A Visualization Framework for Unsupervised Analysis of Latent Structures in SAR Image Time Series.
IEEE J. Sel. Top. Appl. Earth Obs. Remote. Sens.(2023)
摘要
Openly available satellite image time series (SITS) are considered an important resource for spatiotemporal change monitoring. However, obtaining semantically annotated datasets for such tasks is an expensive affair. To alleviate this problem, this article presents a novel framework to model and understand the image dynamics by discovering latent information in Sentinel-1 SITS, even with limited ground truth data. The framework suggests how to use visualizations to efficiently integrate domain knowledge both for execution and evaluation of the machine-learning pipeline in the absence from ground truth data in SITS change studies. In a case study at a Polar region, we extend a limited amount of ground truth data and then discover its temporal evolution at image patch level, in an unsupervised manner. The trustworthiness of the framework is ensured by integration of domain knowledge and intelligent visual verification strategies. A visualization tool is also implemented for this purpose. The proposed framework contains two modules: a classifier and a change modeler. Our experiments show that a domain-knowledge-based classifier gives the best accuracy. The classifier semantically labeled the complete dataset of 24 study months, containing 153 600 patches with a size of 256 x 256 pixels by extending the available semantic labels from just three months. The temporal sequence of these sematic labels are then recorded and fed to a Bayesian model called Latent Dirichlet Allocation (LDA) to discover the underlying patterns. LDA generates a change map containing the dominant dynamic patterns to give a consolidated view of the evolution without having to browse the whole dataset. Further, color-coded change signatures explain the change classes.
更多查看译文
关键词
sar image,unsupervised analysis,visualization framework,latent structures
AI 理解论文
溯源树
样例
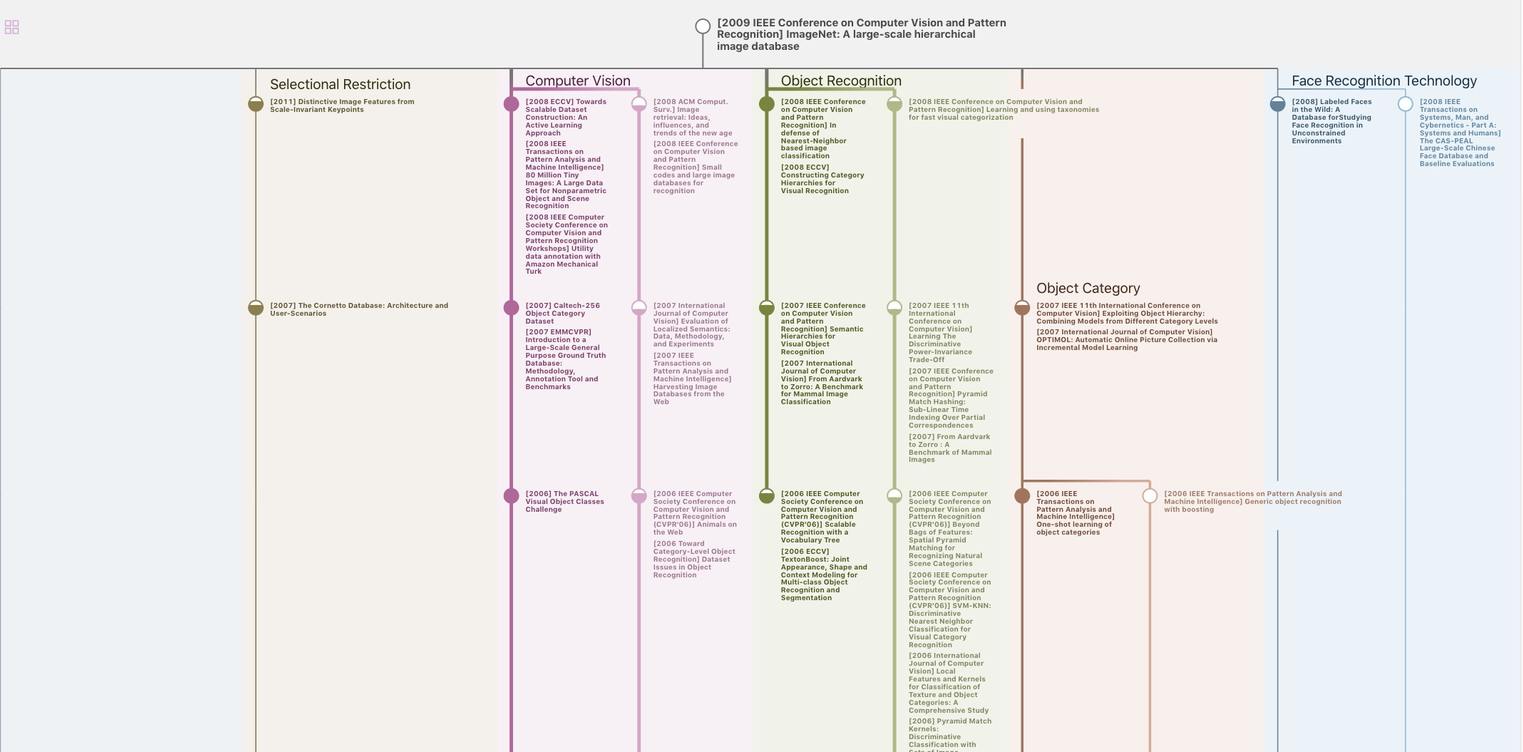
生成溯源树,研究论文发展脉络
Chat Paper
正在生成论文摘要