Applying and dissecting LSTM neural networks and regularized learning for dynamic inferential modeling.
Comput. Chem. Eng.(2023)
摘要
Deep learning models such as the long short-term memory (LSTM) network have been applied for dynamic inferential modeling. However, many studies apply LSTM as a black-box approach without examining the necessity and usefulness of the internal LSTM gates for inferential modeling. In this paper, we use LSTM as a state space realization and compare it with linear state space modeling and statistical learning methods, including N4SID, partial least squares, the Lasso, and support vector regression. Two case studies on an industrial 660 MW boiler and a debutanizer column process indicate that LSTM underperforms all other methods. LSTM is shown to be capable of outperforming linear methods for a simulated reactor process with severely excited nonlinearity in the data. By dissecting the sub-components of a simple LSTM model, the effectiveness of the LSTM gates and nonlinear activation functions is scrutinized.
更多查看译文
关键词
Dynamic inferential modeling,LSTM,Regularized learning,Partial least squares,Subspace identification
AI 理解论文
溯源树
样例
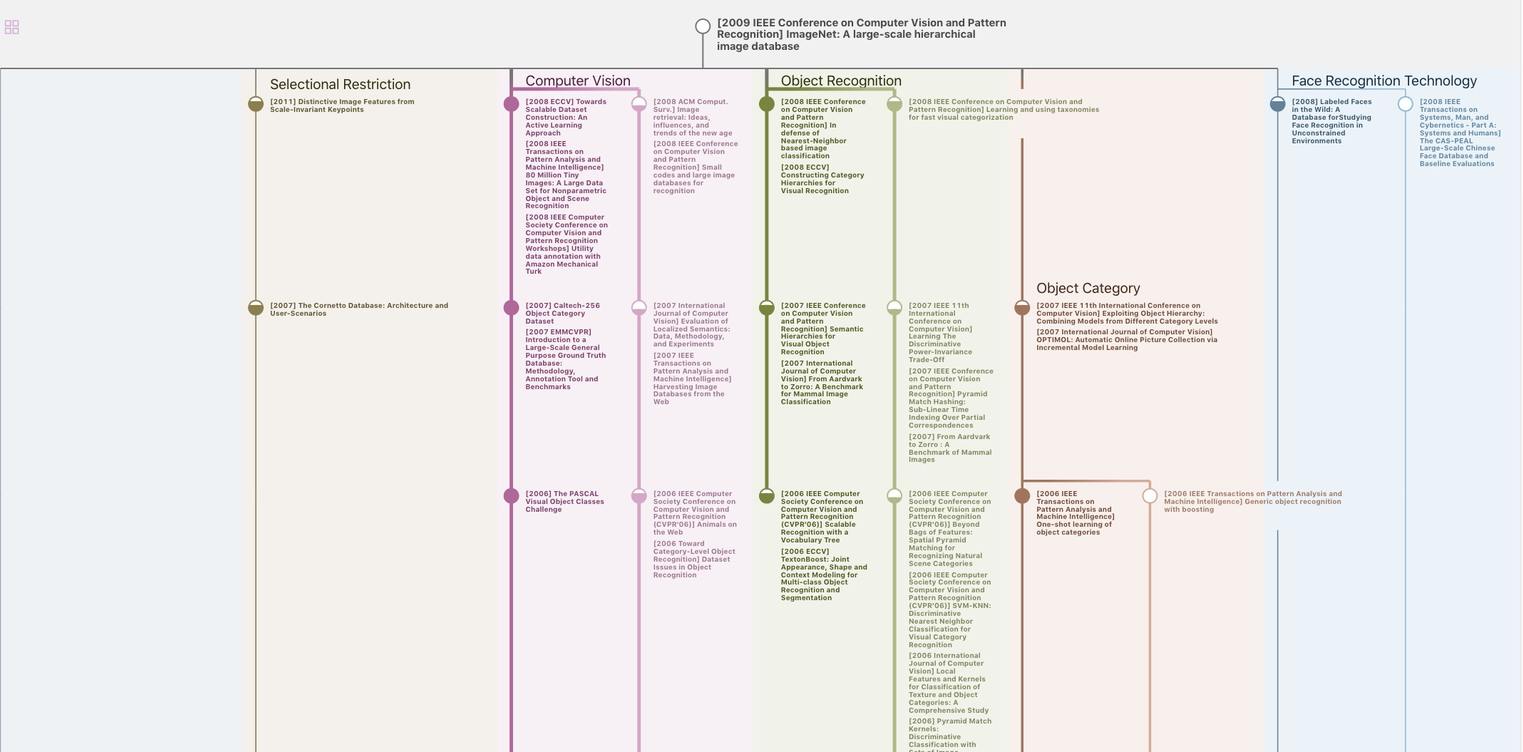
生成溯源树,研究论文发展脉络
Chat Paper
正在生成论文摘要