Connections between Robust Statistical Estimation, Robust Decision-Making with Two-Stage Stochastic Optimization, and Robust Machine Learning Problems
CYBERNETICS AND SYSTEMS ANALYSIS(2023)
摘要
The authors discuss connections between the problems of two-stage stochastic programming, robust decision-making, robust statistical estimation, and machine learning. In the conditions of uncertainty, possible extreme events and outliers, these problems require quantile-based criteria, constraints, and “goodness-of-fit” indicators. The two-stage stochastic optimization (STO) problems with quantile-based criteria can be effectively solved with the iterative stochastic quasigradient (SQG) solution algorithms. The SQG methods provide a new type of machine learning algorithms that can be effectively used for general-type nonsmooth, possibly discontinuous, and nonconvex problems, including quantile regression and neural network training. In general problems of decision-making, feasible solutions and concepts of optimality and robustness are characterized from the context of decision-making situations. Robust machine learning (ML) approaches can be integrated with disciplinary or interdisciplinary decision-making models, e.g., land use, agricultural, energy, etc., for robust decision-making in the conditions of uncertainty, increasing systemic interdependencies, and “unknown risks.”
更多查看译文
关键词
robust statistical estimation,machine learning,optimization,decision-making,two-stage
AI 理解论文
溯源树
样例
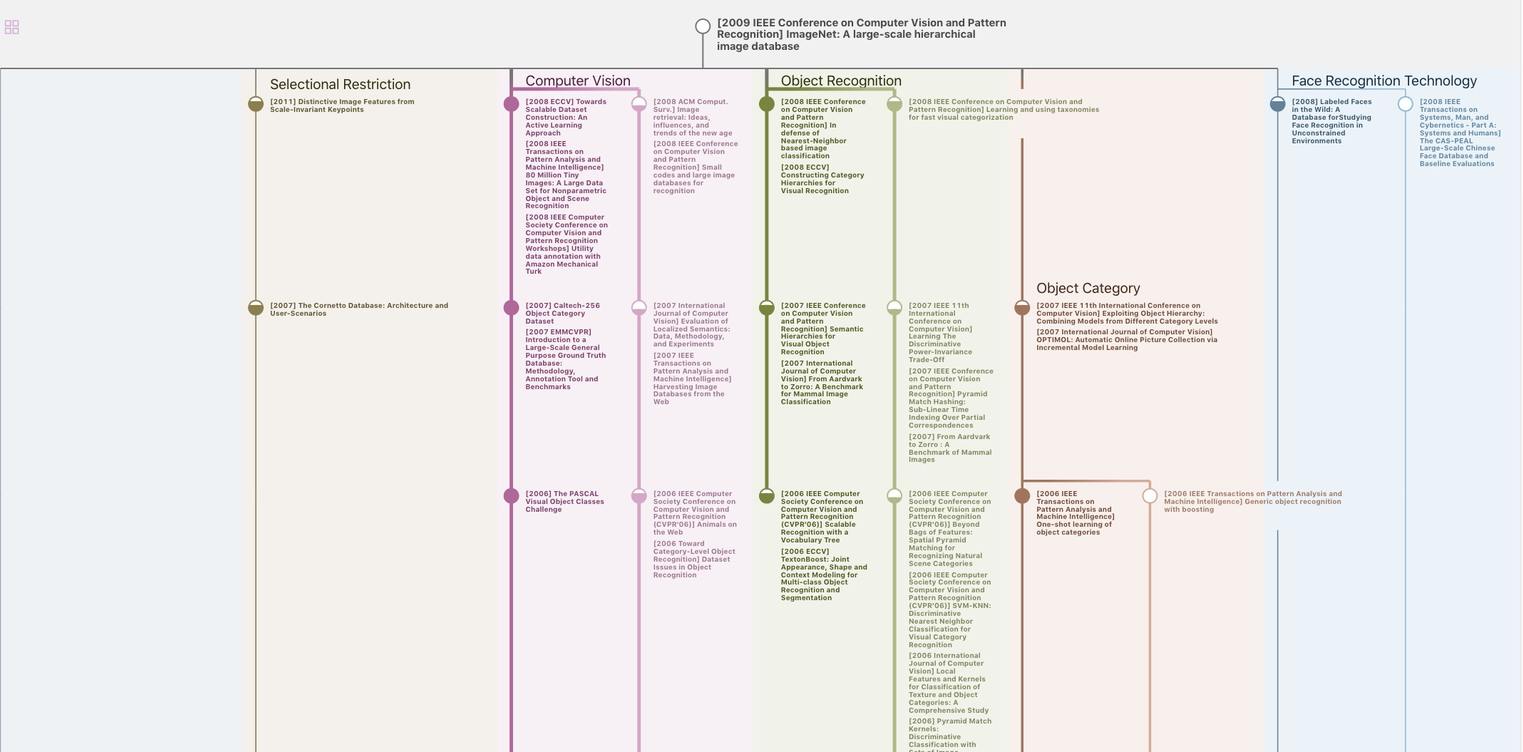
生成溯源树,研究论文发展脉络
Chat Paper
正在生成论文摘要