Using a decision tree algorithm to distinguish between repeated supra-therapeutic and acute acetaminophen exposures
BMC Medical Informatics Decis. Mak.(2023)
摘要
Background This study aimed to compare clinical and laboratory characteristics of supra-therapeutic (RSTI) and acute acetaminophen exposures using a predictive decision tree (DT) algorithm. Methods We conducted a retrospective cohort study using the National Poison Data System (NPDS). All patients with RSTI acetaminophen exposure (n = 4,522) between January 2012 and December 2017 were included. Additionally, 4,522 randomly selected acute acetaminophen ingestion cases were included. After that, the DT machine learning algorithm was applied to differentiate acute acetaminophen exposure from supratherapeutic exposures. Results The DT model had accuracy, precision, recall, and F1-scores of 0.75, respectively. Age was the most relevant variable in predicting the type of acetaminophen exposure, whether RSTI or acute. Serum aminotransferase concentrations, abdominal pain, drowsiness/lethargy, and nausea/vomiting were the other most important factors distinguishing between RST and acute acetaminophen exposure. Conclusion DT models can potentially aid in distinguishing between acute and RSTI of acetaminophen. Further validation is needed to assess the clinical utility of this model.
更多查看译文
关键词
Decision Tree,APAP,Repeated supra-therapeutic ingestion,Acute acetaminophen poisoning,NPDS
AI 理解论文
溯源树
样例
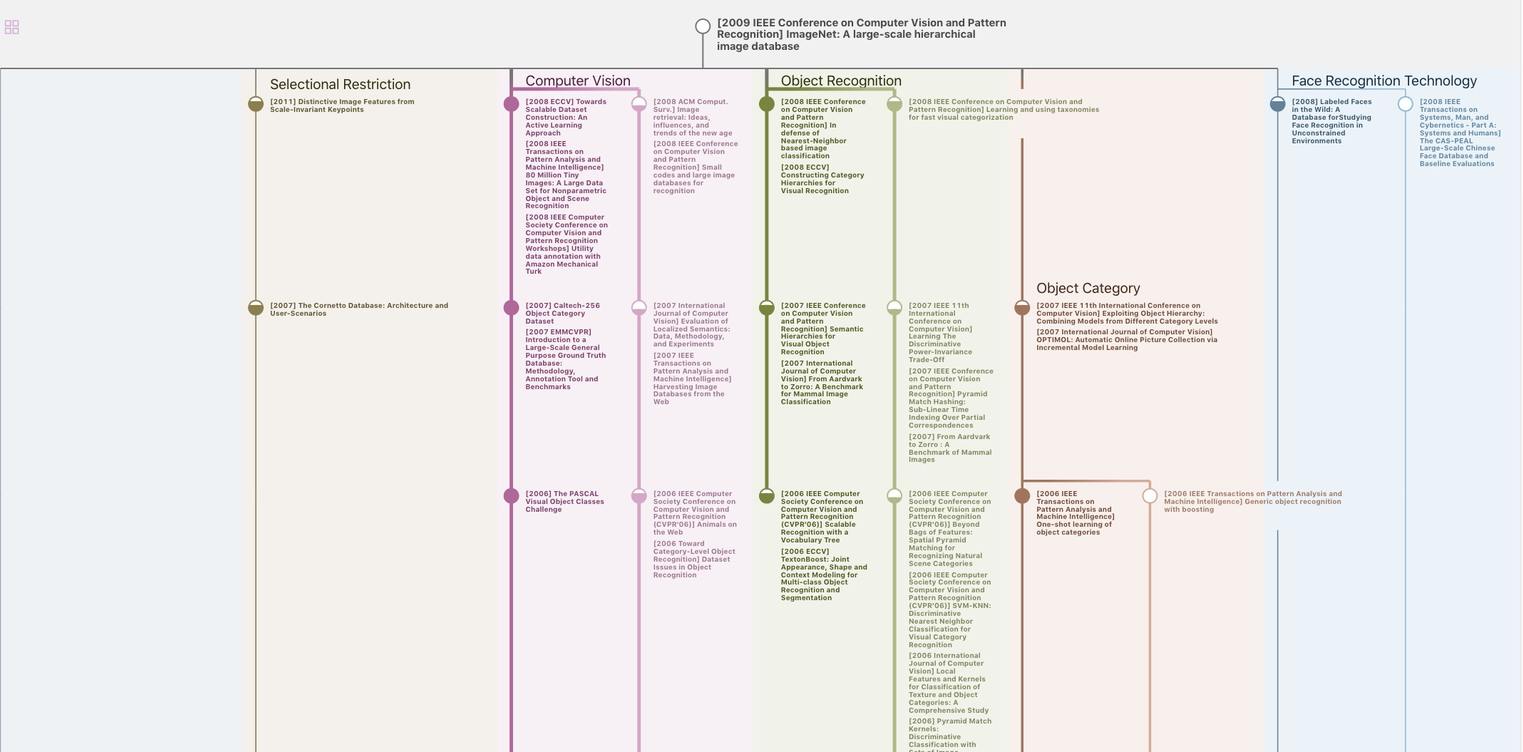
生成溯源树,研究论文发展脉络
Chat Paper
正在生成论文摘要