Conformal Predictive Distribution Trees
ANNALS OF MATHEMATICS AND ARTIFICIAL INTELLIGENCE(2023)
摘要
Being able to understand the logic behind predictions or recommendations on the instance level is at the heart of trustworthy machine learning models. Inherently interpretable models make this possible by allowing inspection and analysis of the model itself, thus exhibiting the logic behind each prediction, while providing an opportunity to gain insights about the underlying domain. Another important criterion for trustworthiness is the model’s ability to somehow communicate a measure of confidence in every specific prediction or recommendation. Indeed, the overall goal of this paper is to produce highly informative models that combine interpretability and algorithmic confidence. For this purpose, we introduce conformal predictive distribution trees, which is a novel form of regression trees where each leaf contains a conformal predictive distribution. Using this representation language, the proposed approach allows very versatile analyses of individual leaves in the regression trees. Specifically, depending on the chosen level of detail, the leaves, in addition to the normal point predictions, can provide either cumulative distributions or prediction intervals that are guaranteed to be well-calibrated. In the empirical evaluation, the suggested conformal predictive distribution trees are compared to the well-established conformal regressors, thus demonstrating the benefits of the enhanced representation.
更多查看译文
关键词
Conformal predictive distributions,Interpretability,Regression trees,Conformal regression
AI 理解论文
溯源树
样例
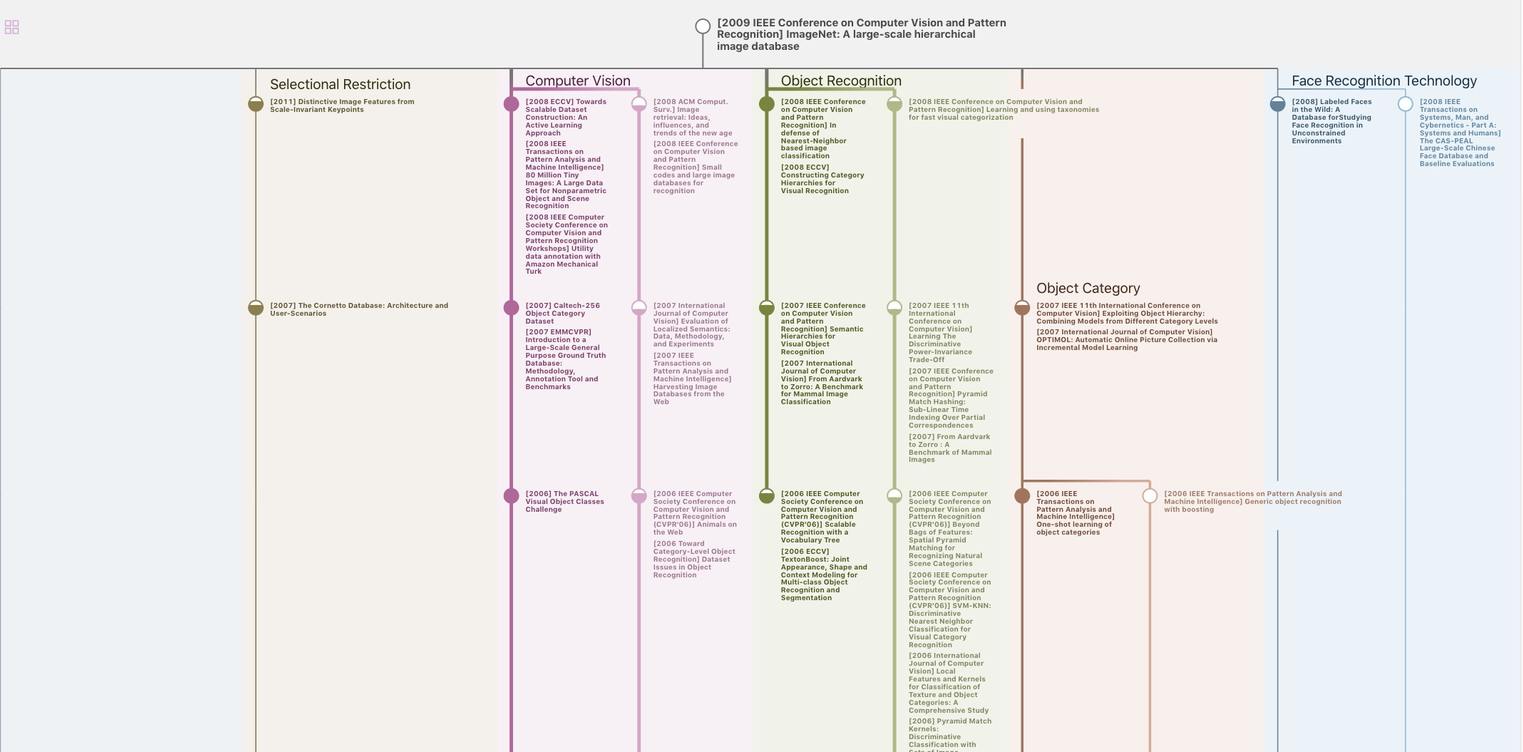
生成溯源树,研究论文发展脉络
Chat Paper
正在生成论文摘要