Deep Learning CNN Model-based Anomaly Detection in 3D Brain MRI Images using Feature Distribution Similarity
INTERNATIONAL JOURNAL OF ADVANCED COMPUTER SCIENCE AND APPLICATIONS(2023)
摘要
Towards detecting an anomaly in brain images, different approaches are discussed in the literature. Features like white mass values and shape features have identified the presence of brain tumors. Various deep learning models like the neural network has been adapted to the problem tumor detection and suffers to meet maximum accuracy in detecting brain tumor. An Adaptive Feature Centric Distribution Similarity Based Anomaly Detection Model with Convolution Neural Network (AFCD-CNN) is sketched towards disease prediction problem to handle the problem. The model considers black-and-white mass features with the distribution of features. First, the method applies the Multi-Hop Neighbor Analysis (MHNA) algorithm in normalizing the brain image. Further, the process uses the which groups the pixels of MRI according to the white and black mass values. The method extracts the ROI with the segmented image and convolves the features with CNN at the training phase. The CNN is designed to convolve the features into one dimension. The output layer neurons are designed to estimate different Feature Distribution Similarity (FDS) values against various According to the ACW value, anomaly detection is performed with higher accuracy up to 97% where the time complexity is reduced up to 32 seconds.
更多查看译文
关键词
Deep learning, brian tumor, disease prediction, anomaly detection, CNN, FDS, ACW
AI 理解论文
溯源树
样例
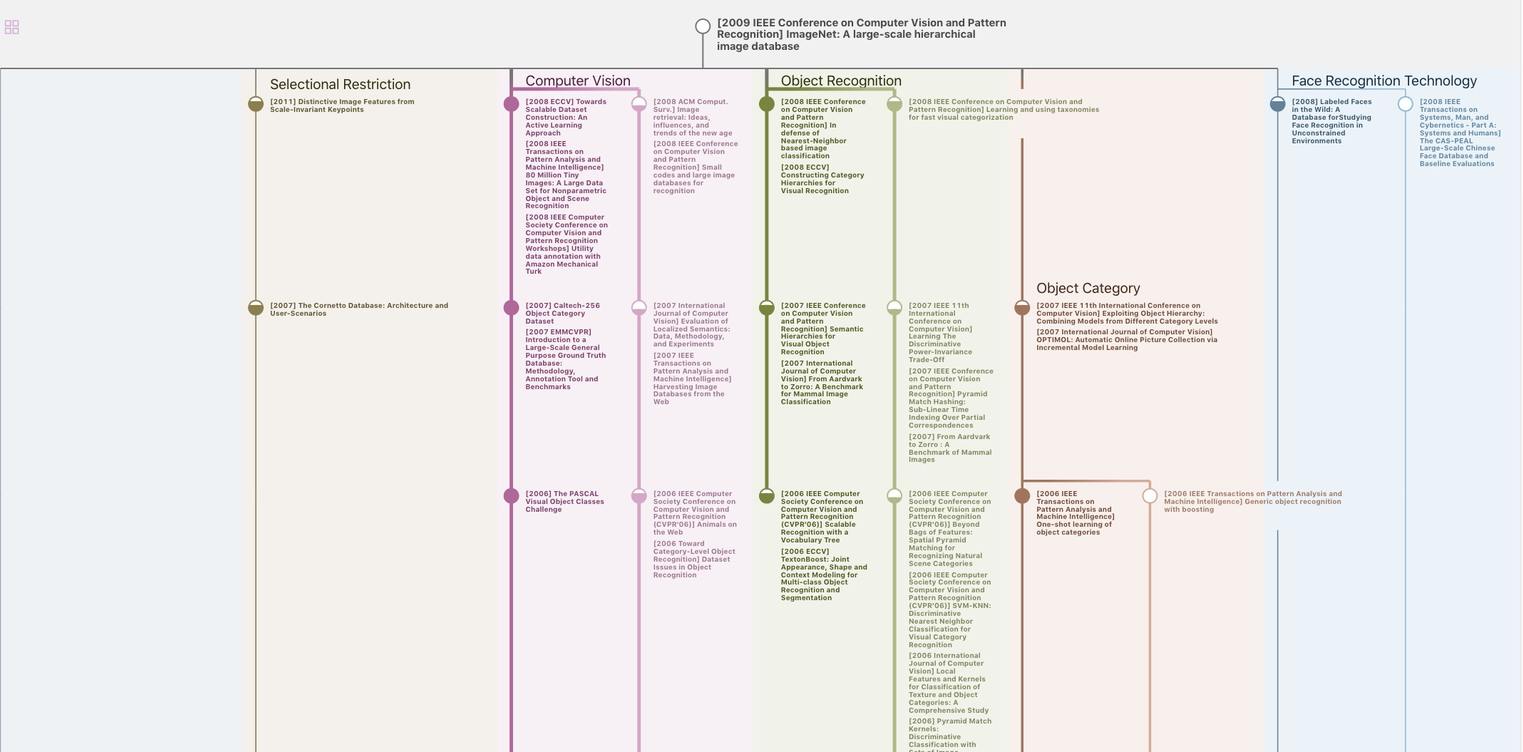
生成溯源树,研究论文发展脉络
Chat Paper
正在生成论文摘要