Pest Detection and Classification in Peanut Crops Using CNN, MFO, and EViTA Algorithms.
IEEE Access(2023)
摘要
The growth of vision transformer (ViT) methods have been quite enormous since its features provide efficient outcome in image classification, and identification. Inspired of this beneficial, this paper propose an Enhanced vision transformer architecture (EViTA) model for pest identification, segmentation, and classification. The as of late found that, compare to machine learning, Convolutional neural network algorithms the ViT has providing trusted results on image classification. Motivated by this, in this paper, we concentrate on the best way to learn dual barch segment representations in ViT models for image arrangement. Based upon its features, here propose a double layer transformer encoder to integrate pest image segments of various sizes of pest images to create more grounded image highlights. The current study uses, three pest datasets that affects peanut crops such as Aphids (IP102 Dataset), Wireworm (IP102 Dataset), and Gram Caterpillar collected from public available repository. Our methodology processes small segment and huge segment of tokens with two separate parts of various computational intricacy also, these tokens are then combined simply by consideration numerous times to complete one another. The taken datasets' are preprocessed utilizing the characteristic by using moth flame optimization (MFO), and flatten the images by using linear projector methodology to enhance the missing quality in pest images, and afterward normalization methods are executed to switch it over completely in to mathematical arrangement. This processed information is standardized further utilizing the self attention in StandardScaler procedures are carried out for choosing the ideal highlights in the dataset accordingly having huge effect towards affecting pest image predictions. These ideal highlights are at last taken care of into the EViTA model and the outcomes created are considered in contrast to the cutting edge models which at last legitimize the predominance of the proposed EViTA+PCA+MFO model in pest image prediction with high accuracy rate. Broad trials show that our methodology performs better compared to or on standard with a few simultaneous deals with vision transformer, notwithstanding productive CNN models.
更多查看译文
关键词
pest detection,peanut crops,classification
AI 理解论文
溯源树
样例
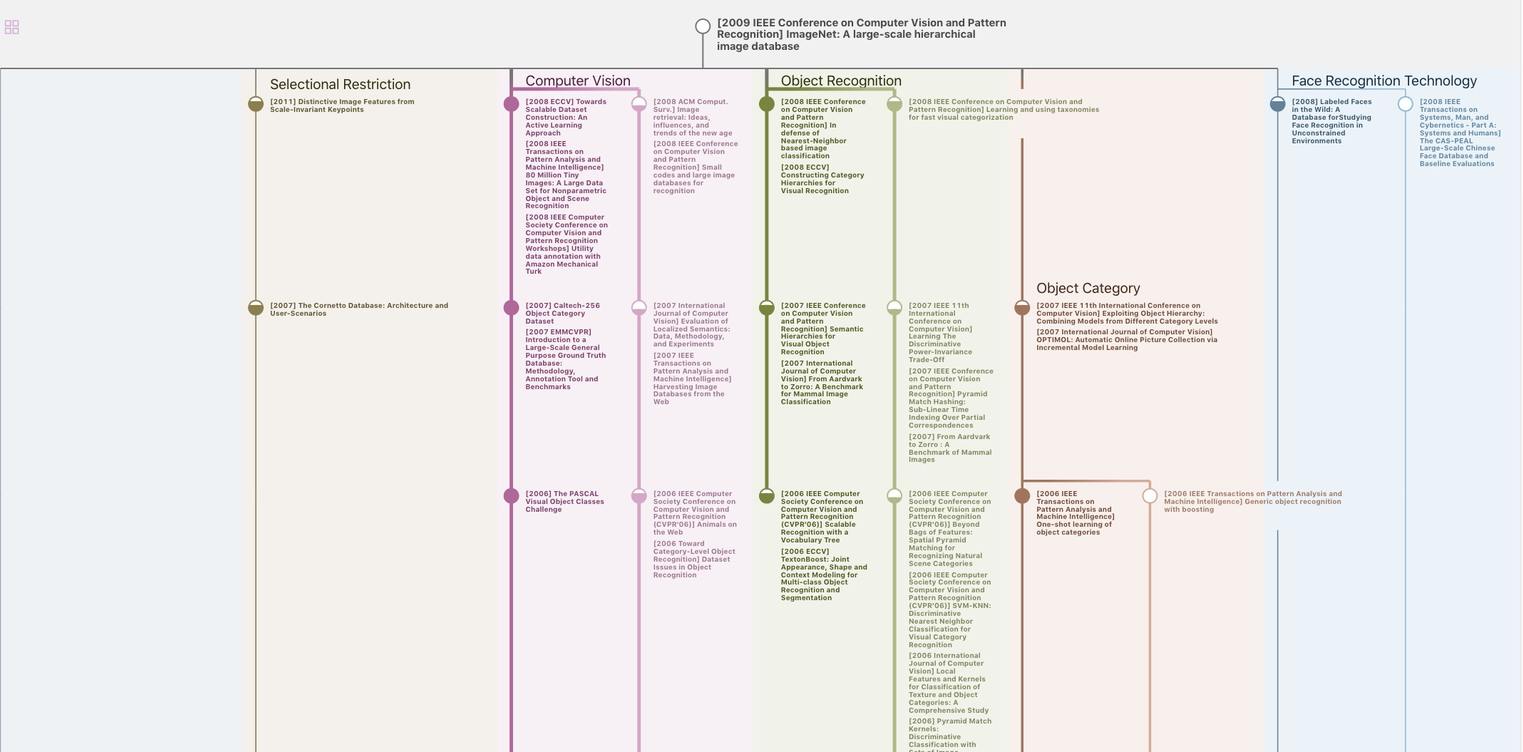
生成溯源树,研究论文发展脉络
Chat Paper
正在生成论文摘要