Deep learning methods improve polygenic risk analysis and prediction for Alzheimer’s disease
Research Square (Research Square)(2021)
摘要
Abstract Recent advances in genetic sequencing have enabled comprehensive genetic analyses of human diseases, resulting in the identification of numerous genetic risk factors for heritable disorders including Alzheimer’s disease (AD). Such analyses enable AD risk prediction well before disease onset, which is critical for early interventions. However, current analytical approaches have limited ability to accurately estimate the risk effects of genetic variants owing to epistatic effects, which have been overlooked in most previous studies, resulting in unsatisfactory disease risk prediction. Herein, we modeled AD polygenic risk using deep learning methods, which outperformed existing models (i.e., weighted polygenic risk score and lasso models) for classifying disease risk. Moreover, by examining the associations between the outcomes from deep learning methods and multi-omics data obtained from our in-house Chinese AD cohorts, we identified the pathways that are potentially regulated by AD polygenic risk, including immune-associated signaling pathways. Thus, our results demonstrate the utility of deep learning methods for modeling the genetic risks of human diseases, which can facilitate both disease risk classification and the study of disease mechanisms.
更多查看译文
关键词
alzheimers disease,deep learning methods,polygenic risk analysis
AI 理解论文
溯源树
样例
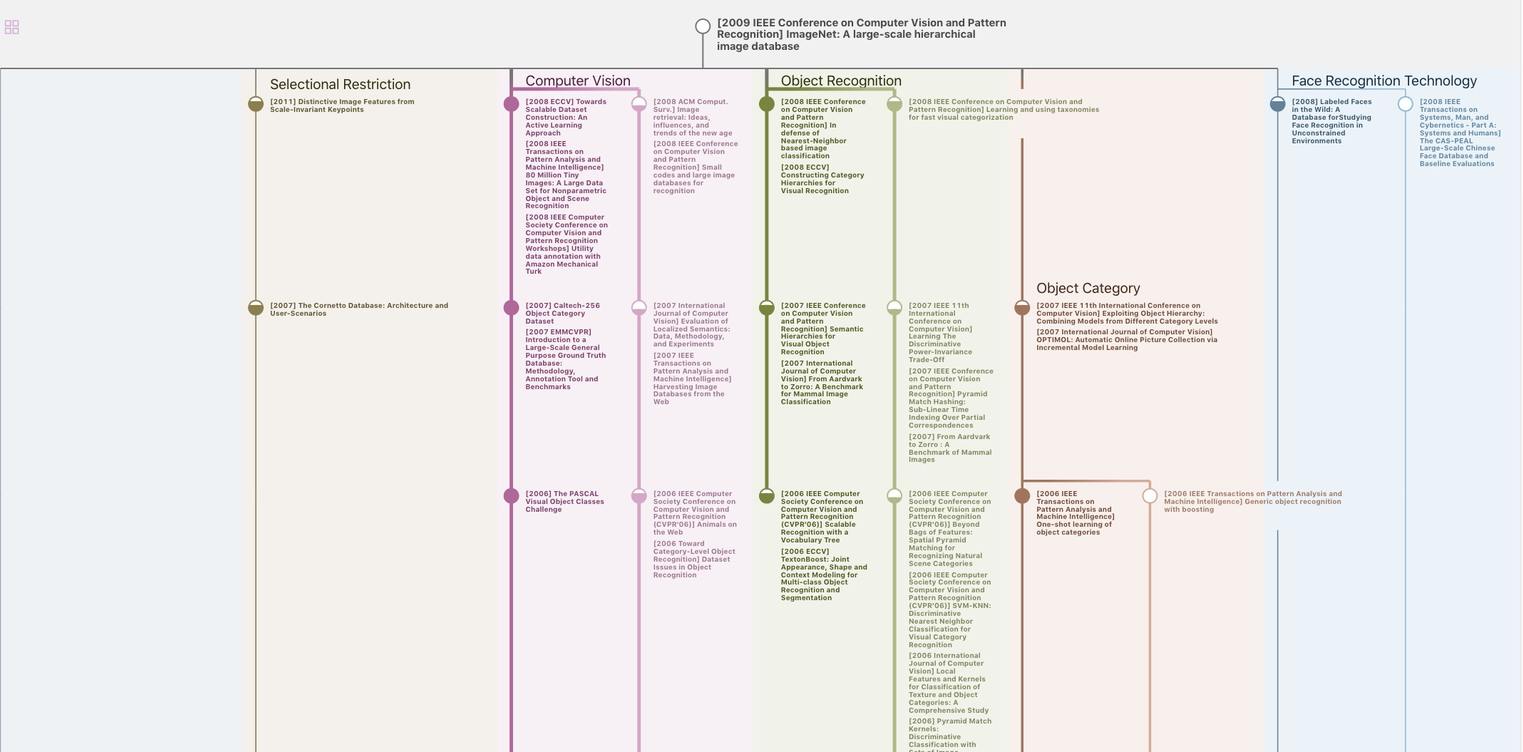
生成溯源树,研究论文发展脉络
Chat Paper
正在生成论文摘要