Adaptive affinity matrix learning for dimensionality reduction
INTERNATIONAL JOURNAL OF MACHINE LEARNING AND CYBERNETICS(2023)
摘要
Conventional graph-based dimensionality reduction methods treat graph leaning and subspace learning as two separate steps, and fix the graph during subspace learning. However, the graph obtained from the original data may be not optimal, because the original high-dimensional data contains redundant information and noise, thus the subsequent subspace learning based on the graph may be affected. In this paper, we propose a model called adaptive affinity matrix learning (AAML) for unsupervised dimensionality reduction. Different from traditional graph-based methods, we integrate two steps into a unified framework and adaptively adjust the learned graph. To obtain an ideal neighbor assignment, we introduce a rank constraint to the Laplacian matrix of the affinity matrix. In this way, the number of connected components of the graph is exactly equal to the number of class numbers. By approximating two low-dimensional subspaces, the affinity matrix can obtain the original neighbor structure from the similarity matrix, and the projection matrix can get low-rank information from the affinity matrix, then a distinctive subspace can be learned. Moreover, we propose an efficient algorithm to solve the optimization problem of AAML. Experimental results on four data sets show the effectiveness of the proposed model.
更多查看译文
关键词
Dimensionality reduction,Graph-based learning,Classification,Unsupervised learning
AI 理解论文
溯源树
样例
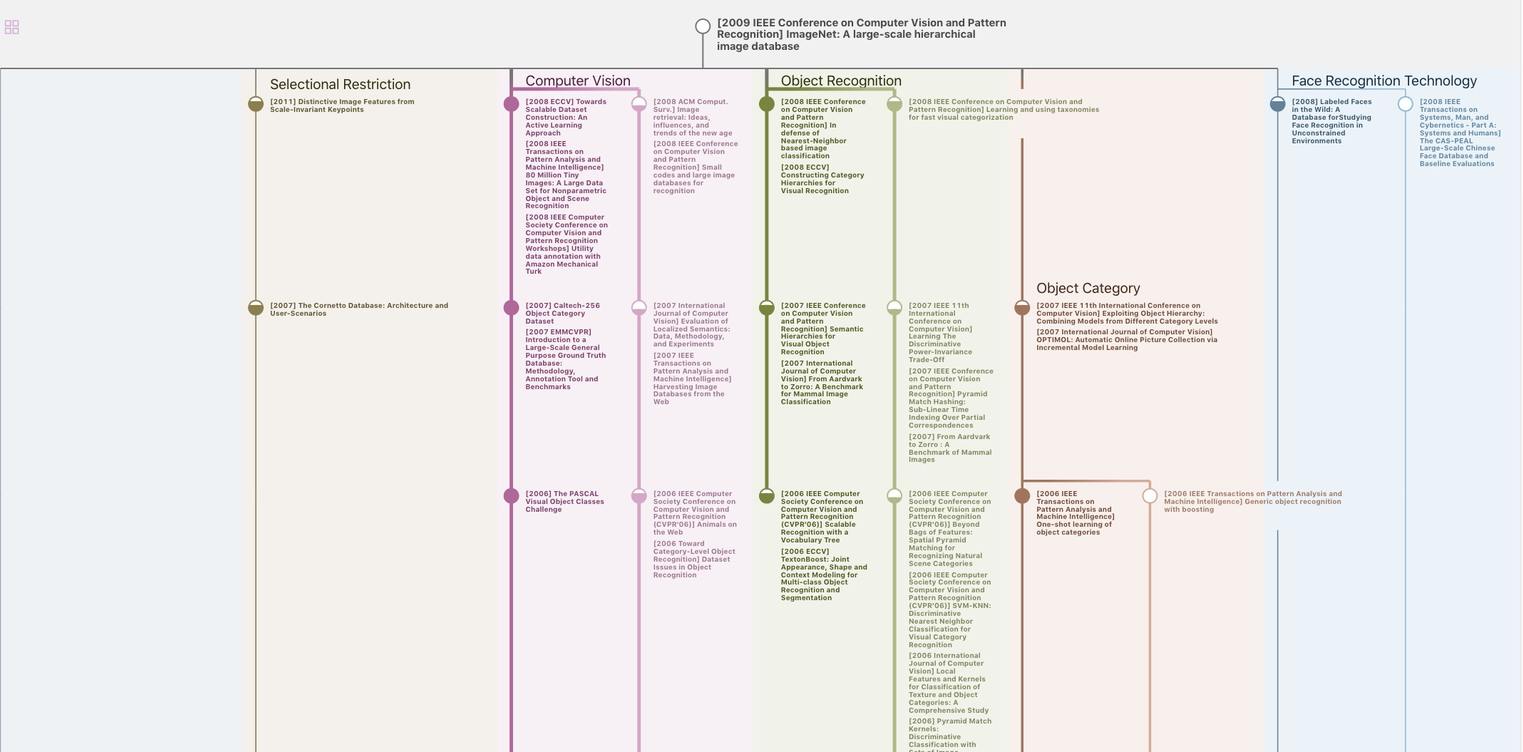
生成溯源树,研究论文发展脉络
Chat Paper
正在生成论文摘要