DED: Diagnostic Evidence Distillation for acne severity grading on face images.
Expert Syst. Appl.(2023)
摘要
Acne seriously affects people’s daily life. Acne severity level grading plays a decisive role in the cure. However, the acne criterion is not unified in the medical field. Most of the current studies explore the application of advanced visual models on acne severity grading but lack the adaptation to the characteristics of acne diagnosis. Meanwhile, some studies propose specifically adapted methods for respectively used acne criteria but cannot be used on other acne criteria. In this study, we propose an acne diagnosis method, Diagnostic Evidence Distillation (DED), that suitably adapts the characteristics of acne diagnosis and can be applied to diagnose under different acne criteria. Firstly, we fully investigate and analyze the commonality of various acne criteria and condense the face acne diagnosis into an unconventional image classification problem based on the diagnostic evidence of type and number of fine-grained lesions distributed on a whole face. Next, we propose the DED framework to adapt the characteristics of acne diagnosis. This framework uses the teacher–student structure of knowledge distillation methods to bring the diagnostic evidence, which is not available for new patients but only in training data, into the diagnosis model. To break the limitation of different criteria, the framework uses convolutional neural networks (CNNs) as backbones to imitate the global estimation of dermatology. We also propose a subtask joint learning for the teacher network to enhance its guidance to the student. The DED is applied to diagnose acne on two datasets ACNE04 and PLSBRACNE01 based on different mainstream acne criteria. The experimental results demonstrate that on both datasets, the DED effectively improves the diagnosis performance, exceeds the state-of-the-art and reaches the diagnostic level of dermatologists. The precision, sensitivity, specificity, Youden Index and accuracy reach 85.31%, 84.83%, 94.66%, 79.48% and 86.06% on the ACNE04 dataset and 69.16%, 65.62%, 88.93%, 54.54% and 67.56% on the PLSBRACNE01 dataset, respectively.
更多查看译文
关键词
acne severity,diagnostic evidence distillation,face,ded
AI 理解论文
溯源树
样例
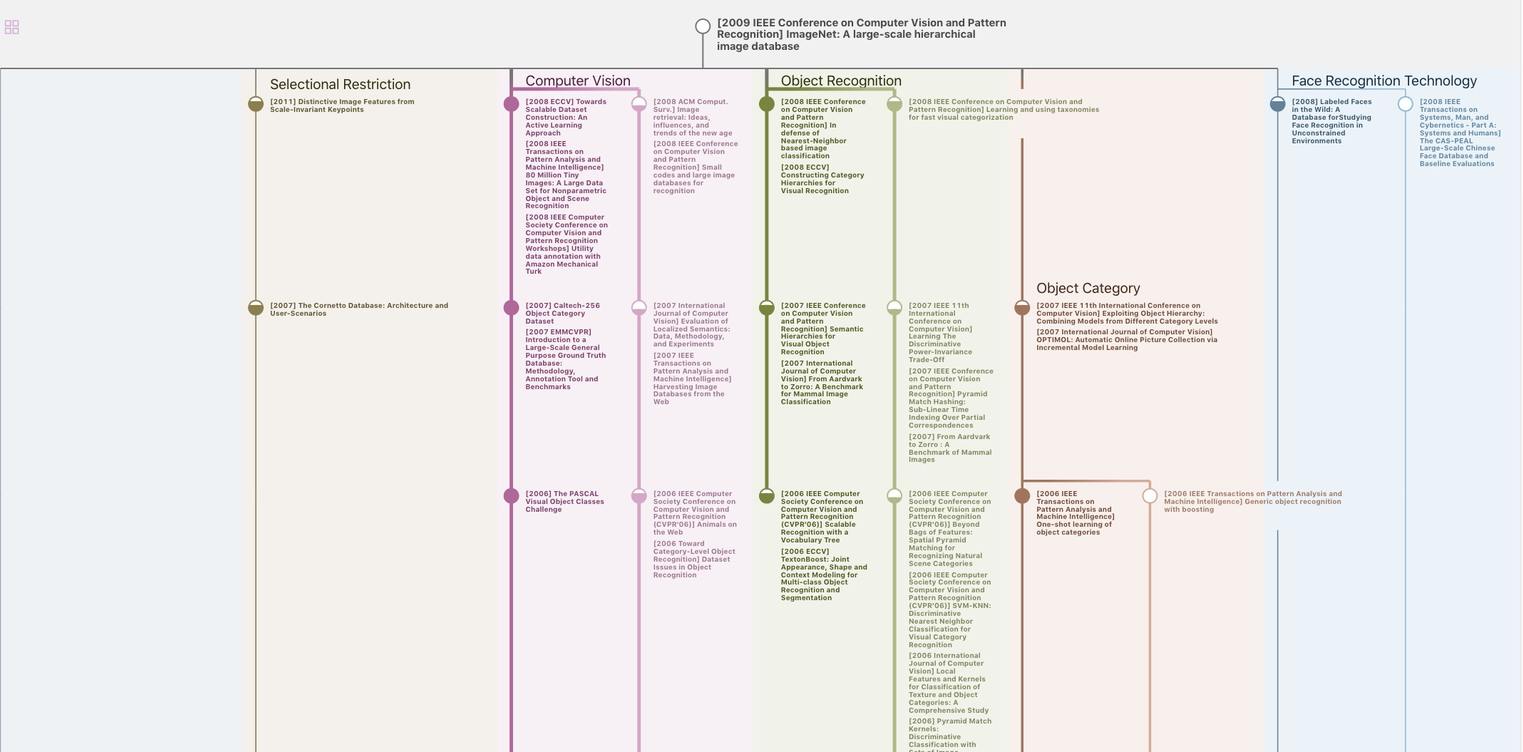
生成溯源树,研究论文发展脉络
Chat Paper
正在生成论文摘要