Sea surface height data reconstruction via inter and intra layer features based on dual attention.
Neurocomputing(2023)
摘要
Understanding of geoscience relies on spatio-temporal continuous fields, such as Sea Surface Height (SSH). Data reconstruction for restoring spatio-temporal continuous and gridded maps from incomplete observations and inaccuracy interpolation products has long been a crucial challenge in the marine geoscience. Despite remarkable progress, most existing learning-based reconstruction methods neglect to fully and discriminatively utilize the inter-layer and intra-layer features. The inter-layer semantics are complementary, and the significance of intra-layer components varies with frequency. To address these issues, we propose a multi-layer Feature Combination Network based on Attention mechanism (FCANet) for SSH data reconstruction. Specifically, a novel trainable Multi-layer Feature Combination Block (MFCB) is developed to enrich the features through inter-layer dependencies. Dual attention mechanism is introduced into the MFCB to enhance saliency of intra-layer features by adaptively rescaling the spatial-wise and channel-wise features. Furthermore, experimental results on SSH variable demonstrate the superiority of our FCANet over state-of-the-art reconstruction methods in the metrics of RMSE and SSIM.
更多查看译文
关键词
sea,intra layer features,dual attention
AI 理解论文
溯源树
样例
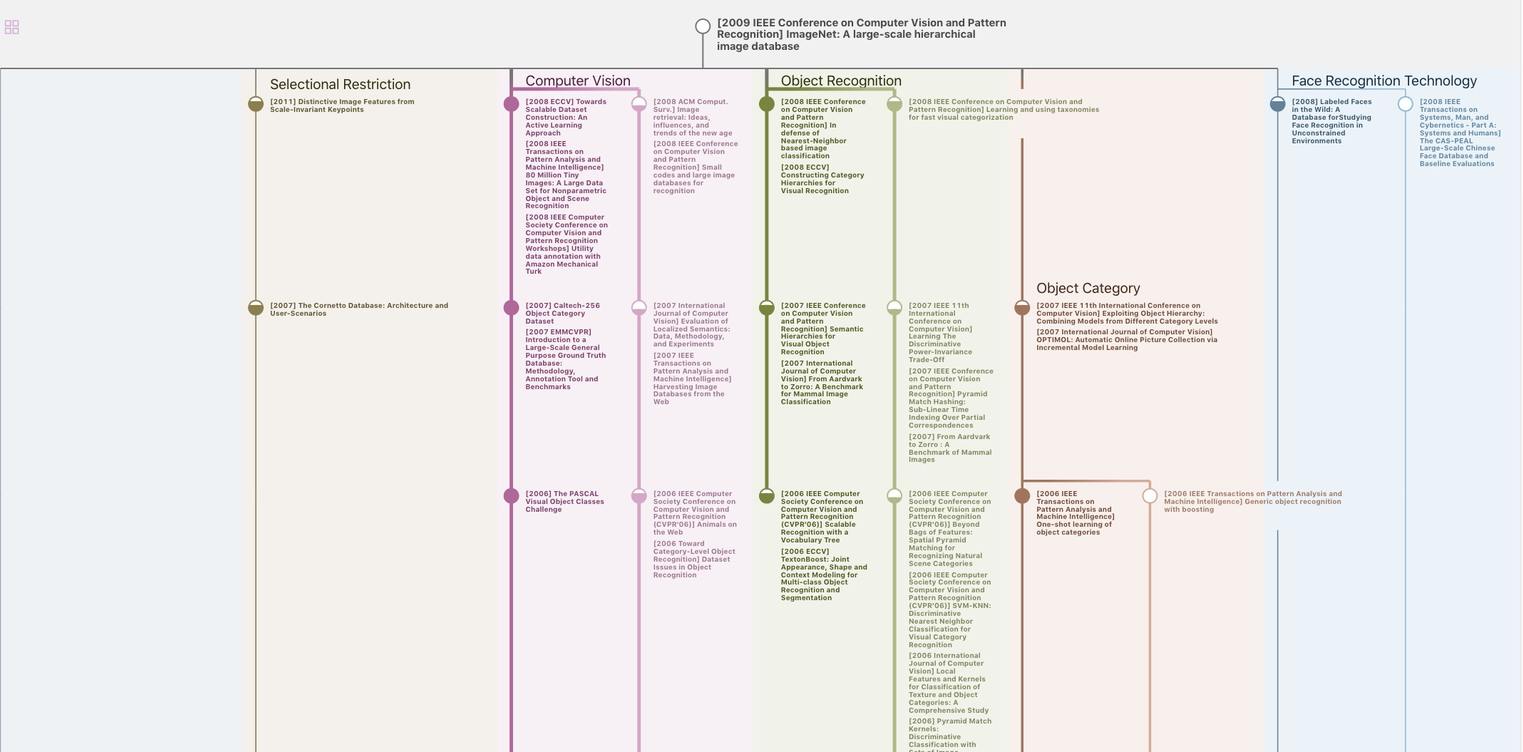
生成溯源树,研究论文发展脉络
Chat Paper
正在生成论文摘要