Personalized federated learning based on multi-head attention algorithm
INTERNATIONAL JOURNAL OF MACHINE LEARNING AND CYBERNETICS(2023)
摘要
Federated Learning (FL) is an algorithm for the encrypted exchange of model parameters while ensuring the independence of participants. Classic federated learning does not take into account the correlation between features, nor does it take into account the data differences caused by the reasonable personalization of each client. Therefore, this paper proposes a personalized federated learning algorithm based on a multi-head attention mechanism. First, in order to improve the personalization of local models, attention mechanism is used to capture the relevance of local features. Then, when aggregating local models, the weight λ is generated for local models based on the differences between models, and finally aggregate them into a new global model. Finally, the multi-head attention is proposed to calculate the importance score of the global model parameters on the current local model, and assign it to the local model as the attention coefficient, so as to realize personalized federated learning. Through experiments on MNIST, SVHN and STL10 datasets, the validity of Personalized Federated Learning is verified, and the rationality of hyperparameter setting is discussed through visualizing results.
更多查看译文
关键词
Federated learning,Multi-head attention mechanism,Personalize
AI 理解论文
溯源树
样例
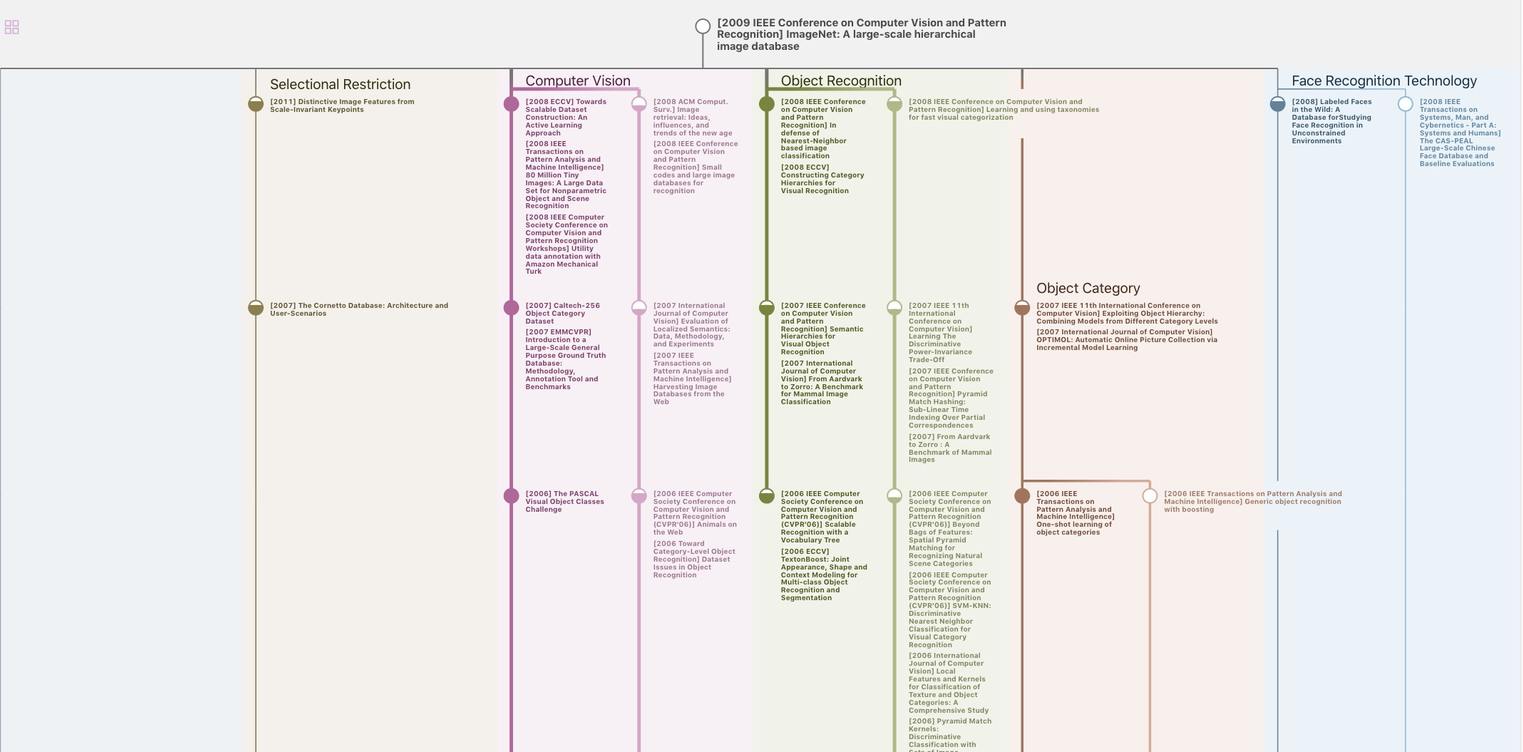
生成溯源树,研究论文发展脉络
Chat Paper
正在生成论文摘要