Differentially Private Streaming Data Release under Temporal Correlations via Post-processing.
CoRR(2023)
摘要
The release of differentially private streaming data has been extensively studied, yet striking a good balance between privacy and utility on temporally correlated data in the stream remains an open problem. Existing works focus on enhancing privacy when applying differential privacy to correlated data, highlighting that differential privacy may suffer from additional privacy leakage under correlations; consequently, a small privacy budget has to be used which worsens the utility. In this work, we propose a post-processing framework to improve the utility of differential privacy data release under temporal correlations. We model the problem as a maximum posterior estimation given the released differentially private data and correlation model and transform it into nonlinear constrained programming. Our experiments on synthetic datasets show that the proposed approach significantly improves the utility and accuracy of differentially private data by nearly a hundred times in terms of mean square error when a strict privacy budget is given.
更多查看译文
关键词
private streaming data release,temporal correlations,post-processing
AI 理解论文
溯源树
样例
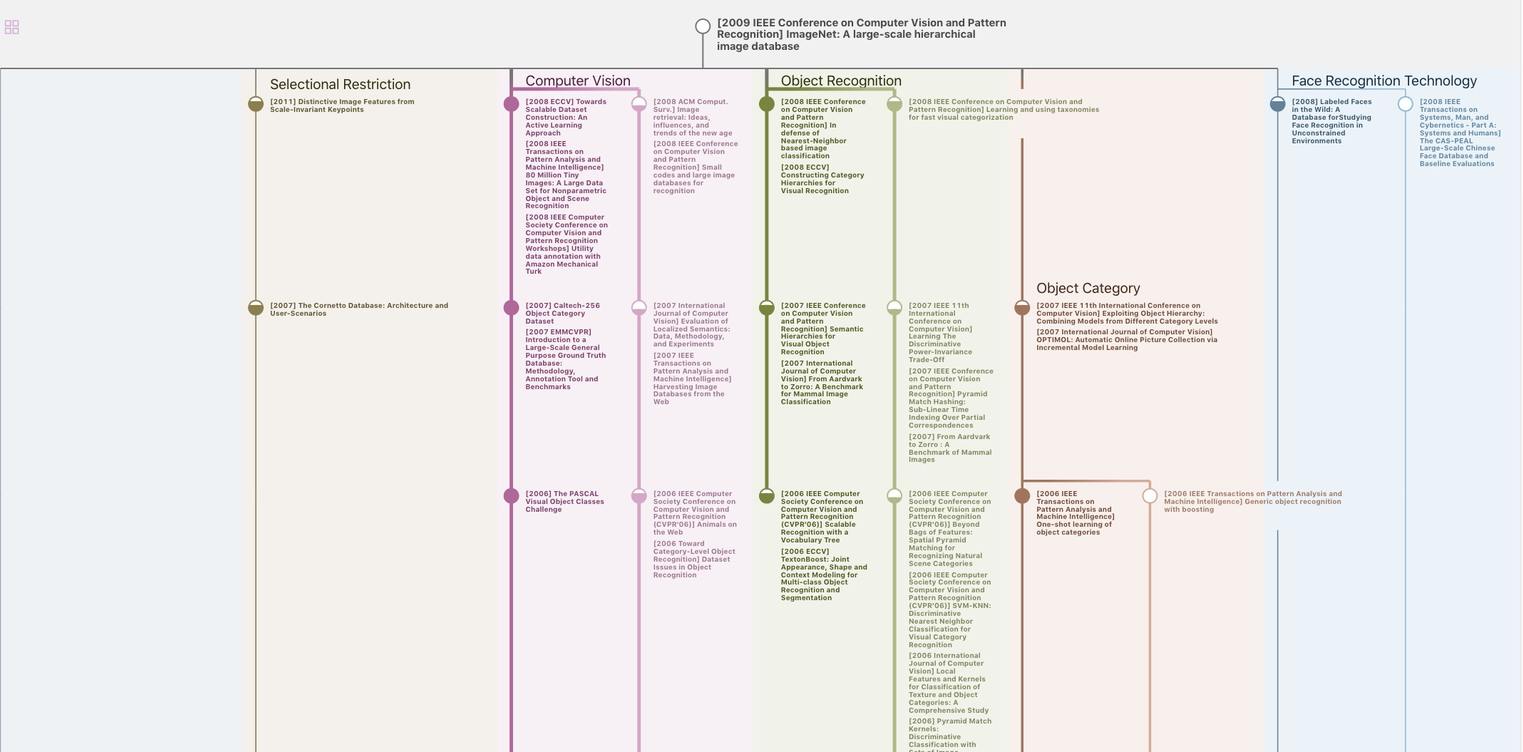
生成溯源树,研究论文发展脉络
Chat Paper
正在生成论文摘要