ParallelEye Pipeline: An Effective Method to Synthesize Images for Improving the Visual Intelligence of Intelligent Vehicles
IEEE TRANSACTIONS ON SYSTEMS MAN CYBERNETICS-SYSTEMS(2023)
摘要
Virtual simulated scenes are becoming a critical part of autonomous driving. In the context of knowledge automation and machine learning, simulated images are widely used for visual environmental perception. However, even the most inspirational applications have not fully exploited the potential of simulated images in solving real-world problems. In this article, we propose a novel framework "ParallelEye Pipeline", which uses image-to-image translation and simulated images to automatically generate realistic synthetic images with multiple ground-truth annotations. Specifically, this method has three steps: first, we use Unity3D software to simulate driving scenarios and generate simulated image pairs (including raw images and six ground-truth labels) from the simulated scenes; second, advanced image-to-image translation algorithms can generate realistic and high-resolution synthetic images from simulated image pairs; third, we exploit publicly datasets, simulated images, and synthetic images to conduct experiments for visual perception. The experimental results suggest: 1) synthetic images and simulated images can improve the performance of detectors in real autonomous driving scenarios and 2) image-to-image translation algorithms can be affected by occlusion condition.
更多查看译文
关键词
Annotations,Pipelines,Autonomous vehicles,Generative adversarial networks,Task analysis,Semantics,Visualization,Generative adversarial network (GAN),intelligent vehicles,object detection,simulated scene,synthetic image
AI 理解论文
溯源树
样例
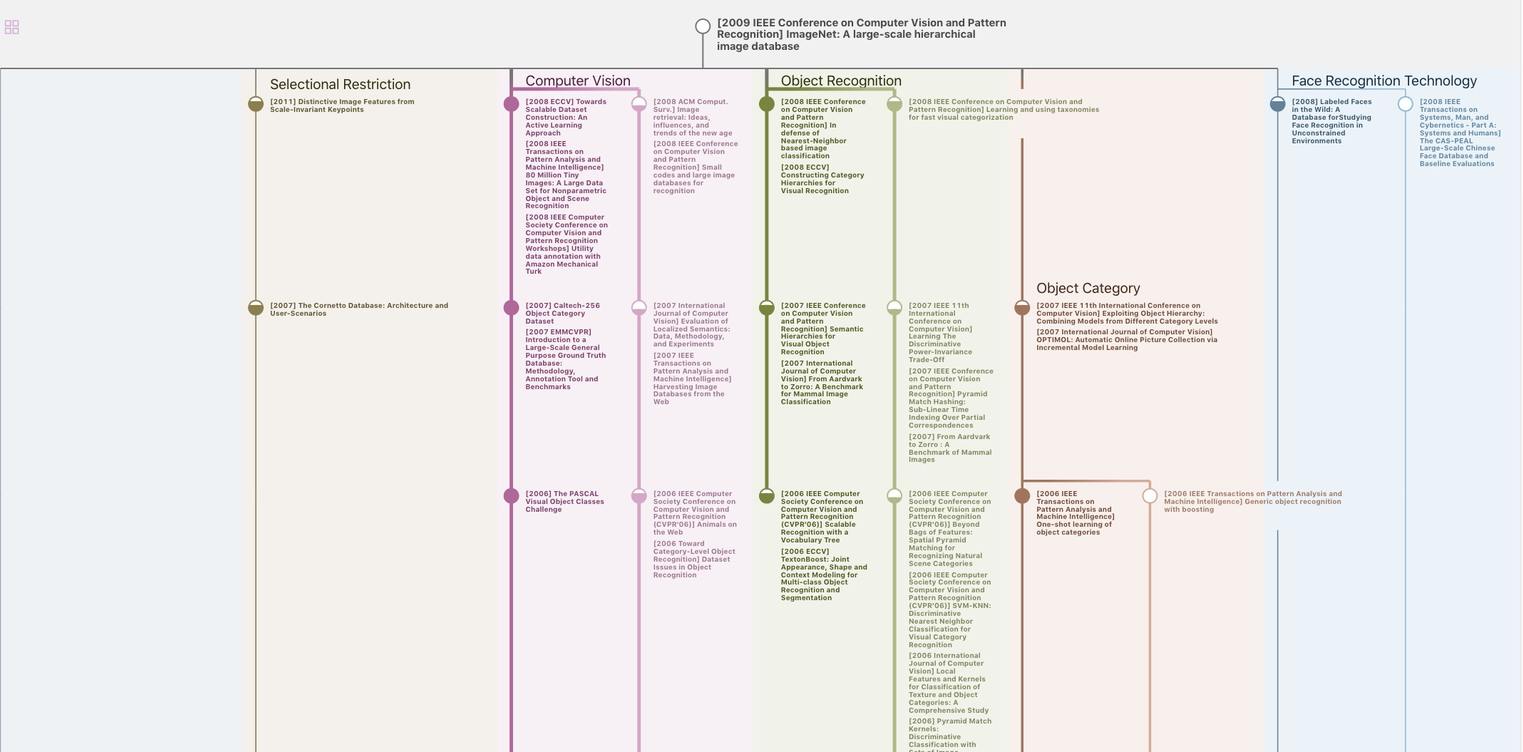
生成溯源树,研究论文发展脉络
Chat Paper
正在生成论文摘要