Collapsed Building Detection in High-Resolution Remote Sensing Images Based on Mutual Attention and Cost Sensitive Loss
IEEE GEOSCIENCE AND REMOTE SENSING LETTERS(2023)
摘要
Detection of collapsed buildings based on high-resolution remote sensing images is of significance to post-earthquake emergency response and post-disaster reconstruction. In recent years, the UNet network architecture has been applied in the detection of collapsed buildings to good effect. However, most existing methods directly fuse features of encoder and decoder subnets, which results in redundant or mutually exclusive information, thus weakening the capability of expressing details of the outline of collapsed buildings in detail. In addition, using these methods, the collapsed building samples in post-earthquake damage scenes are difficult to be acquired or account for a small proportion of the total detected image. These approaches indistinguishably processed the misclassified samples with different types of training processes, while ignoring the enhanced learning of collapsed building samples. Hence, a multimodule concatenation based on the U-Shape network (MC-UNet) for detecting post-earthquake collapsed buildings based on a mutual attention module (MAM) and cost-sensitive loss (CS loss) was proposed. To begin, a MAM was designed in skip connections in UNet to fully utilize high-level and low-level feature information, as a result, feature expressive capability can be enhanced; on this basis, a novel CS loss was designed to force the model to focus more on the learning of collapsed building samples. Experiments on three groups of datasets (Mexico dataset, HTI dataset, and YSH dataset) about post-earthquake collapsed buildings indicate the scheme proposed remarkably outperforms some state-of-the-art algorithms.
更多查看译文
关键词
remote sensing,images based,detection,high-resolution
AI 理解论文
溯源树
样例
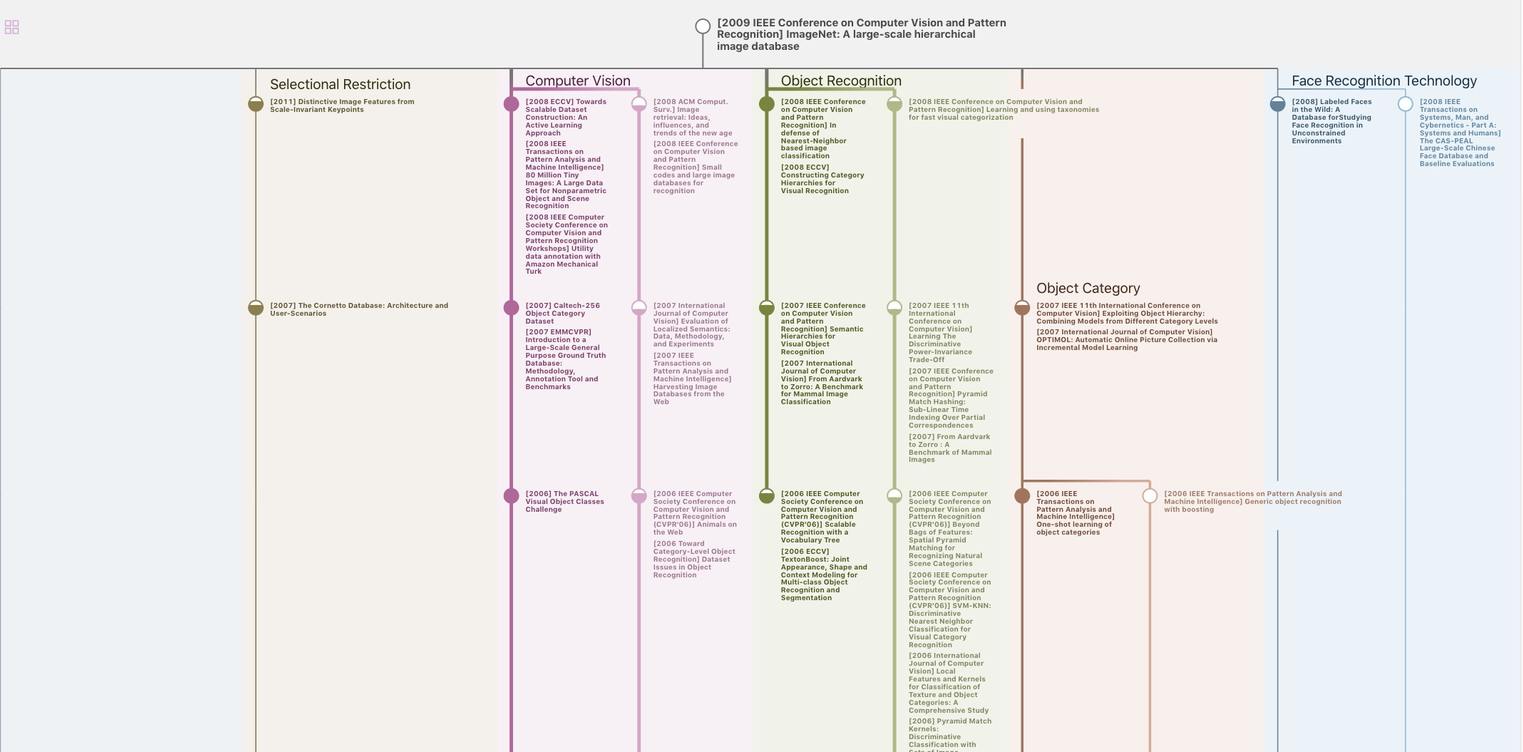
生成溯源树,研究论文发展脉络
Chat Paper
正在生成论文摘要