A Novel Projection Neural Network for Solving a Class of Monotone Variational Inequalities
IEEE TRANSACTIONS ON SYSTEMS MAN CYBERNETICS-SYSTEMS(2023)
摘要
This article provides a novel projection neural network (PNN) for a category of monotone variational inequality (MVI). For simplifying calculation, the feasible region of MVI is separated into two parts: one is the set on which projection has quantitative expression, and the rest part can be described by inequalities. A novel continuous-time PNN is well designed in this article based on the division and its states are verified to exist and ultimately converge to a solution of MVI. The proposed PNN differs from conventional approaches since it only depends on the projection operator on the sets with projection-quantifiable structure instead of the whole feasible region. The feature determines the PNN here owns the advantage of low amount of calculation and flexibility when encountering diverse MVIs. Compared with existing numerical algorithms, model convergence in this article is established on weaker assumptions and one can monitor its state at every moment. Finally, the effectiveness and the efficiency of PNN are testified by several simulation examples and an application in image fusion.
更多查看译文
关键词
Neural networks,Convergence,Optimization,Mathematical models,Real-time systems,Convex functions,Predictive models,global solution,monotone variational inequality (MVI),projection neural network (PNN)
AI 理解论文
溯源树
样例
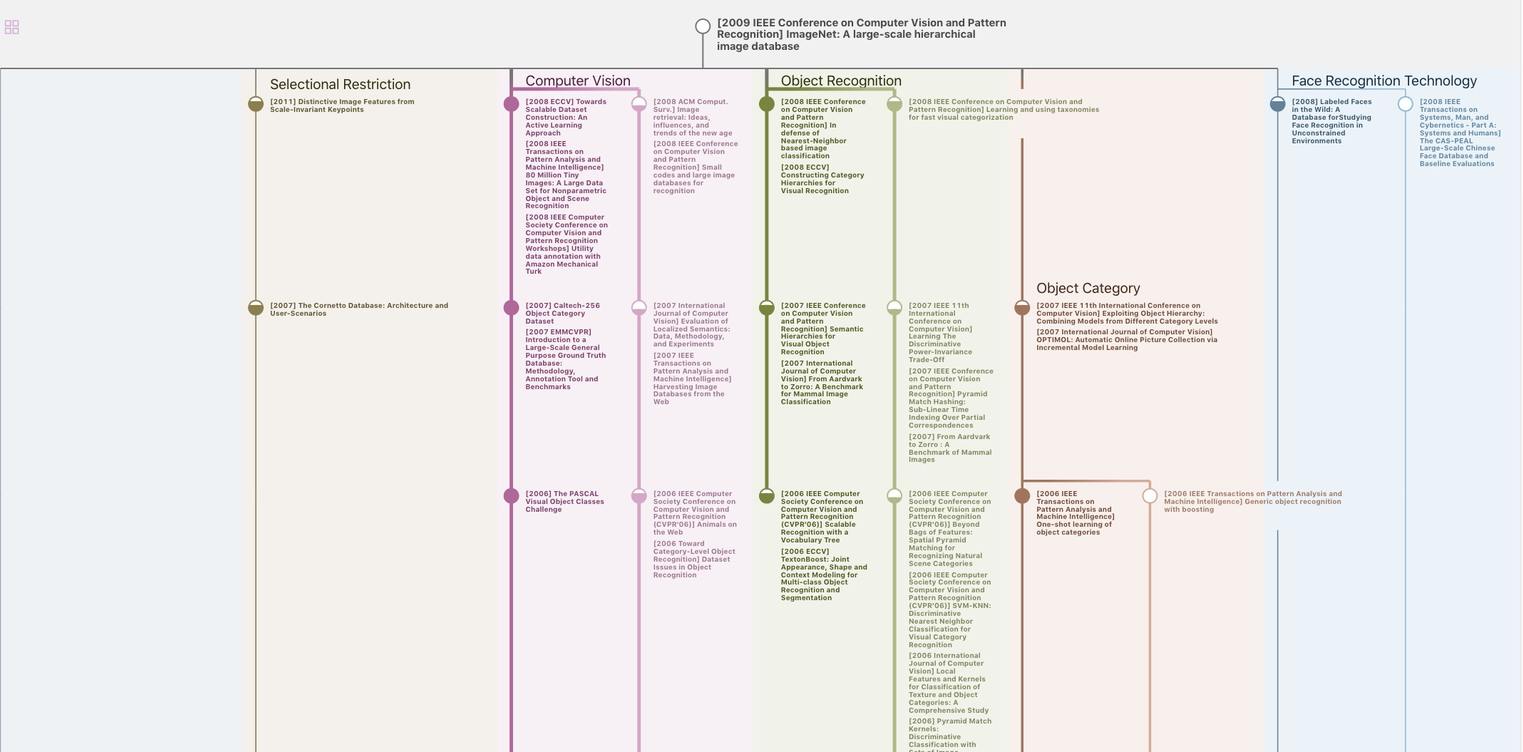
生成溯源树,研究论文发展脉络
Chat Paper
正在生成论文摘要