Imbalanced learning for wind turbine blade icing detection via spatio-temporal attention model with a self-adaptive weight loss function.
Expert Syst. Appl.(2023)
摘要
Accurate blade icing detection of wind turbines is of great significance to avoid secondary damages or accidents. Recently, data-driven methods have attracted increasing attention due to the availability of supervisory control and data acquisition (SCADA) data with their low-cost and high volume. However, SCADA data usually present complex characteristics of high dimensionality and strong spatio-temporal correlations among different sensor variables and severe class imbalance. It is challenging to extract effective features for accurate and reliable icing detection from imbalanced SCADA data. To address these challenges, we propose an imbalanced learning method for blade icing detection. First, we propose a spatio-temporal attention model to extract and assign weights to temporal–spatial features based on their contributions to blade icing detection. Then, to address the class imbalance issue, we design a self-adaptive weight (SAW) loss function to divide SCADA data into multiple batches and then classify them during model training. Unlike conventional loss functions, our SAW loss can adaptively assign weights to each data category according to their numbers in the divided batches. The effectiveness of the proposed method is verified on real SCADA datasets. The results demonstrate our designed spatio-temporal attention and SAW loss achieved better classification results and superior self-adaptability.
更多查看译文
关键词
Wind turbine, Blade icing detection, Class imbalance learning, Spatio-temporal attention, Self-adaptive weight loss function
AI 理解论文
溯源树
样例
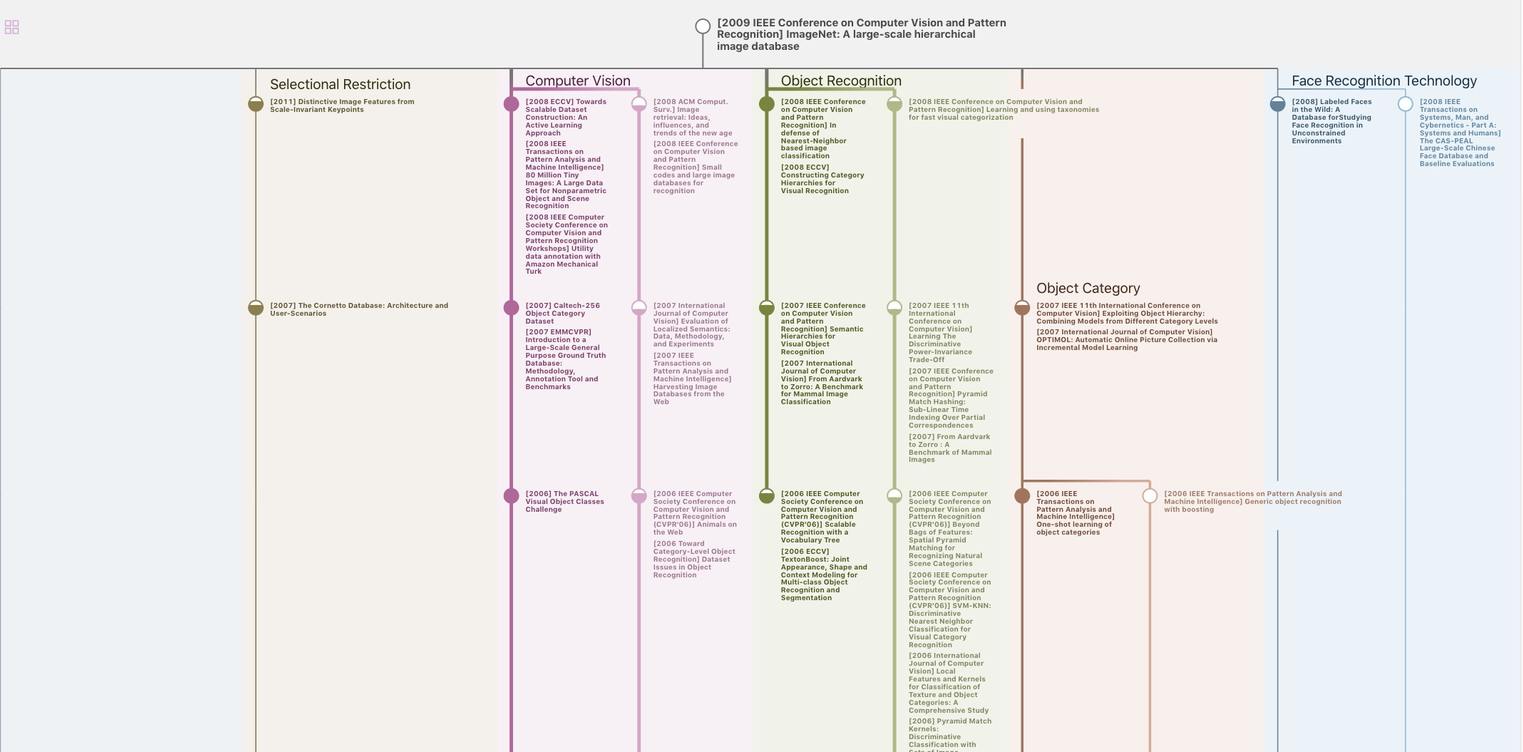
生成溯源树,研究论文发展脉络
Chat Paper
正在生成论文摘要