A new support vector machine for categorical features
Expert Syst. Appl.(2023)
摘要
Support vector machine (SVM) was originally developed to solve binary classification problems for objects with only continuous features, and it often outperforms other classifiers. However, we often encounter datasets with mixed-type features or categorical features only. This study proposes an efficient SVM for dealing with such datasets. The proposed SVM uses a subset of categorical features and it performs well in most cases, including imbalanced and/or high-dimensional categorical datasets. In particular, it is more efficient than existing SVMs in high-dimensional categorical datasets. To validate its performance, it is applied to simulated datasets and various benchmark datasets, and it is also compared to existing SVMs for categorical features.
更多查看译文
关键词
SVM,Categorical features,Mixed-type features,Variable importance
AI 理解论文
溯源树
样例
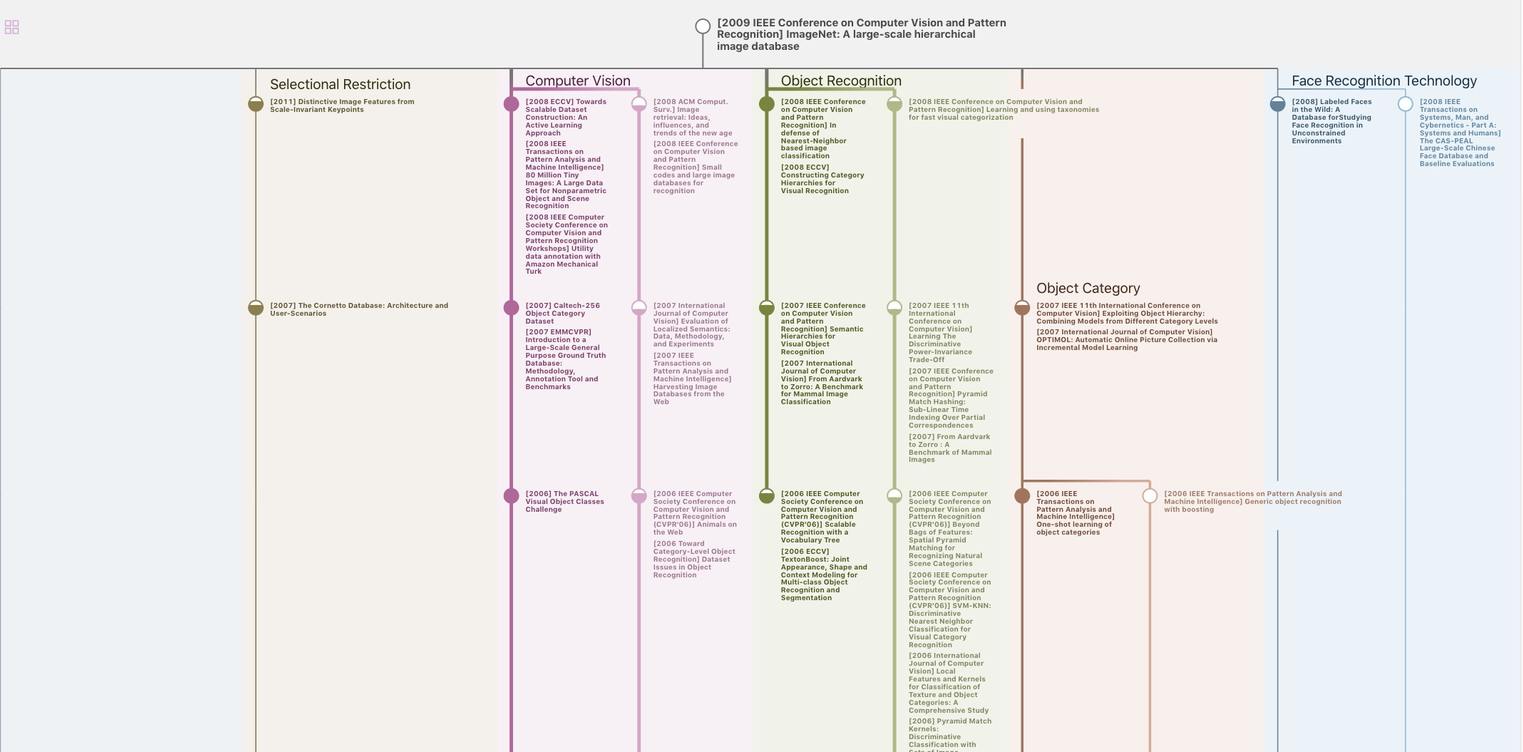
生成溯源树,研究论文发展脉络
Chat Paper
正在生成论文摘要