Traffic sign recognition using proposed lightweight twig-net with linear discriminant classifier for biometric application.
Image Vis. Comput.(2023)
摘要
The development and rise in Artificial Intelligence (AI) applications including Intelligent Transport System (ITS) has significantly reshaped and transformed. A key research area that is, an intelligent visual aid for Traffic Sign Recognition (TSR) has been certainly persuaded and transformed to control automobiles and ensure safe driving. Similarly, TSR is an important feature of Advanced Driver Assistance Systems (ADAS) that contributes to the safety of drivers, pedestrians, and automobiles. This work is aimed to recognize traffic signs robustly and efficiently in challenging environmental conditions. Hence, keeping the diversity of the problem in mind, a novel 30-layered deep Convolutional Neural Network (CNN) model is proposed. In the proposed model each convolutional layer produced around a minimum number of salient and highly discriminative features incorporating different datasets for classification purposes. This novel approach used the extracted features to train the model without the assistance of a Graphics Processing Unit (GPU). Once the model becomes trained, different competitive classifiers including Tree, SVM, KNN, and discriminant analysis are used to classify the data. The result reflects that Linear Discriminant Analysis (LDA) achieves comparable results among all other competitive classifiers with 97.4% accuracy with a minimum training time of 5.12 s on the CURE-TSR dataset. To evaluate and monitor the efficacy of the novel approach, it is further tested on two more datasets including GTSRB and BTSRB. The proposed approach acquired 99.12% and 98.16% accuracy on both datasets respectively. Finally, the comparable results on all the benchmark datasets reflect the proposed approach's applicability and advantage.
更多查看译文
关键词
Traffic sign,Classification,Machine learning,Autonomous vehicles,Computer vision
AI 理解论文
溯源树
样例
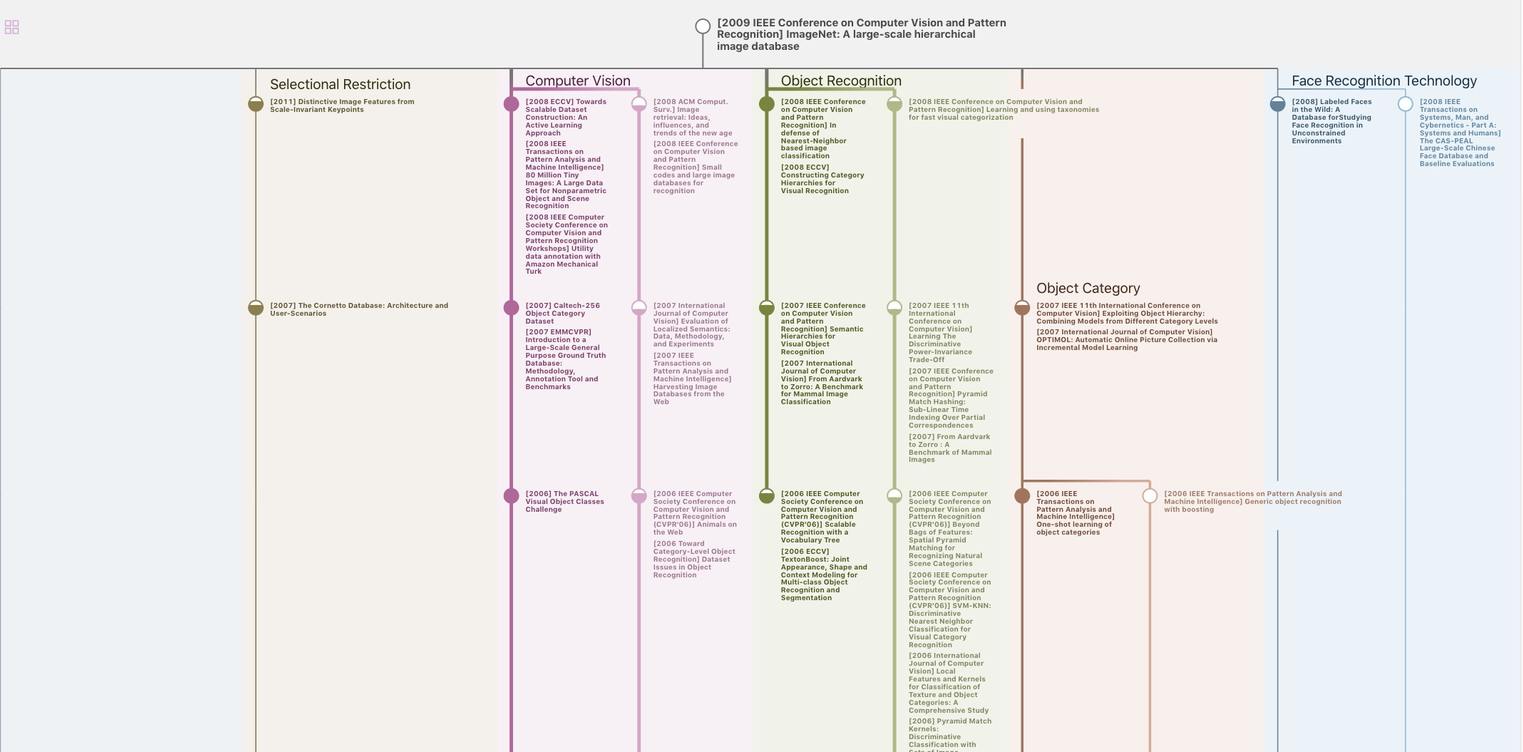
生成溯源树,研究论文发展脉络
Chat Paper
正在生成论文摘要