GGD-GAN: Gradient-Guided dual-Branch adversarial networks for relic sketch generation
Pattern Recognit.(2023)
摘要
Sketch reflects the main content and structure of the painted cultural relics, which help researcher understand the original drawing intention and painting skills. Although the existing automatic sketch extraction methods can improve efficiency, most of them are based on edge detection leading the limitations in incomplete details, serious disease noise and blurring. In this paper, a gradient guided dual-branch generative adversarial networks (GANs) is proposed for highquality relic sketch generation. The sketch generation branch (SGB) and auxiliary gradient-image generation branch (GGB) were designed via two independent GANs for learning different and complement characteristics. We also designed a feature transmission module for transferring context features from SGB to GGB, and a fusion block to realize the gradient guidance from GGB to SGB, forcing SGB to pay more attention to shape, details, and noise suppression. Both branches not only learn different characteristics independently, but also affect and complement each other, which generates rich and clean high-quality sketches. In our experiments, we compared our method with eight state-of-the-art algorithms quantitatively and qualitatively, analysis the effects of the gradient guidance feature transfer, and the generalization of the proposed dual-branch GAN framework. Experiments show the proposed framework is promising in its ability to extract a clear, coherent, and complete sketch of painted cultural relics. (c) 2023 Elsevier Ltd. All rights reserved.
更多查看译文
关键词
Relics digital protection,Sketch generation,Image translation,Deep learning
AI 理解论文
溯源树
样例
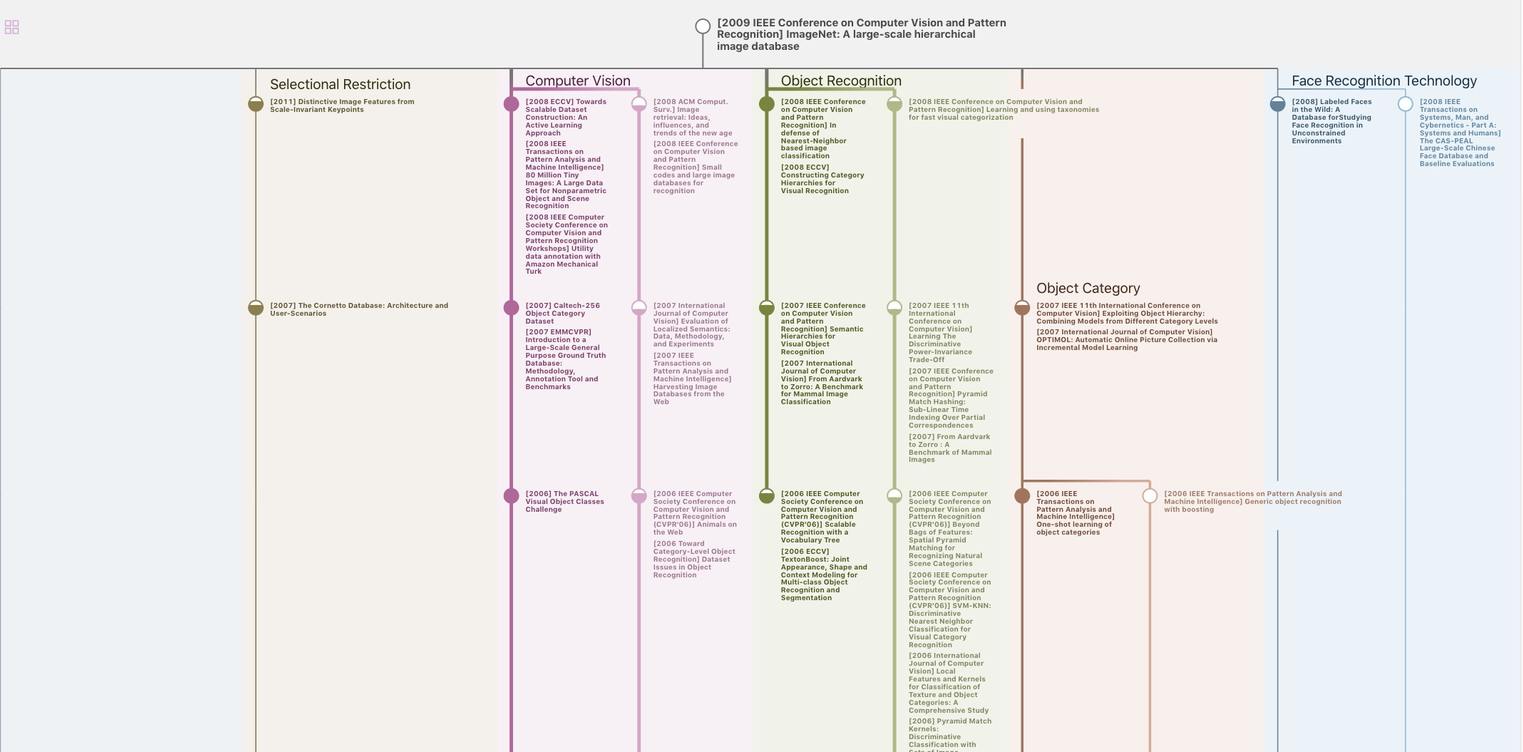
生成溯源树,研究论文发展脉络
Chat Paper
正在生成论文摘要