PF-YOLOv4-Tiny: Towards Infrared Target Detection on Embedded Platform
INTELLIGENT AUTOMATION AND SOFT COMPUTING(2023)
摘要
Infrared target detection models are more required than ever before to be deployed on embedded platforms, which requires models with less memory consumption and better real-time performance while considering accuracy. To address the above challenges, we propose a modified You Only Look Once (YOLO) algorithm PF-YOLOv4-Tiny. The algorithm incorpo-rates spatial pyramidal pooling (SPP) and squeeze-and-excitation (SE) visual attention modules to enhance the target localization capability. The PANet-based-feature pyramid networks (P-FPN) are proposed to transfer semantic information and location information simultaneously to ameliorate detection accuracy. To lighten the network, the standard convolutions other than the backbone network are replaced with depthwise separable convolutions. In post-processing the images, the soft-non-maximum suppression (soft-NMS) algorithm is employed to subside the missed and false detection problems caused by the occlusion between targets. The accuracy of our model can finally reach 61.75%, while the total Params is only 9.3 M and GFLOPs is 11. At the same time, the inference speed reaches 87 FPS on NVIDIA GeForce GTX 1650 Ti, which can meet the requirements of the infrared target detection algorithm for the embedded deployments.
更多查看译文
关键词
Infrared target detection,visual attention module,spatial pyramid pooling,dual-path feature fusion,depthwise separable convolution,soft-NMS
AI 理解论文
溯源树
样例
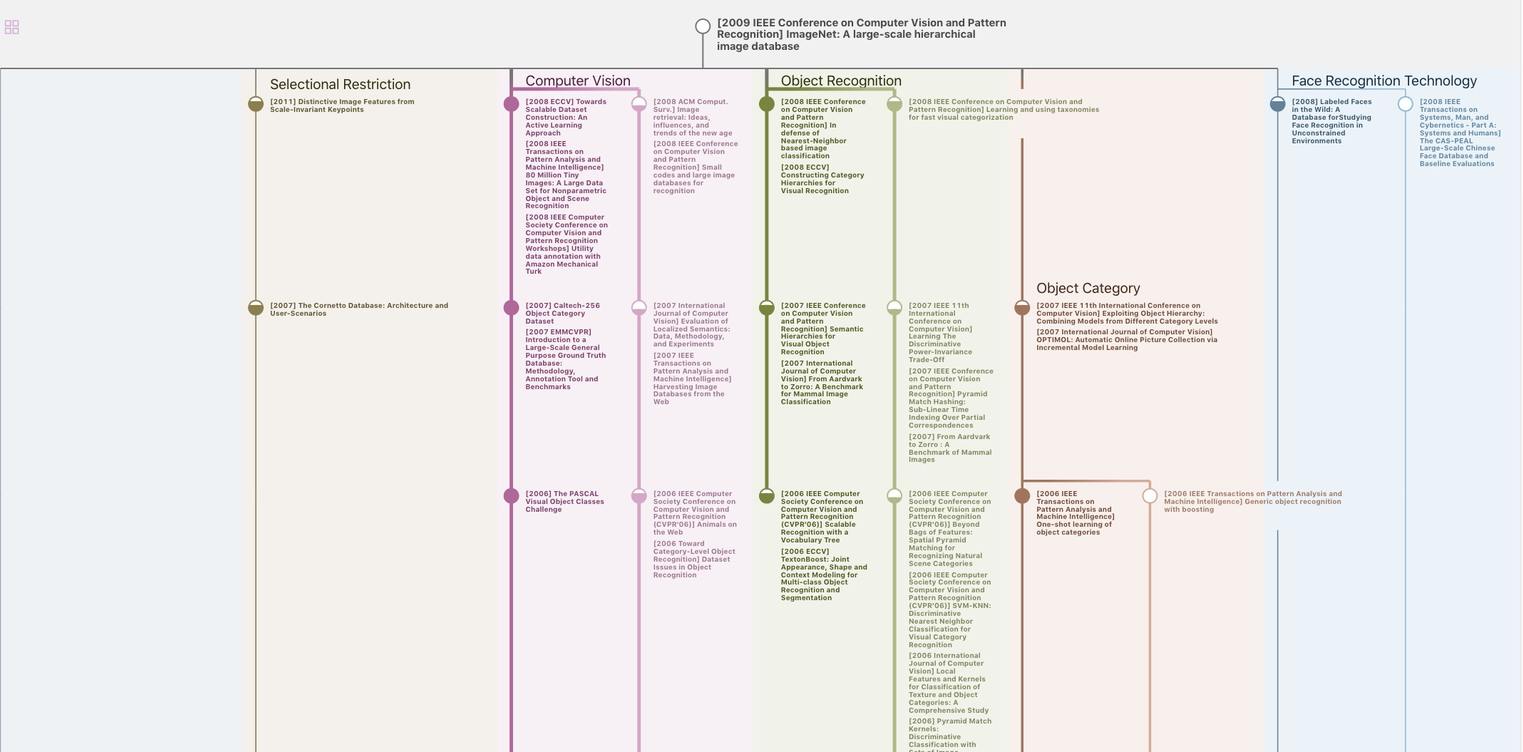
生成溯源树,研究论文发展脉络
Chat Paper
正在生成论文摘要