Day ahead carbon emission forecasting of the regional National Electricity Market using machine learning methods
ENGINEERING APPLICATIONS OF ARTIFICIAL INTELLIGENCE(2023)
摘要
Accurate forecasting of regional electrical grid carbon emissions is an essential part of demand response programs for emissions reduction. Most existing research for short-term (day ahead) forecasting focuses on the use of variations of recurrent neural networks, in particular, the Long-Short Term Memory (LSTM) cells. This research proposes a Particle Swarm Optimised-extremely randomised trees (PSO-ERT) regressor model for day ahead forecasting of the emissions intensity of the Australian National Electricity Market (NEM). The PSO-ERT model is compared with LSTM, another method known as Extreme Learning Machine (ELM), and also with two classic machine learning algorithms, Multi-layer perceptron (MLP) and Decision Tree (DT). Models are trained using 1.5 years of weather, demand and generation data collected from the five NEM regions. Results show that the ERT model performs better than both LSTM and ELM with Root Mean Squared Error (RMSE) of 42.9, 109.8, 165.7, 25.4 and 74.1 for New South Wales, Tasmania, South Australia, Queensland and Victoria, respectively. For all models, forecasting performance was better for regions with less renewable generation and for times when renewable generation was a smaller percentage of demand suggesting that the addition of weather forecasts in the predictor feature set would further improve performance.
更多查看译文
关键词
Emissions forecasting,National electricity market,Net-zero emission,Carbon footprint,Machine learning
AI 理解论文
溯源树
样例
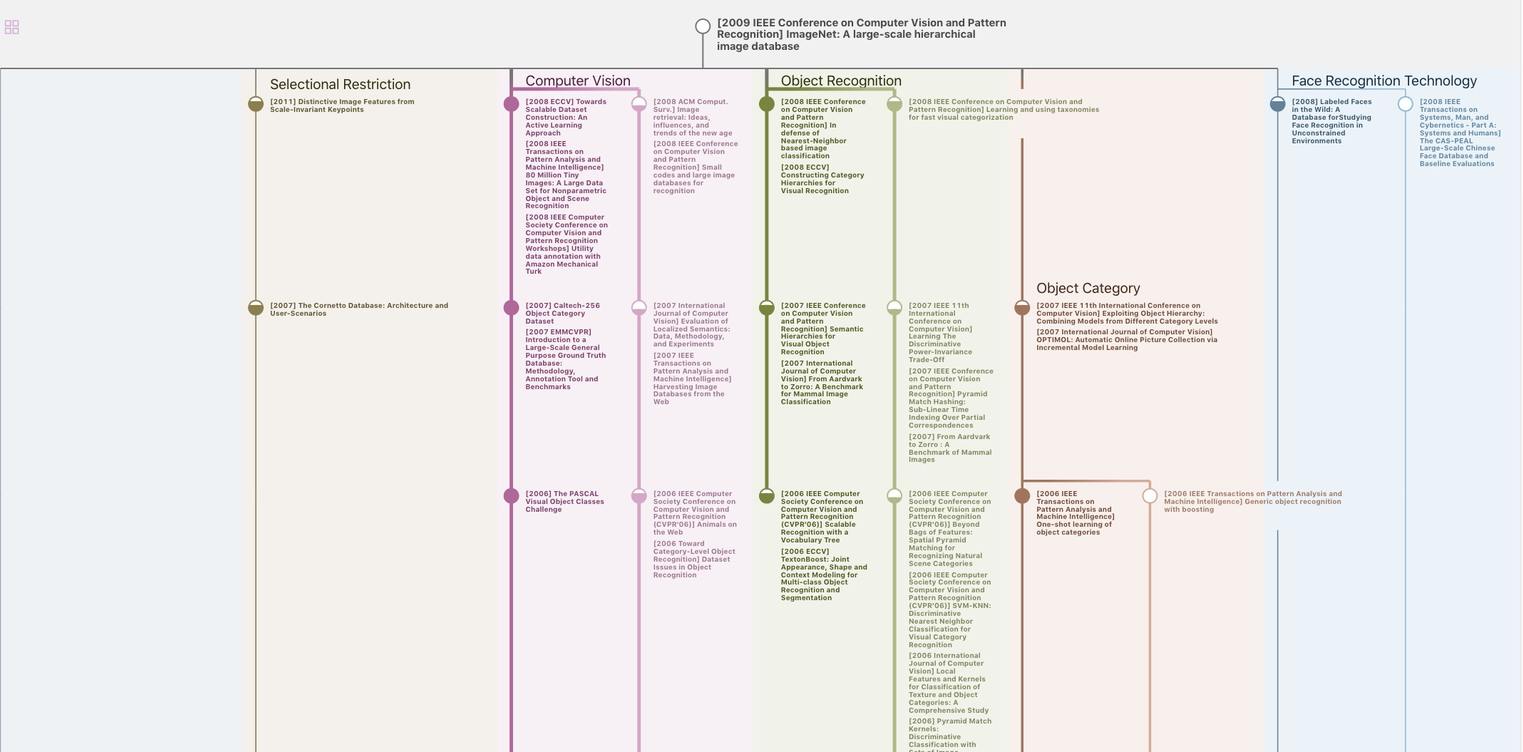
生成溯源树,研究论文发展脉络
Chat Paper
正在生成论文摘要