Hierarchical Residual Encoding for Multiresolution Time Series Compression.
Proc. ACM Manag. Data(2023)
摘要
Data compression is a key technique for reducing the cost of data transfer from storage to compute nodes. Increasingly, modern data scales necessitate lossy compression techniques, where exactness is sacrificed for a smaller compressed representation. One challenge in lossy compression is that different applications may have different accuracy demands. Today's compression techniques struggle in this setting either forcing the user to compress at the strictest accuracy demand, or to re-encode the data at multiple resolutions. This paper proposes a simple, but effective multiresolution compression algorithm for time series data, where a single encoding can effectively be decompressed at multiple output resolutions. There are a number of benefits over current state-of-the-art techniques for time series compression. (1) The storage footprint of this encoding is smaller than re-encoding the data at multiple resolutions. (2) Similarly, the compression latency is generally smaller than re-encoding at multiple resolutions. (3) Finally, the decompression latency of our encoding is significantly faster than single encodings at the strictest accuracy demand.
更多查看译文
关键词
hierarchical residual encoding,compression
AI 理解论文
溯源树
样例
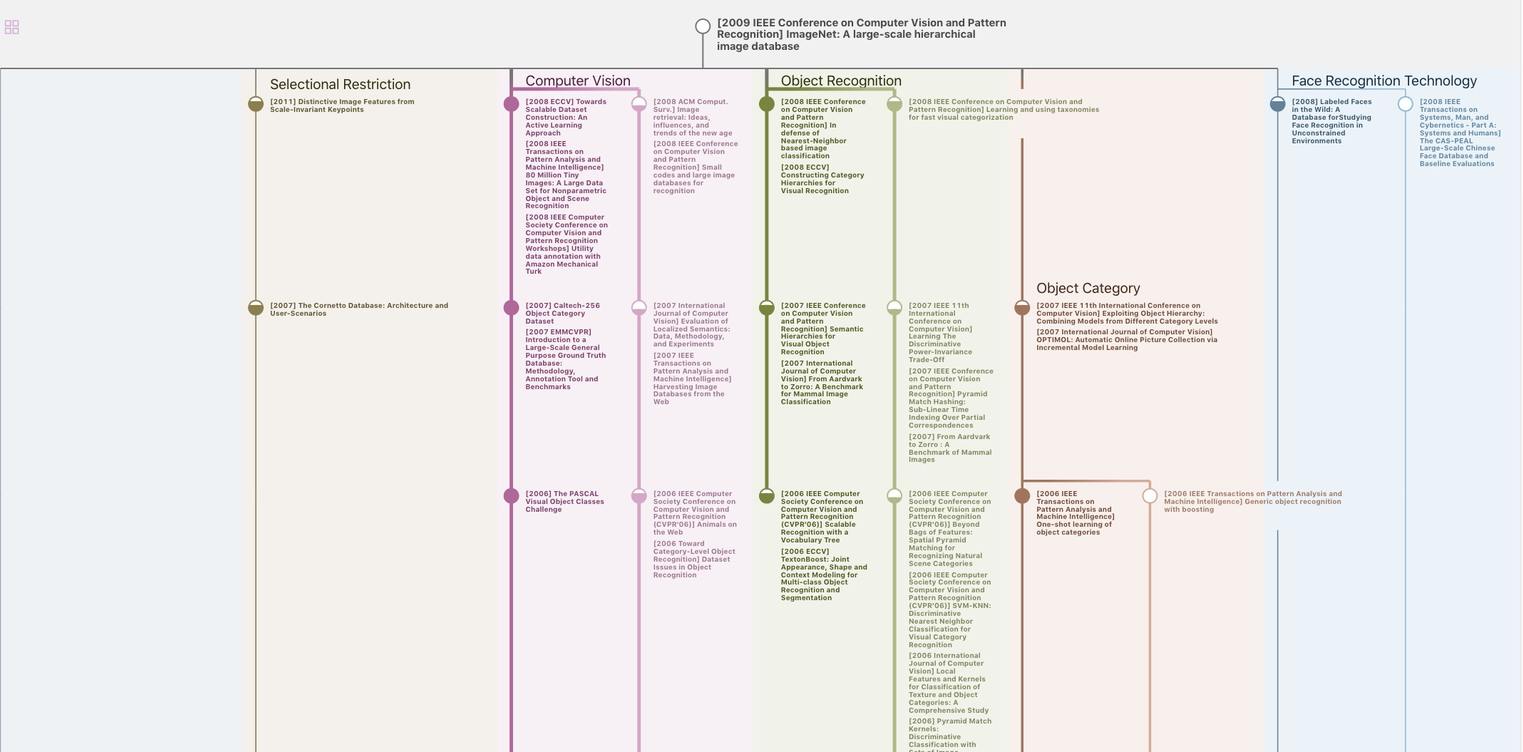
生成溯源树,研究论文发展脉络
Chat Paper
正在生成论文摘要