Multilevel Feature Aggregated Network with Instance Contrastive Learning Constraint for Building Extraction.
Remote. Sens.(2023)
摘要
Building footprint extraction from remotely sensed imagery is a critical task in the field of illegal building discovery, urban dynamic monitoring, and disaster emergency response. Recent research has made significant progress in this area by utilizing deep learning techniques. However, it remains difficult to efficiently balance the spatial detail and rich semantic features. In particular, the extracted building edge is often inaccurate, especially in areas where the buildings are densely distributed, and the boundary of adjacent building instances is difficult to distinguish accurately. Additionally, identifying buildings with varying scales remains a challenging problem. To address the above problems, we designed a novel framework that aggregated multilevel contextual information extracted from multiple encoders. Furthermore, we introduced an instance constraint into contrastive learning to enhance the robustness of the feature representation. Experimental results demonstrated that our proposed method achieved 91.07% and 74.58% on the intersection over union metric on the WHU and Massachusetts datasets, respectively, outperforming the most recent related methods. Notably, our method significantly improved the accuracy of building boundaries, especially at the building instance level, and the integrity of multi-scale buildings.
更多查看译文
关键词
instance contrastive learning constraint,building,extraction
AI 理解论文
溯源树
样例
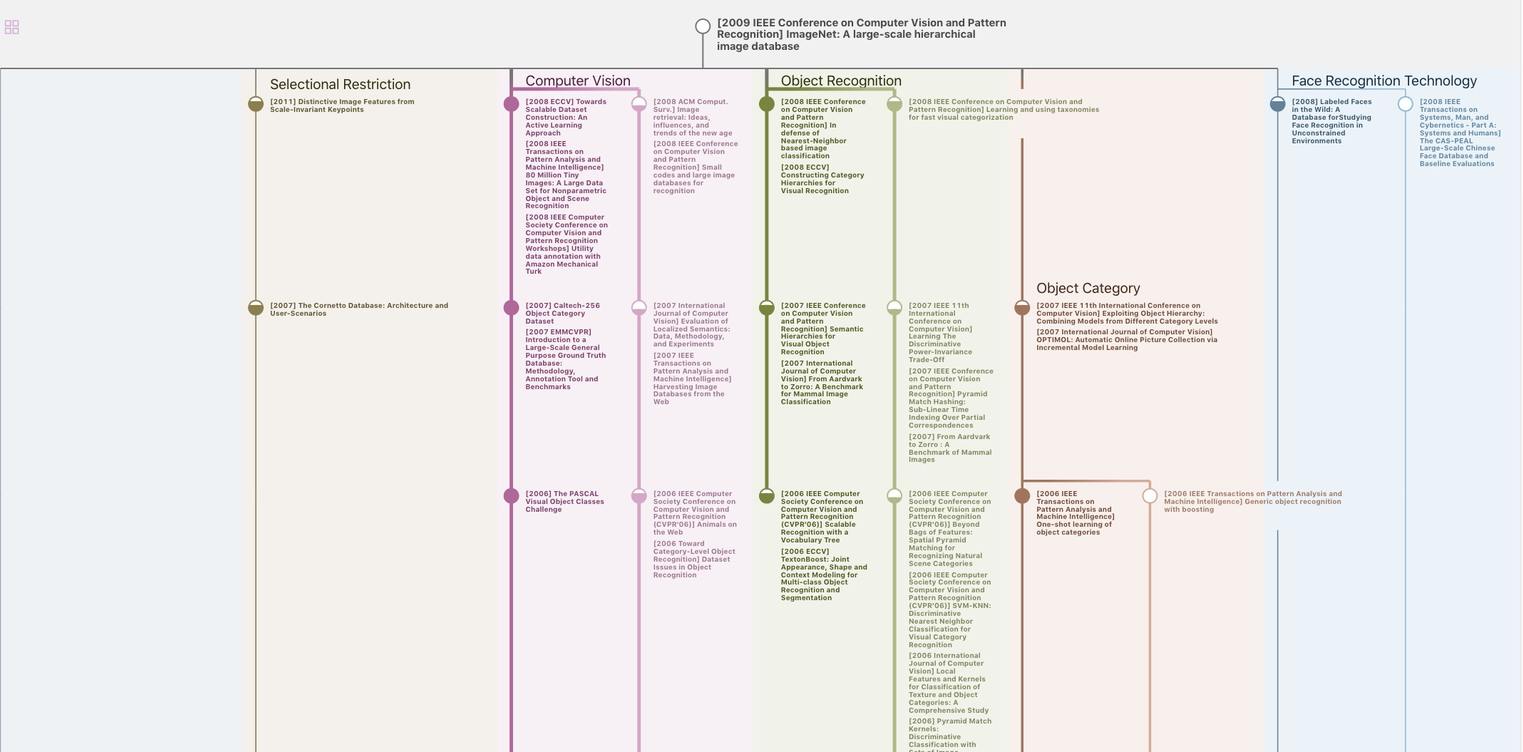
生成溯源树,研究论文发展脉络
Chat Paper
正在生成论文摘要