Growing Hierarchical Self-Organising Representation Map (GHSORM)
Inf. Sci.(2023)
摘要
The Growing Hierarchical Self-Organising Representation Map (GHSORM) is a model fusing the denoising autoencoder, used to better represent a dataset, and the Growing Hierarchical Self -Organising Map, used for organizing and projecting the input data in clusters of varying detail. It is shown here that the GHSORM is instrumental in sub-grouping clusters that are not fully separable by a single SOM. This combined approach is first tested and illustrated on the problem of clustering handwritten digits where a modification of the Activation Maximisation method for use at the SOM output layer demonstrates the benefit of hierarchical growth in the GHSORM. In particular, the SOM Node Activation Maximisation method is used to visually represent the best approximation of each of the SOM nodes at the output layer. This demonstrates the improvement in representing difficult to separate digits in the hierarchical case. To test and measure the effi-cacy of the GHSORM hierarchical model in class and sub-class separation the method is applied to complex digital gene expression data. A cancer dataset, comprising of gene expression data that has samples of different classes and sub-classes, is used for this purpose. The GHSORM demon-strates robust capabilities to cluster and sub-cluster the different classes and subclasses of cancer, where the results are superior to both linear methods, currently in use, as well as the methods of its constituent algorithms.
更多查看译文
关键词
Unsupervised learning,Hierarchical,Self -organizing map,Denoising autoencoder,Cancer clustering,Cancer sub -typing
AI 理解论文
溯源树
样例
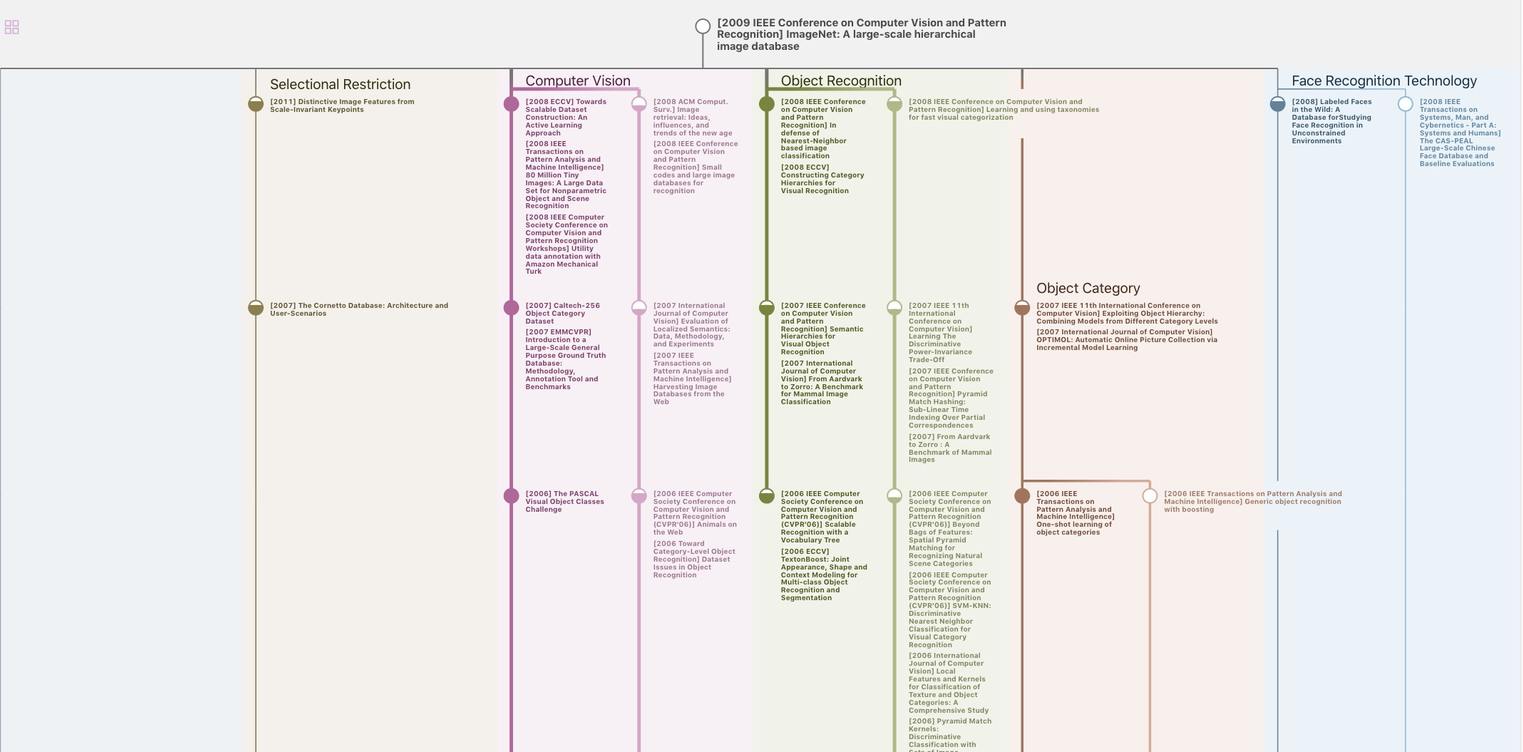
生成溯源树,研究论文发展脉络
Chat Paper
正在生成论文摘要