A New Ontology Convolutional Neural Network for Extorting Essential Elements in Video Mining
JOURNAL OF SIGNAL PROCESSING SYSTEMS FOR SIGNAL IMAGE AND VIDEO TECHNOLOGY(2023)
摘要
Nowadays, people use video compression for recreating video without affecting the quality with reduced size. In recent years, the number of video files has increased in social media, smartphones and video recording tools. It is not easy to search and retrieve specific content-based videos. With the use of advanced techniques, the video clips were retrieved based on an object, themes, people and other entities. Video communication tolerates a lot of problems despite using a restricted volume of cutting-edge methodology to accumulate huge-sized videos. For this motivation, the video compression technique is used. Extracting semantic data from a large number of video-based applications is necessary. These advanced techniques were mainly used in digital marketing, social marketing, and video editing. The semantic data analysis is aimed to extract the video by expressing it in text or speech. It is used to understand the content of the video and extract it in the form of footage or video clips through a query. The existing methodologies are insufficient and high amount of optimization cost. The speedy expansion in the available quantity of video information has increased an essential constraint to lengthen. Intellectual methodologies are used to construct and extort the semantic substance. In this paper, Convolutional Neural Network with VGGNet is developed for extorting essential elements in videos and for spatial modification within the frames. Rule-related information employs temporal associations to extort the characterizations. The dynamic movements are extorted by the Optical stream algorithm and it finds the temporal positions. The new algorithm is experimentally validated.
更多查看译文
关键词
Video compression,Convolutional Neural Network,Ontology Model,Temporal Position,Semantic contents
AI 理解论文
溯源树
样例
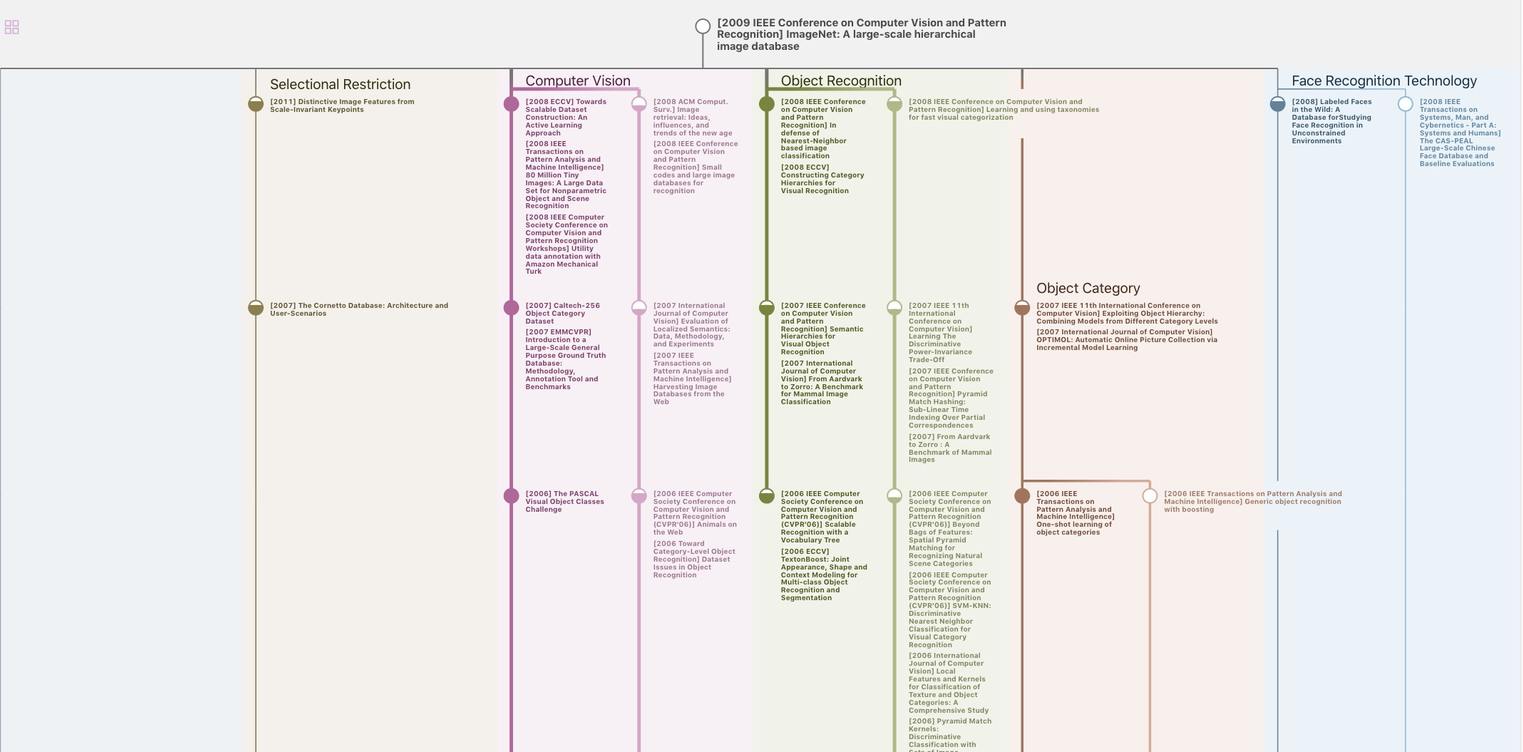
生成溯源树,研究论文发展脉络
Chat Paper
正在生成论文摘要