Application of asymmetric proximal support vector regression based on multitask learning in the stock market.
Expert Syst. Appl.(2023)
摘要
Predicting the stock price is challenging because of its volatility, high dimensions, and complex non-linearity. The multitask learning methods can capture the internal relationship among sub-tasks and obtain better prediction effect than the traditional single-task learning methods. However, most multitask learning methods ignore the inherent distribution of the original samples, which fails at achieving good generalization performance. In this paper, we first present an asymmetric squared ɛ-insensitive loss function, which can improve the generalization ability of the regressor by adjusting the asymmetric parameter. Then, an asymmetric proximal support vector regression (a-PSVR) model is proposed, which greatly improves the flexibility of proximal support vector regression (PSVR). Based on different multitask learning assumptions, two multitask learning asymmetric proximal support vector regression algorithms, i.e., MTL-a-PSVR and EMTL-a-PSVR, are advanced. Both multitask learning algorithms can obtain optimal solutions by solving quadratic programming problems. Additionally, a special case of multitask learning proximal support vector regression (MTL-PSVR) is introduced by analyzing the asymmetric squared ɛ-insensitive loss function. To illustrate the merit of the methods, the proposed models are applied to predict the trends of stock market indices in China and the U.S. and stock prices of four Chinese securities companies. The experimental results demonstrate the significant advantages of the proposed algorithms in prediction effect and generalization performance.
更多查看译文
关键词
Multitask learning, Asymmetric squared e-insensitive loss function, a-PSVR, EMTL-a-PSVR, Stock market prediction
AI 理解论文
溯源树
样例
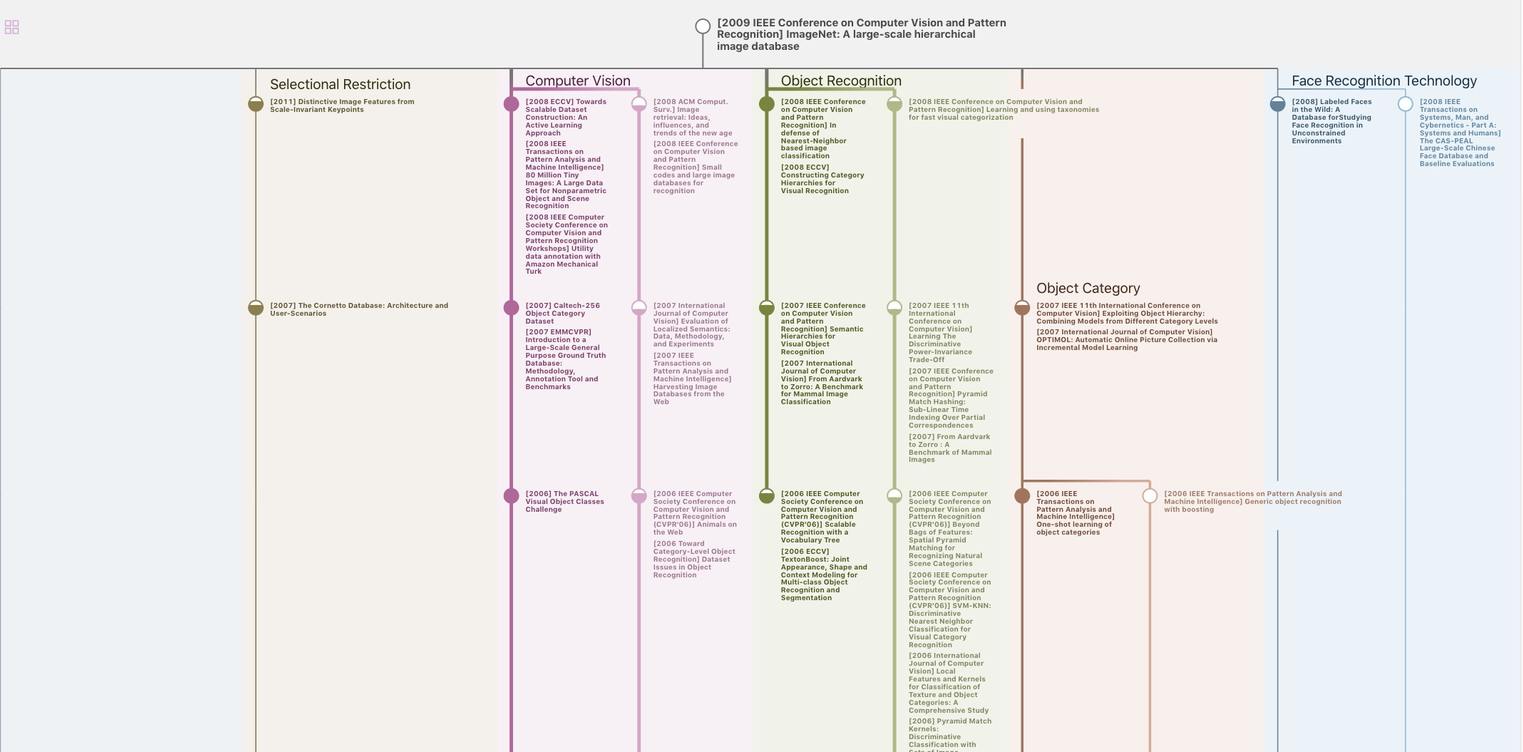
生成溯源树,研究论文发展脉络
Chat Paper
正在生成论文摘要