Multi-view unsupervised feature selection with tensor robust principal component analysis and consensus graph learning.
Pattern Recognit.(2023)
摘要
Recently, multi-view unsupervised feature selection has attracted much attention due to its efficiency and better interpretability in processing high-dimensional multi-view datasets. Most existing methods rely on the constructed similarity matrices to obtain reliable pseudo labels to guide the feature selec-tion. However, the considerable adverse noise in the raw data inevitably impedes the exploration of true underlying similarity structures. Besides, the inter-view correlations are often ignored during the com-mon representation learning, which limits the effective fusion of the essential information from multiple views. To solve these issues, we design a novel robust multi-view unsupervised feature selection frame-work. Specifically, our method seeks a set of noise-free view-specific similarity matrices by leveraging tensor robust principal component analysis, where the high-order connections among different views are well exploited through the constructed low-rank tensor. Meanwhile, a high-quality consensus similarity matrix is adaptively learned from the view-specific representations within the same unified framework to capture the shared local structures. To enhance the discriminative ability of the feature selection matrix, we further impose a rank constraint on the consensus similarity matrix to obtain reliable pseudo cluster indicators. We present an efficient optimization algorithm ground on the alternating direction method of multipliers to solve the proposed model. Experimental results on six multi-view datasets confirm the superiority of our method. (c) 2023 Elsevier Ltd. All rights reserved.
更多查看译文
关键词
robust principal component analysis,feature selection,tensor,multi-view
AI 理解论文
溯源树
样例
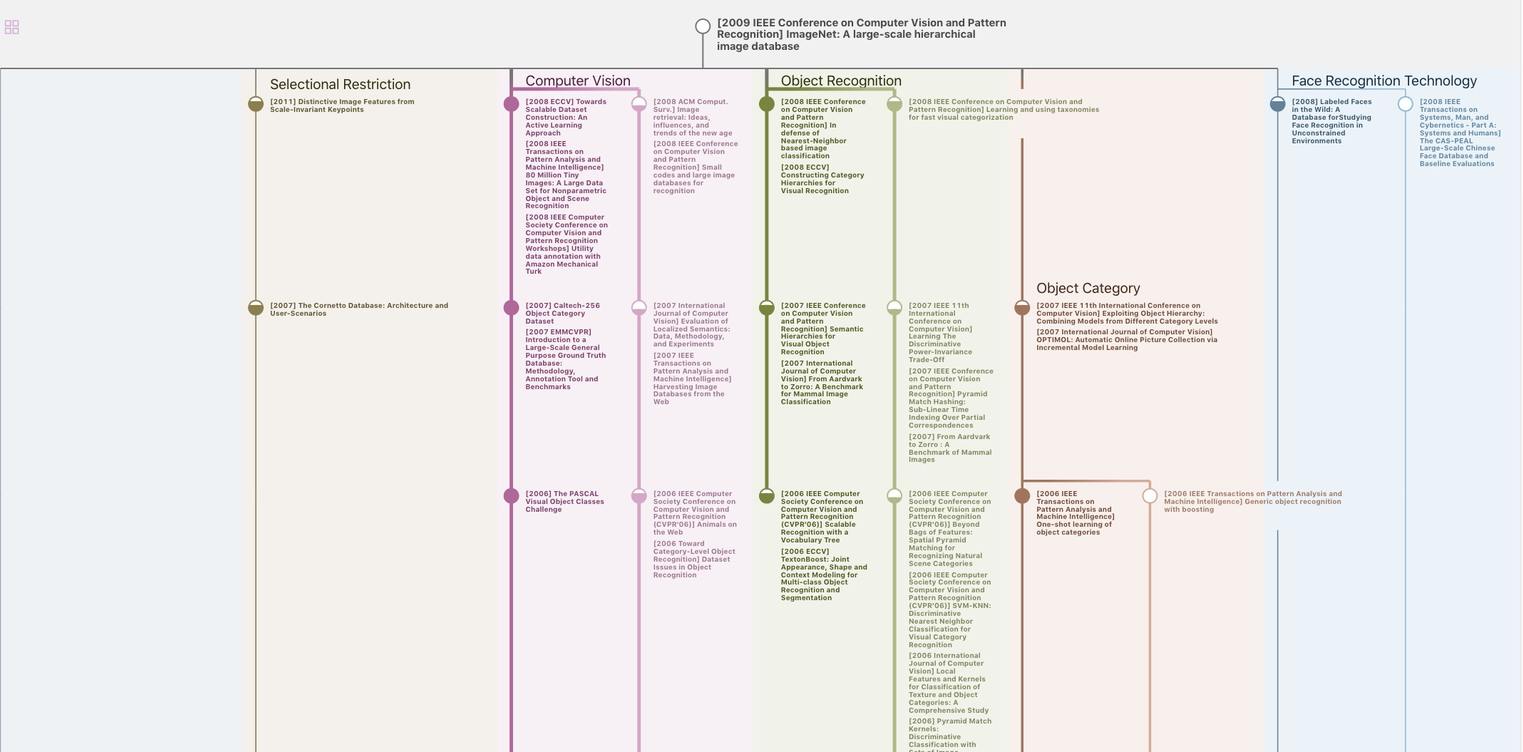
生成溯源树,研究论文发展脉络
Chat Paper
正在生成论文摘要