Self-paced multi-view positive and unlabeled graph learning with auxiliary information.
Inf. Sci.(2023)
摘要
Graph widely exists in the research study, with complex structure and rich information, and it has always been a powerful mode to analyze difficult problems. Meanwhile, graph classification is an important application problem in many fields, it means that we use the structure of known graph datasets to classify the unknown graph. Most of the current work is to classify through positive and unlabeled learning (PU learning) and multi-view learning respectively. For training and testing data, it is believed that they all have the same feature representation, but in fact, we can gain auxiliary features called privileged information for the training data. In the past, privileged information is always ignored in PU graph learning. To enhance the efficiency of the classifier, a new method called SMPUP is presented, which first extracts the features of graph from different perspectives, and introduces privileged information into the PU learning method to construct the classifier model. Further, we have introduced a self-paced learning (SPL) framework to ensure the model can learn efficiently. A large number of experiments with graph and biological datasets show that the SMPUP method performs better than previous methods.
更多查看译文
关键词
Graph classification,Multi-view learning,Privileged information,Self-paced learning,PU learning
AI 理解论文
溯源树
样例
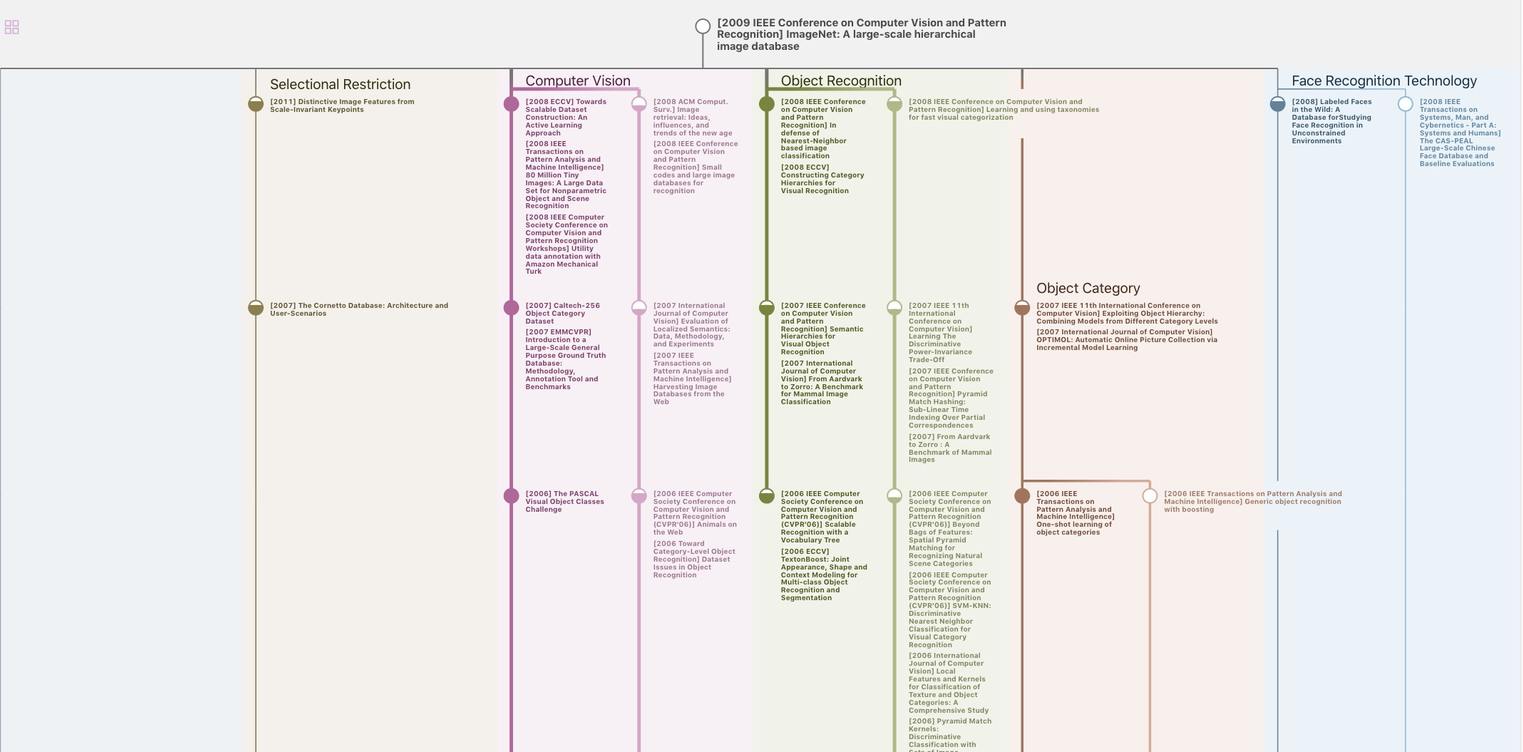
生成溯源树,研究论文发展脉络
Chat Paper
正在生成论文摘要