An efficient lightweight network for single image super-resolution*
J. Vis. Commun. Image Represent.(2023)
摘要
The outstanding performance of convolutional neural networks (CNNs) shown in single image super-resolution (SISR) strongly depends on network's depth, which hampers its application in low-power computing devices. In this paper, a lightweight and efficient network (LESR) is proposed for SISR by constructing the shallow feature extraction block (SFBlock), the cascaded sparse mask blocks (SMBlocks) and the feature fusion block (FFBlock). The SFBlock efficiently extracts global informative features from the original low resolution image using sparse self-attention, SMBlock skips the redundant computation in extracted features, and more meaningful information is distilled for the sequential reconstruction block by the FFBlock. In addition, a recently proposed activation function called ACON-C is used to replace the ReLU function to ease the training difficulty. Extensive experiments show that our proposed network performs better than most advanced lightweight SISR algorithms with comparable parameters and less FLOPs on benchmark database for x2/3/4 SISR.
更多查看译文
关键词
Super-resolution,Sparse,Efficiency,Lightweight,Self-attention
AI 理解论文
溯源树
样例
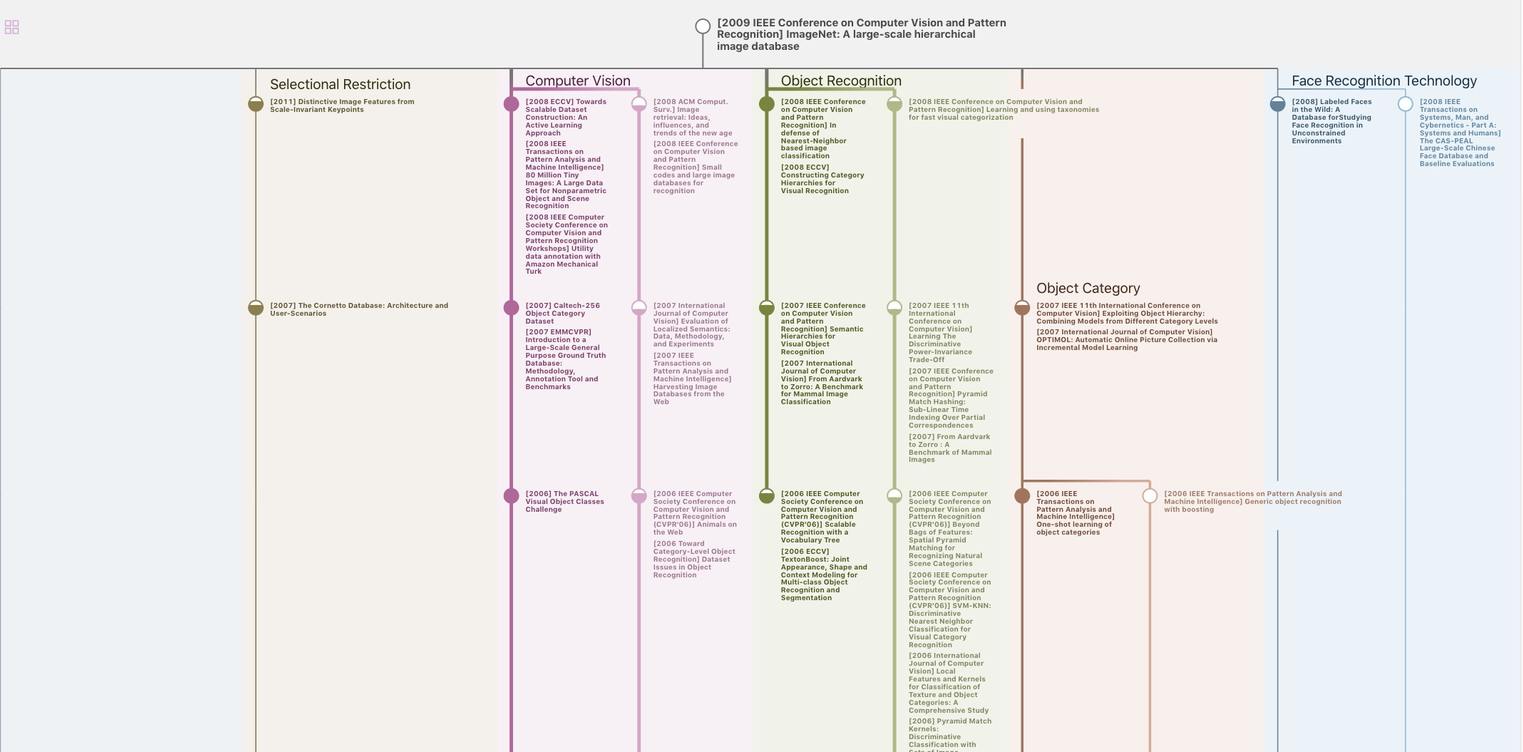
生成溯源树,研究论文发展脉络
Chat Paper
正在生成论文摘要