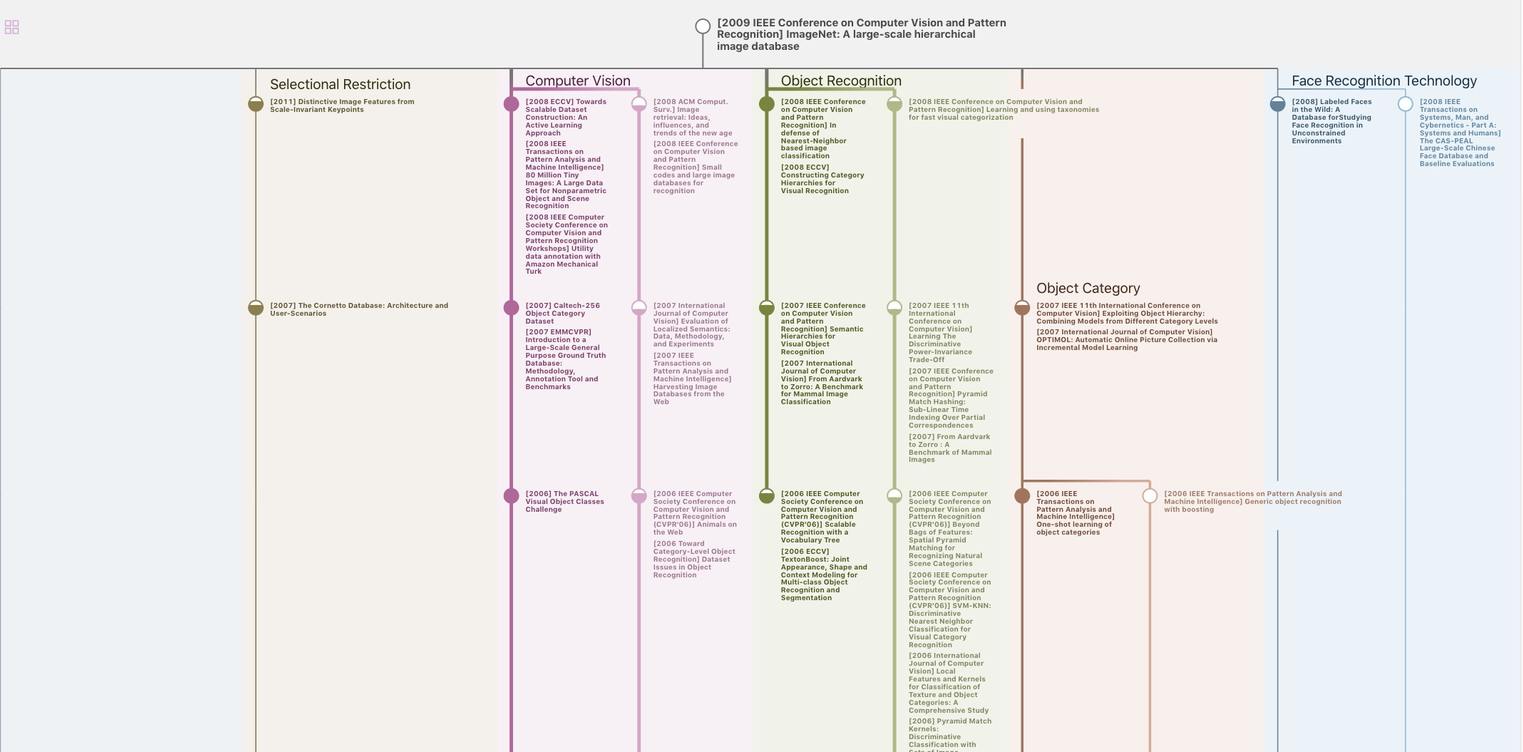
生成溯源树,研究论文发展脉络
Chat Paper
正在生成论文摘要