Deep expectation-maximization network for unsupervised image segmentation and clustering
Image Vis. Comput.(2023)
摘要
Unsupervised learning, such as unsupervised image segmentation and clustering, are fundamental tasks in image representation learning. In this paper, we design a deep expectation-maximization (DEM) network for unsuper-vised image segmentation and clustering. It is based on the statistical modeling of image in its latent feature space by Gaussian mixture model (GMM), implemented in a novel deep learning framework. Specifically, in the unsu-pervised setting, we design an auto-encoder network and an EM module over the image latent features, for jointly learning the image latent features and GMM model of the latent features in a single framework. To con-struct the EM-module, we unfold the iterative operations of EM algorithm and the online EM algorithm in fixed steps to be differentiable network blocks, plugged into the network to estimate the GMM parameters of the image latent features. The proposed network parameters can be end-to-end optimized using losses based on log-likelihood of GMM, entropy of Gaussian component assignment probabilities and image reconstruction error. Extensive experiments confirm that our proposed networks achieve favorable results compared with sev-eral state-of-the-art methods in unsupervised image segmentation and clustering.& COPY; 2023 Elsevier B.V. All rights reserved.
更多查看译文
关键词
Deep clustering,EM algorithm,Image clustering,Representation learning,Unsupervised image segmentation
AI 理解论文
溯源树
样例
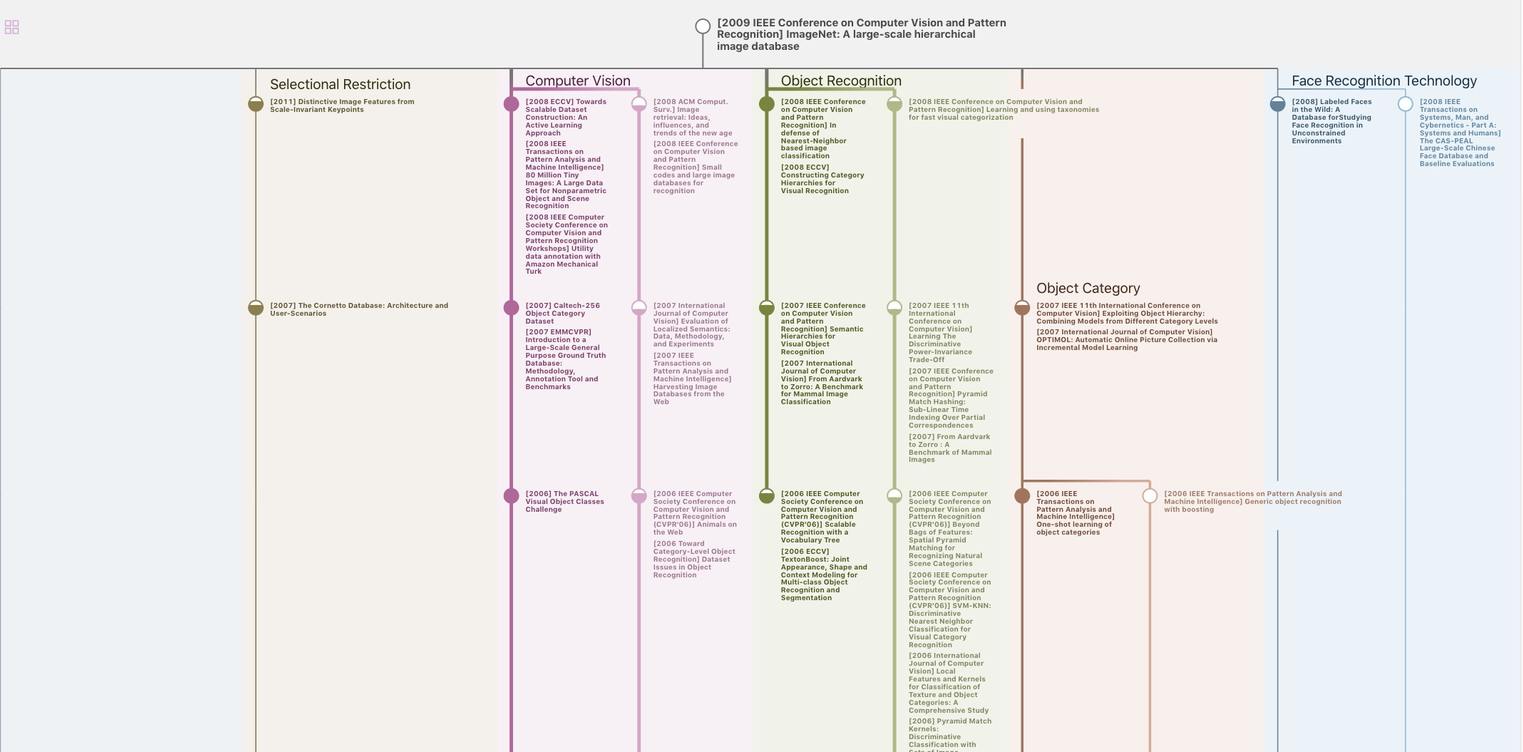
生成溯源树,研究论文发展脉络
Chat Paper
正在生成论文摘要