Recursive Quantile Estimation: Non-Asymptotic Confidence Bounds
JOURNAL OF MACHINE LEARNING RESEARCH(2023)
摘要
This paper considers the recursive estimation of quantiles using the stochastic gradient descent (SGD) algorithm with Polyak-Ruppert averaging. The algorithm offers a computationally and memory efficient alternative to the usual empirical estimator. Our focus is on studying the non-asymptotic behavior by providing exponentially decreasing tail probability bounds under mild assumptions on the smoothness of the density functions. This novel non-asymptotic result is based on a bound of the moment generating function of the SGD estimate. We apply our result to the problem of best arm identification in a multi-armed stochastic bandit setting under quantile preferences.
更多查看译文
关键词
Finite sample bounds,quantiles,stochastic gradient descent,Polyak-Ruppert averaging,recursive estimation
AI 理解论文
溯源树
样例
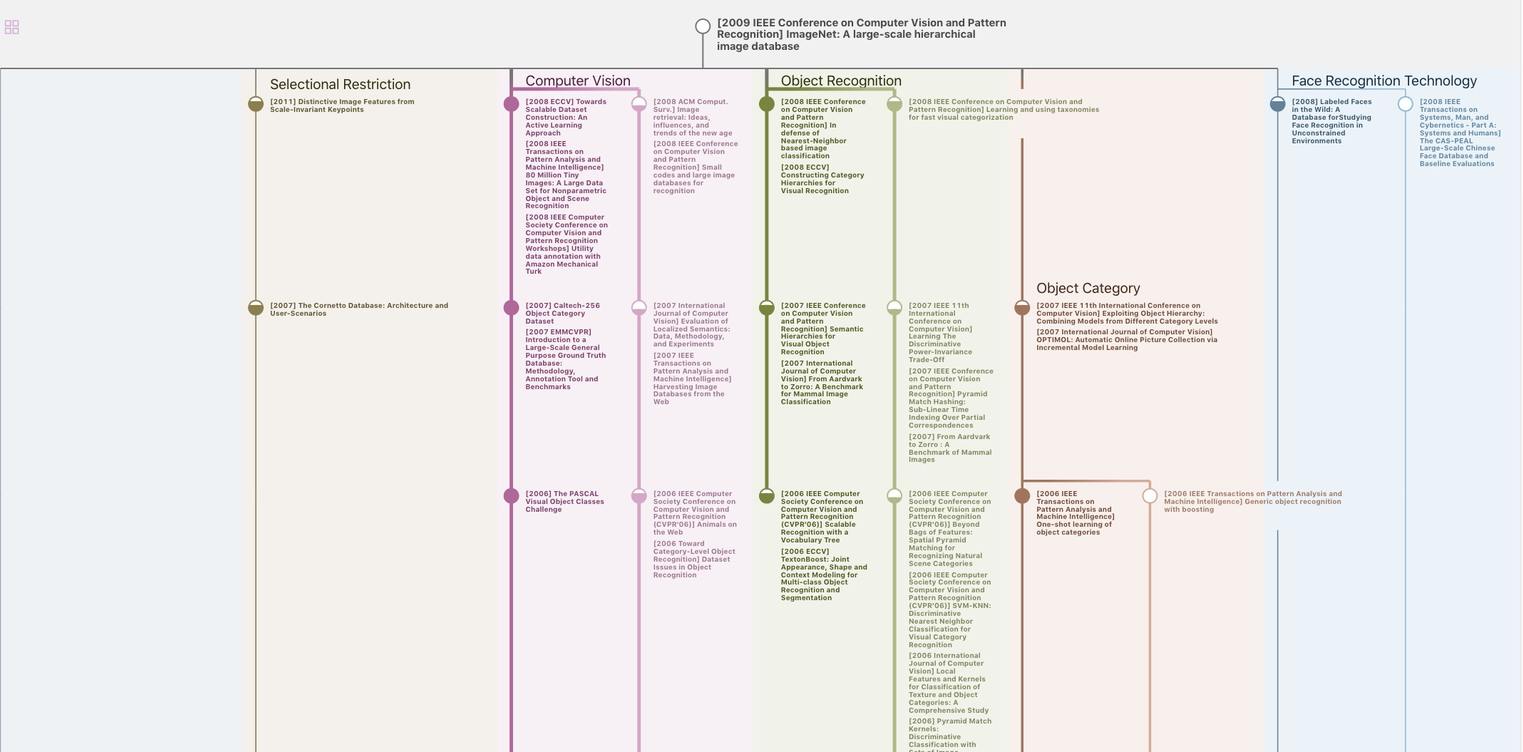
生成溯源树,研究论文发展脉络
Chat Paper
正在生成论文摘要