Measuring dynamic inefficiency through machine learning techniques.
Expert Syst. Appl.(2023)
摘要
This paper contributes by developing new models for assessing dynamic inefficiency that incorporate machine learning techniques. In particular, the new approaches apply decision trees models for the estimation of dynamic production technologies that account for investment adjustment costs. Methodologically, the new models build on the recently developed techniques of Efficiency Analysis Trees (EAT) and Convexified Efficiency Analysis Trees (CEAT) and extend them even further to a dynamic framework comprising dynamic EAT and CEAT models. The study compares dynamic inefficiency scores estimated assuming the new models against the traditional dynamic free disposal hull (FDH) and dynamic data envelopment analysis (DEA). Our empirical application focuses on dairy manufacturing firms in the main dairy processing countries in the European Union for the years 2014 and 2018. The results show that inefficiency related to the dynamic CEAT or EAT is higher than their corresponding values calculated through the dynamic DEA or FDH. The discriminating power of dynamic DEA (dynamic FDH) drastically improves when switching to dynamic CEAT (dynamic EAT). Finally, the differences between countries are observed regarding the development of dynamic inefficiency in the period associated with milk quota abolition.
更多查看译文
关键词
dynamic inefficiency,machine learning,machine learning techniques
AI 理解论文
溯源树
样例
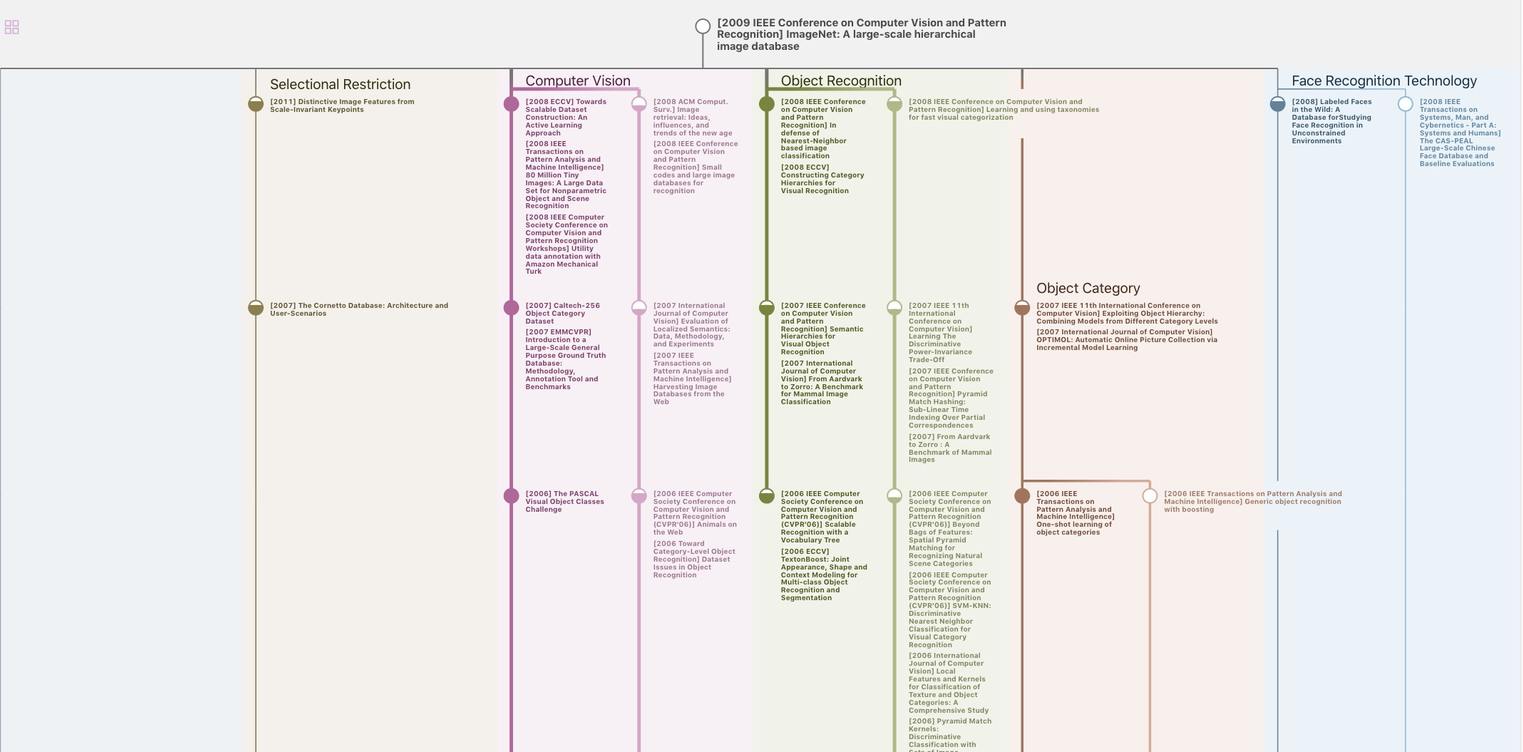
生成溯源树,研究论文发展脉络
Chat Paper
正在生成论文摘要