Consensus Big Data Clustering for Bayesian Mixture Models.
Algorithms(2023)
摘要
In the context of big-data analysis, the clustering technique holds significant importance for the effective categorization and organization of extensive datasets. However, pinpointing the ideal number of clusters and handling high-dimensional data can be challenging. To tackle these issues, several strategies have been suggested, such as a consensus clustering ensemble that yields more significant outcomes compared to individual models. Another valuable technique for cluster analysis is Bayesian mixture modelling, which is known for its adaptability in determining cluster numbers. Traditional inference methods such as Markov chain Monte Carlo may be computationally demanding and limit the exploration of the posterior distribution. In this work, we introduce an innovative approach that combines consensus clustering and Bayesian mixture models to improve big-data management and simplify the process of identifying the optimal number of clusters in diverse real-world scenarios. By addressing the aforementioned hurdles and boosting accuracy and efficiency, our method considerably enhances cluster analysis. This fusion of techniques offers a powerful tool for managing and examining large and intricate datasets, with possible applications across various industries.
更多查看译文
关键词
mixture,big data
AI 理解论文
溯源树
样例
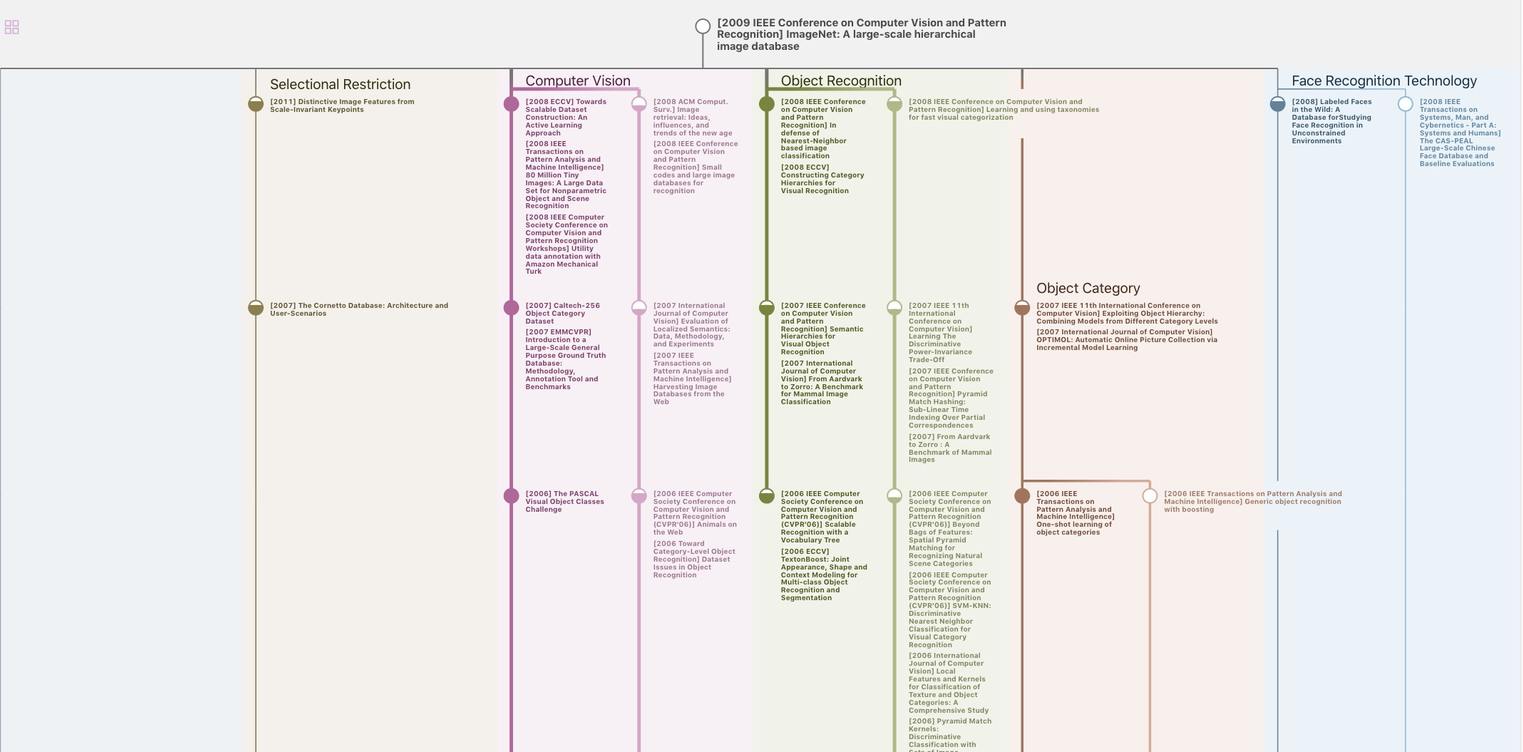
生成溯源树,研究论文发展脉络
Chat Paper
正在生成论文摘要