Machine learning integrated patient flow simulation: why and how?
JOURNAL OF SIMULATION(2023)
摘要
Stochastic distribution methods were used to construct patient flow simulation sub-models such as patient inflow, length of stay (LoS), cost of treatment (CoT) and clinical pathways (CPs). However, the patient inflow rate demonstrates seasonality, trend, and variation due to natural and human-made factors. LoS, CoT and CPs are determined by social-demographics factors, clinical and laboratory test results, resource availability and healthcare structure. For this reason, patient flow simulation models developed using stochastic methods have limitations including uncertainty, not recognising patient heterogeneity, and not representing personalised and value-based healthcare. This, in turn, results in a low acceptance level and implementation of solutions suggested by patient flow simulation models. On the other hand, machine learning becomes effective in predicting patient inflow, LoS, CoT, and CPs. This paper, therefore, describes why coupling machine learning with patient flow simulation is important, proposes a conceptual architecture for machine learning integrated patient flow simulation and demonstrates its implementation with examples.
更多查看译文
关键词
patient flow simulation,machine learning
AI 理解论文
溯源树
样例
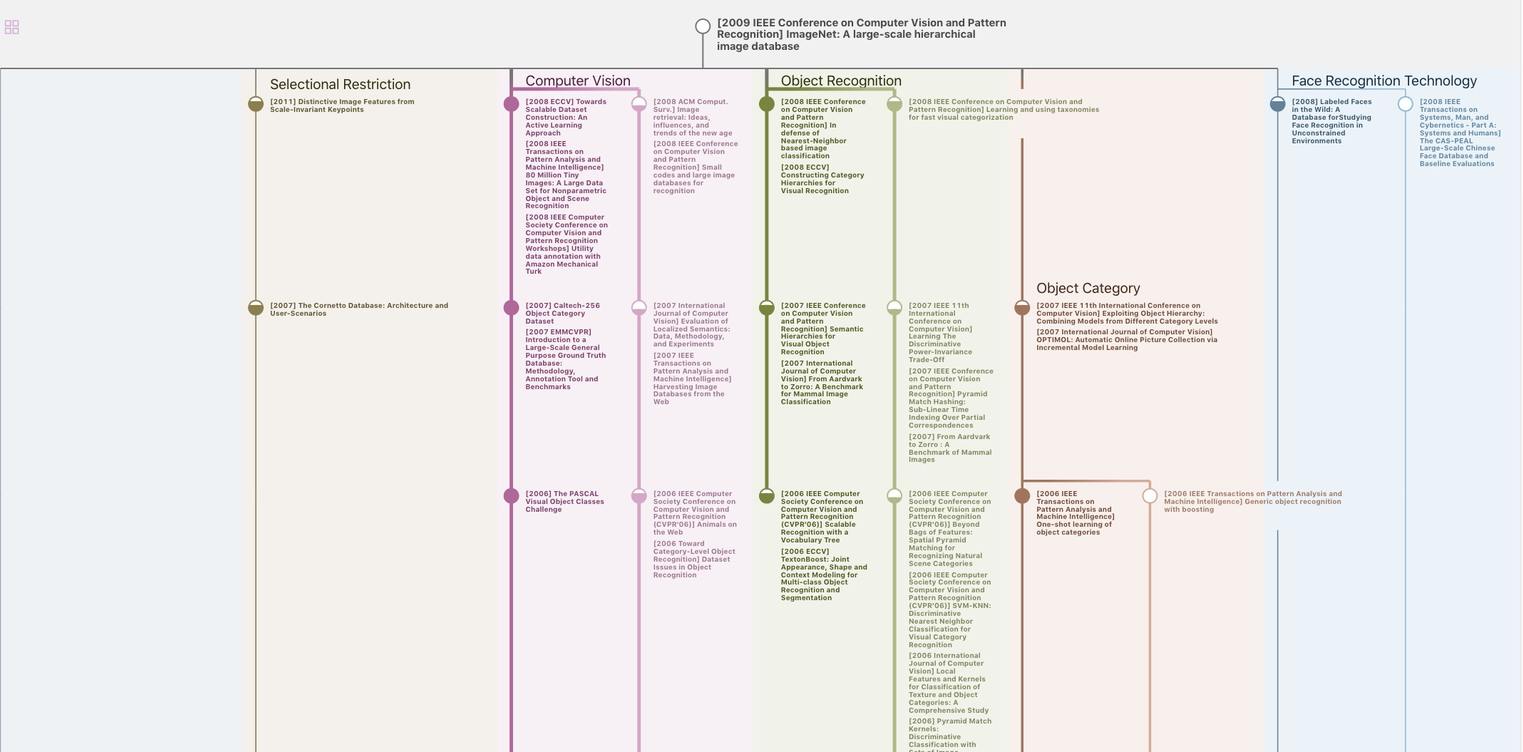
生成溯源树,研究论文发展脉络
Chat Paper
正在生成论文摘要