Common Latent Embedding Space for Cross-Domain Facial Expression Recognition
IEEE TRANSACTIONS ON COMPUTATIONAL SOCIAL SYSTEMS(2023)
摘要
In practical facial expression recognition (FER), the training data and test data are often obtained from different domains. It is obvious that the domain disparity could significantly degrade the recognition performance. To tackle this challenging cross-domain FER problem, we put forward a novel method termed common latent embedding space (CLES). To be specific, first, we obtain a common embedding space for cross-domain samples by matrix factorization (MF). Then, the dual-graph Laplacian is applied to this common embedding space to narrow the gap across distinct domains and, meanwhile, explores the inherent geometric information. Furthermore, to characterize the global relationship of the cross-domain samples, the self-representation strategy is used to guide the learning of the common embedding space. Finally, comprehensive experiments on four benchmark databases indicate that the proposed method can achieve better performance in comparison with the state-of-the-art methods on cross-domain FER tasks.
更多查看译文
关键词
Feature extraction,Face recognition,Sparse matrices,Matrix decomposition,Transfer learning,Task analysis,Laplace equations,Domain adaptation,facial expression recognition (FER),latent embedding space
AI 理解论文
溯源树
样例
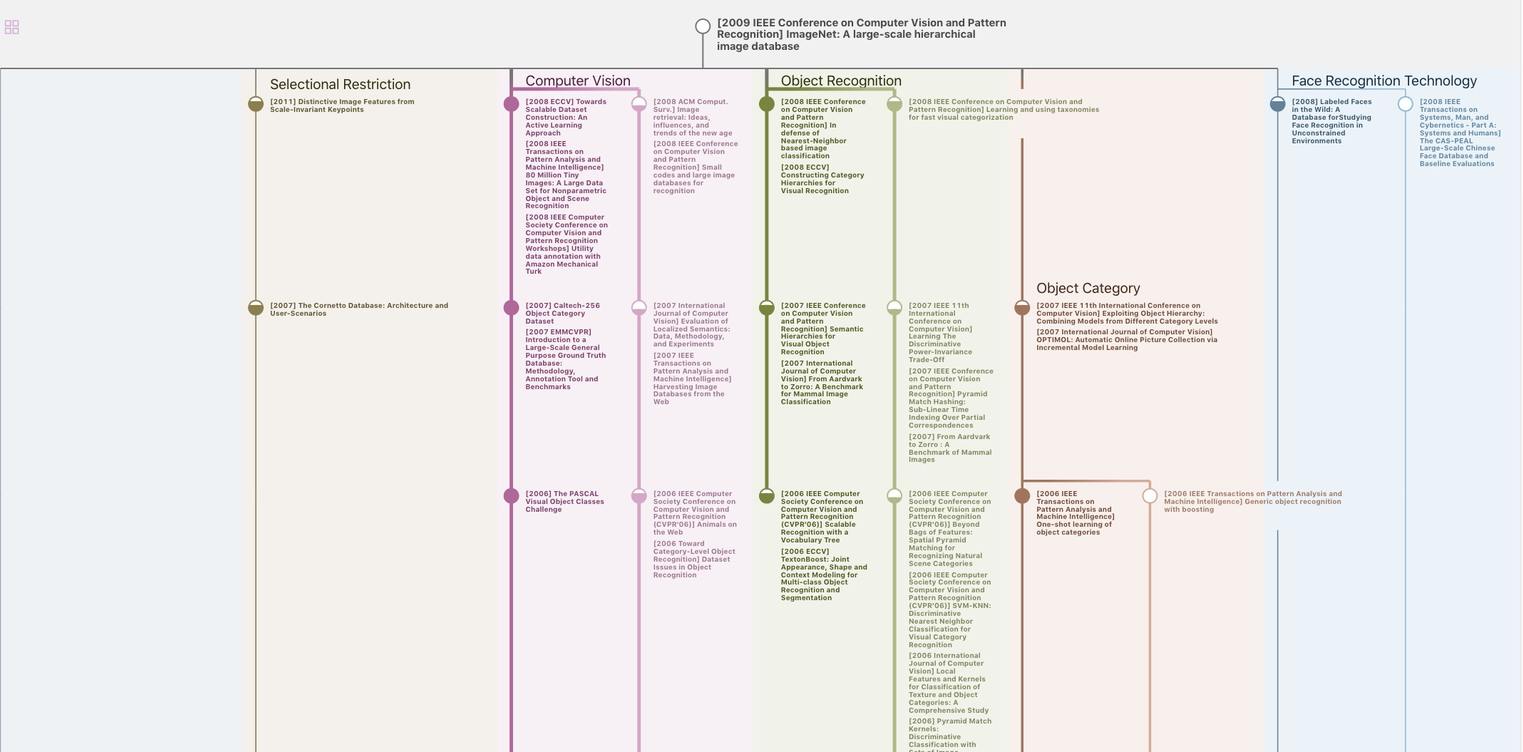
生成溯源树,研究论文发展脉络
Chat Paper
正在生成论文摘要