Risk-averse optimization and resilient network flows
NETWORKS(2023)
摘要
We propose an approach to constructing metrics of network resilience, where resilience is understood as the network's amenability to restoring its optimal or near-optimal operations subsequent to unforeseen (stochastic) disruptions of its topology or operational parameters, and illustrated it on the examples of the resilient maximum network flow problem and the resilient minimum cost network problem. Specifically, the network flows in these problems are designed for resilience against unpredictable losses of network carrying capacity, and the mechanism of attaining a degree of resilience is through preallocation of resources toward (at least partial) restoration of the capacities of the arcs. The obtained formulations of resilient network flow problems possess a number of useful properties, for example, similarly to the standard network flow problems, the network flow is integral if the arc capacities, costs, and so forth, are integral. It is also shown that the proposed formulations of resilient network flow problems can be viewed as "network measures of risk", similar in properties and behavior to convex measures of risk. Efficient decomposition algorithms have been proposed for both the resilient maximum network flow problem and the resilient minimum cost network flow problem, and a study of the network flow resilience as a function of network's structure has been conducted on networks with three types of topology: that of uniform random graphs, scale-free graphs, and grid graphs.
更多查看译文
关键词
benders decomposition,convex risk measures,resilient maximum network flow problem,resilient minimum cost network flow problem,stochastic network
AI 理解论文
溯源树
样例
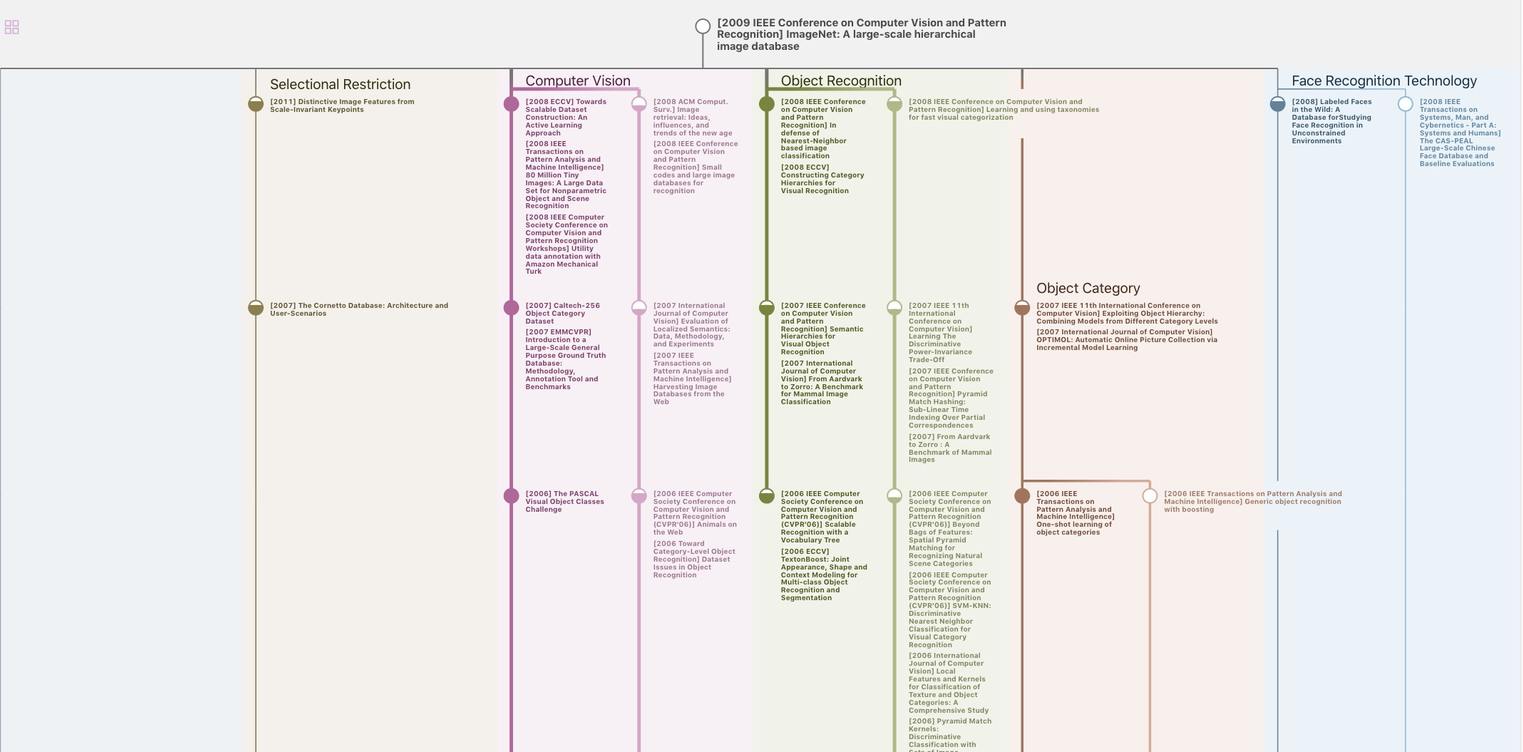
生成溯源树,研究论文发展脉络
Chat Paper
正在生成论文摘要