CerCan·Net: Cervical cancer classification model via multi-layer feature ensembles of lightweight CNNs and transfer learning.
Expert Syst. Appl.(2023)
摘要
Cervical cancer ranks among the most prevalent causes of fatality in women around the world. Early diagnosis is essential for treating cervical cancer using pap smear slides, but it is complex and time-consuming. Computer-aided diagnostic (CAD) systems based on deep learning models, particularly convolutional neural networks (CNNs), can facilitate the accurate and rapid diagnosis of cervical cancer. Recent CAD structures relied on using attributes extracted from a single layer of an individual CNN. However, distinct layers of CNNs are capable of specifying various-level knowledge, so merging deep features from various layers of a CNN could boost performance. Furthermore, incorporating deep features from multiple CNNs with various configurations could improve performance. This study proposes an efficient CAD entitled “CerCan·Net” for the automatic diagnosis of cervical cancer. CerCan·Net employs three lightweight CNNs with fewer parameters and lesser deep layers than that used in the literature, such as MobileNet, DarkNet-19, and ResNet-18, to reduce the complexity of classification. CerCan·Net engages transfer learning to obtain deep features out of the last three deep layers of each CNN, rather than using deep features of a specific layer. It also combines the features acquired by utilising the multi-CNN layers. Then, by maintaining a feature selection approach, it investigates the impact of generating a smaller set of deep features that could differentiate distinct subgroups of cervical cancer. CerCanNet performance is validated using two benchmark datasets, namely, the SIPaKMeD dataset and the Mendeley dataset. The results show that CerCan·Net is capable of achieving a high accuracy of 97.7% and 100% for the SIPaKMeD and Mendeley datasets using 400 and 200 features. Moreover, when comparing the performance of CerCan·Net with recent CADs, the comparison demonstrated its superiority. Therefore, it can be employed to assist cytopathologists in the automatic inspection, thereby avoiding limitations of routine diagnosis.
更多查看译文
关键词
cervical cancer classification model,lightweight cnns,feature,multi-layer
AI 理解论文
溯源树
样例
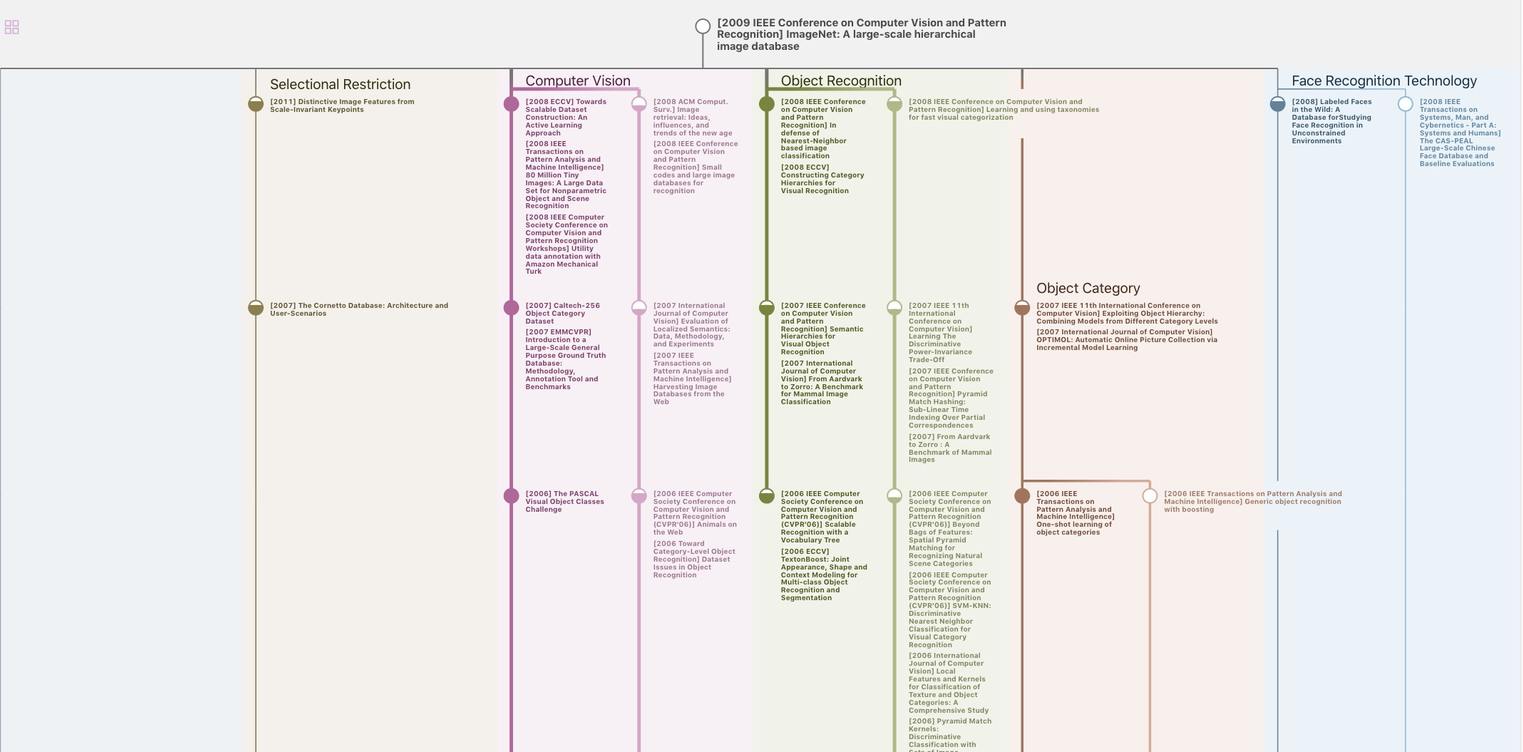
生成溯源树,研究论文发展脉络
Chat Paper
正在生成论文摘要